HeadDiff: Exploring Rotation Uncertainty With Diffusion Models for Head Pose Estimation
IEEE TRANSACTIONS ON IMAGE PROCESSING(2024)
摘要
In this paper, we propose a probabilistic regression diffusion model for head pose estimation, dubbed HeadDiff, which typically addresses the rotation uncertainty, especially when faces are captured in wild conditions. Unlike conventional image-to-pose methods which cannot explicitly establish the rotational manifold of head poses, our HeadDiff aims to ensure the pose rotation via the diffusion process and in parallel, refine the mapping process iteratively. Specifically, we initially formulate the head pose estimation problem as a reverse diffusion process, defining a paradigm for progressive denoising on the manifold, which explores the uncertainty by decomposing the large gap into intermediate steps. Moreover, our HeadDiff is equipped with an isotropic Gaussian distribution by encoding the incoherence information in our rotation representation. Finally, we learn the facial relationship of nearest neighbors with a cycle-consistent constraint for robust pose estimation versus diverse shape variations. Experimental results on multiple datasets demonstrate that our proposed method outperforms existing state-of-the-art techniques without auxiliary data.
更多查看译文
关键词
Pose estimation,Solid modeling,Manifolds,Uncertainty,Three-dimensional displays,Faces,Rotation measurement,Head pose estimation,diffusion model,structural learning,biometrics
AI 理解论文
溯源树
样例
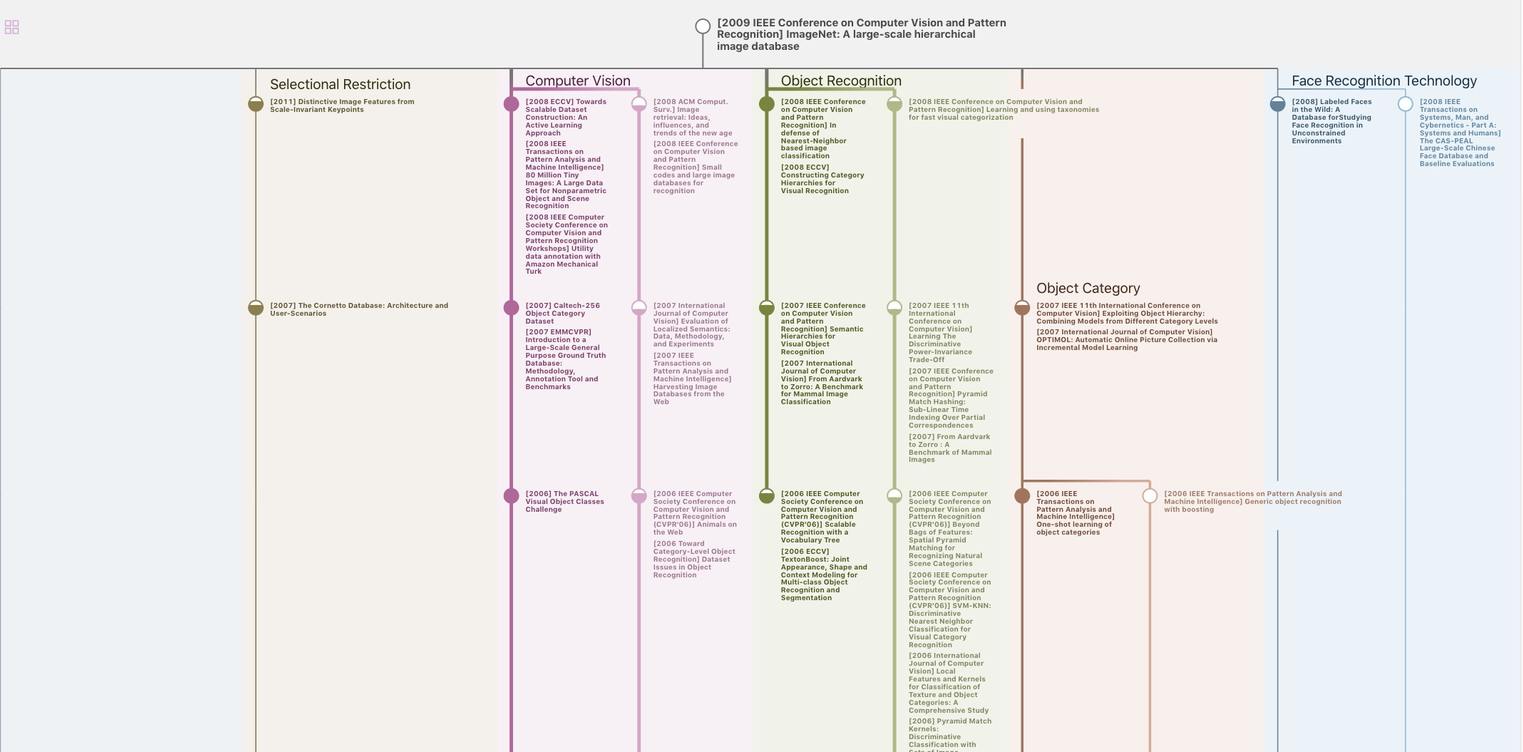
生成溯源树,研究论文发展脉络
Chat Paper
正在生成论文摘要