Global Optimality for Non-linear Constrained Restoration Problems via Invexity
ICLR 2024(2024)
摘要
Signal restoration is an important constrained optimization problem with significant applications in various domains. Although non-convex constrained optimization problems have been shown to perform better than convex counterparts in terms of reconstruction quality, convex constrained optimization problems have been preferably for its global optima guarantees. Despite the success of non-convex methods in a large number of applications, it is not an overstatement to say that there is little or no hope for non-convex problems to ensure global optima. In this paper, for the first time, we develop invex constrained optimization theory to mitigate the loss of guarantees for global optima in non-convex constrained inverse problems, where the invex function is a mapping where any critical point is a global minimizer. We also develop relevant theories to extend the global optima guarantee to a set of quasi-invex functions - the largest optimizable mappings. More specifically, we propose a family of invex/quasi-invex of functions for handling constrained inverse problems using the non-convex setting along with guarantees for their global optima. Our experimental evaluation shows that the proposed approach is very promising and can aid in extending existing convex optimization algorithms, such as the alternating direction method of multipliers, and accelerated proximal gradient methods.
更多查看译文
关键词
Constrained optimization,Invexity,Quasi-invexity,Global optima
AI 理解论文
溯源树
样例
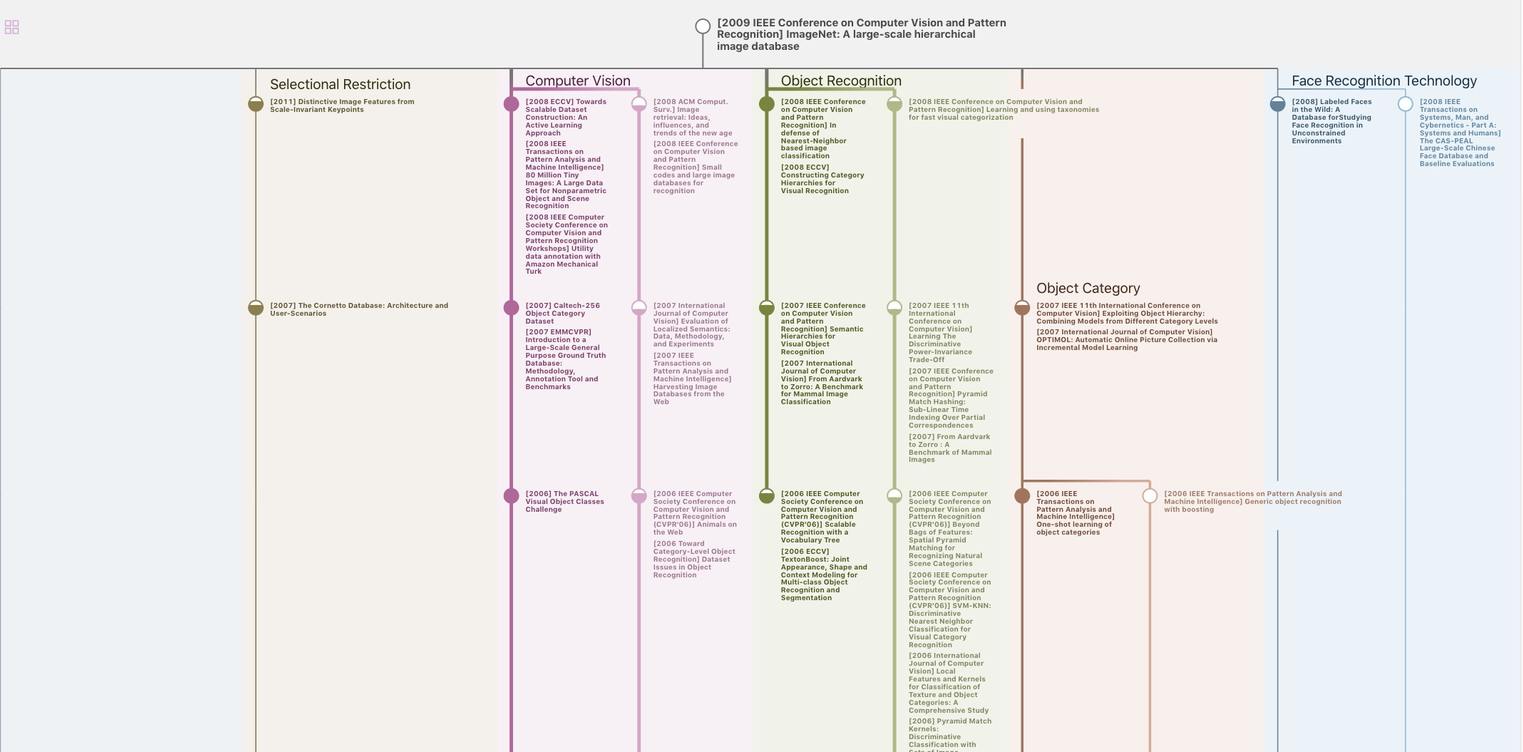
生成溯源树,研究论文发展脉络
Chat Paper
正在生成论文摘要