Bi-SGTAR: A Simple Yet Efficient Model for CircRNA-Disease Association Prediction Based on Known Association Pair Only
KNOWLEDGE-BASED SYSTEMS(2024)
摘要
Identifying circRNA (circular RNA) associated with diseases holds promise as diagnostic and prognostic biomarkers, offering potential avenues for novel therapeutics. Several computational methods have been designed to predict circRNA-disease associations. Unfortunately, current computational models face issues stemming from the integration of data from multiple sources, leading to blind spots in data combination and increased model complexity. Thus, this article introduces a novel method named Bi-SGTAR (Bi-view Sparse Gating and True Association Regression). Notably, Bi-SGTAR demonstrates comparable performance to existing multi-source information fusion methods while utilizing only known circRNA-disease association pairs. In contrast to previous methods, the model divides the adjacency matrix into two views and employs an encoder with sparse gating to assess the reliability of all associations. Additionally, a supervised reconstructor is employed to define the true association probability, quantifying the truthfulness of all associations. The Encoding-Reconstruction-Regression (ERR) framework adeptly merges both reliable and truthful associations from both views. The experimental results unequivocally show that Bi-SGTAR surpasses state-of-the-art models across seven circRNA-disease datasets, one lncRNA-disease dataset, and one microbe-drug dataset, with fewer data needed.
更多查看译文
关键词
Circular RNAs,circRNA-disease association,Biological network,Machine learning,Sparse gating
AI 理解论文
溯源树
样例
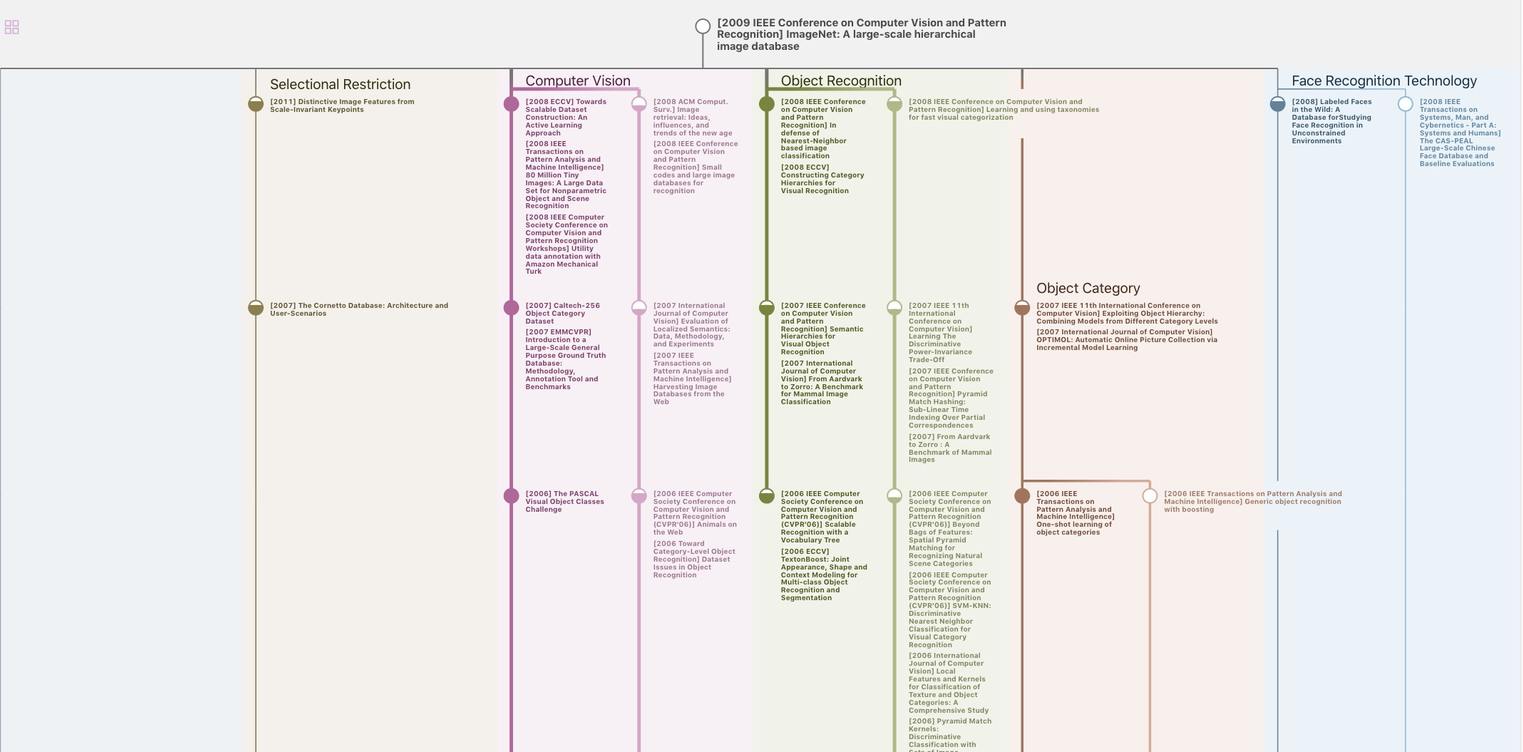
生成溯源树,研究论文发展脉络
Chat Paper
正在生成论文摘要