FedDA: Faster Adaptive Gradient Methods for Federated Constrained Optimization
ICLR 2024(2024)
摘要
Federated learning (FL) is an emerging learning paradigm in which a set of distributed clients learns a task under the coordination of a central server. The FedAvg algorithm is one of the most widely used methods to solve FL problems. In FedAvg, the learning rate is a constant rather than changing adaptively. Adaptive gradient methods have demonstrated superior performance over the constant learning rate schedules in non-distributed settings, and they have recently been adapted to FL. However, the majority of these methods are designed for unconstrained settings. Meanwhile, many crucial FL applications, like disease diagnosis and biomarker identification, often rely on constrained formulations such as Lasso and group Lasso. It remains an open question as to whether adaptive gradient methods can be effectively applied to FL problems with constrains. In this work, we introduce \textbf{FedDA}, a novel adaptive gradient framework for FL. This framework utilizes a restarted dual averaging technique and is compatible with a range of gradient estimation methods and adaptive learning rate schedules. Specifically, an instantiation of our framework \textbf{FedDA-MVR} achieves gradient complexity $\tilde{O}(K^{-1}\epsilon^{-1.5})$ and communication complexity $\tilde{O}(K^{-0.25}\epsilon^{-1.25})$ for finding a stationary point $\epsilon$ in the constrained setting. We conduct experiments over both constrained and unconstrained tasks to confirm the effectiveness of our approach.
更多查看译文
关键词
Federated Learning,Adaptive Gradient Methods
AI 理解论文
溯源树
样例
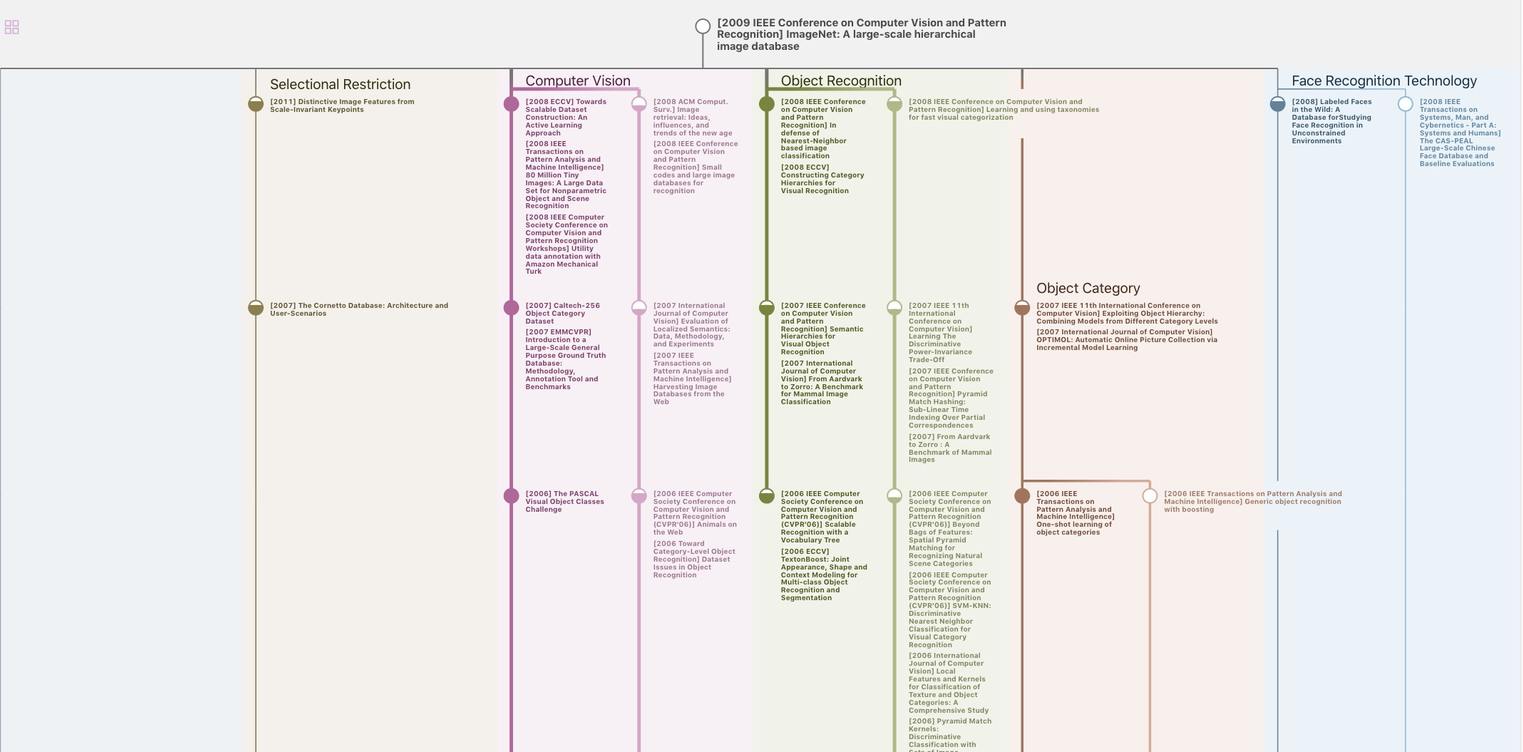
生成溯源树,研究论文发展脉络
Chat Paper
正在生成论文摘要