Fast Imitation via Behavior Foundation Models
ICLR 2024(2024)
摘要
Imitation learning (IL) aims at producing agents that can imitate any behavior given a few expert demonstrations. Yet existing approaches require many demonstrations and/or running (online or offline) reinforcement learning (RL) algorithms for each new imitation task. Here we show that recent RL foundation models based on successor measures can imitate any expert behavior almost instantly with just a few demonstrations and no need for RL or fine-tuning, while accommodating several IL principles (behavioral cloning, feature matching, reward-based, and goal-based reductions). In our experiments, imitation via RL foundation models matches, and often surpasses, the performance of SOTA offline IL algorithms, and produces imitation policies from new demonstrations within seconds instead of hours.
更多查看译文
关键词
Behavior Foundation Models,unsupervised reinforcement learning,imitation learning
AI 理解论文
溯源树
样例
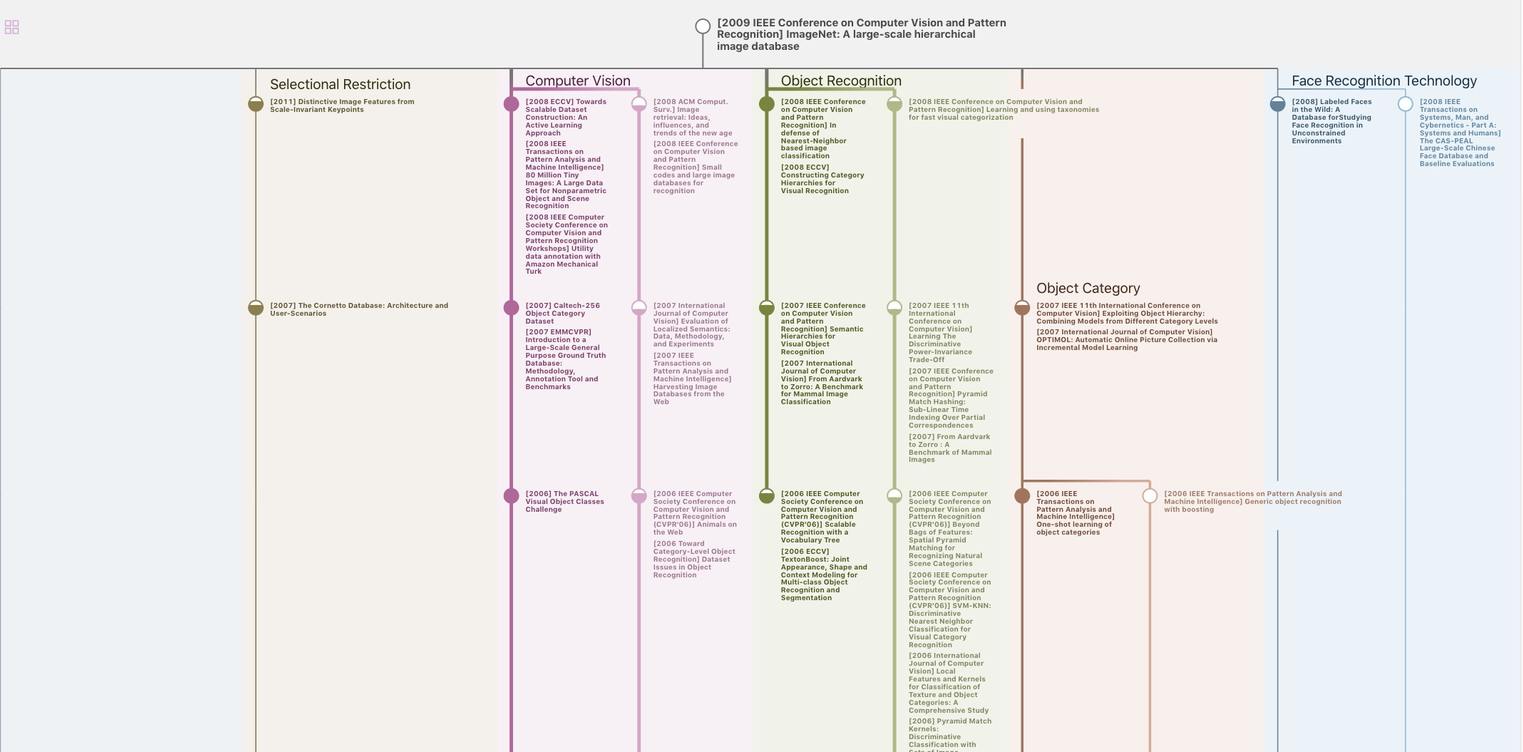
生成溯源树,研究论文发展脉络
Chat Paper
正在生成论文摘要