MixSATGen: Learning Graph Mixing for SAT Instance Generation
ICLR 2024(2024)
摘要
The Boolean satisfiability problem (SAT) stands as a canonical NP-complete task. In particular, the scarcity of real-world SAT instances and their usefulness for tuning SAT solvers underscore the necessity for effective and efficient ways of hard instance generation, whereas existing methods either struggle to maintain plausible hardness or suffer from limited applicability. Different from the typical construction-based methods, this paper introduces an adaptive and efficient graph interpolation approach that in place modifies the raw structure of graph-represented SAT instance by replacing it with a counterpart from another instance. Specifically, it involves a two-stage matching and mixing pipeline. The matching aims to find a correspondence map of literal nodes from two instance graphs via learned features from a matching network; while the mixing stage involves iteratively exchanging clause pairs with the highest correspondence scores until a specified replacement ratio is achieved. We further show that under our matching-mixing framework, moderate randomness can avoid hardness degradation of instances by introducing Gumbel noise. Experimental results show the superiority of our method with both resemblance in structure and hardness, and general applicability.
更多查看译文
关键词
Combinatorial Optimization,Boolean Satisfiability Problem,Graph Generation,Graph Matching
AI 理解论文
溯源树
样例
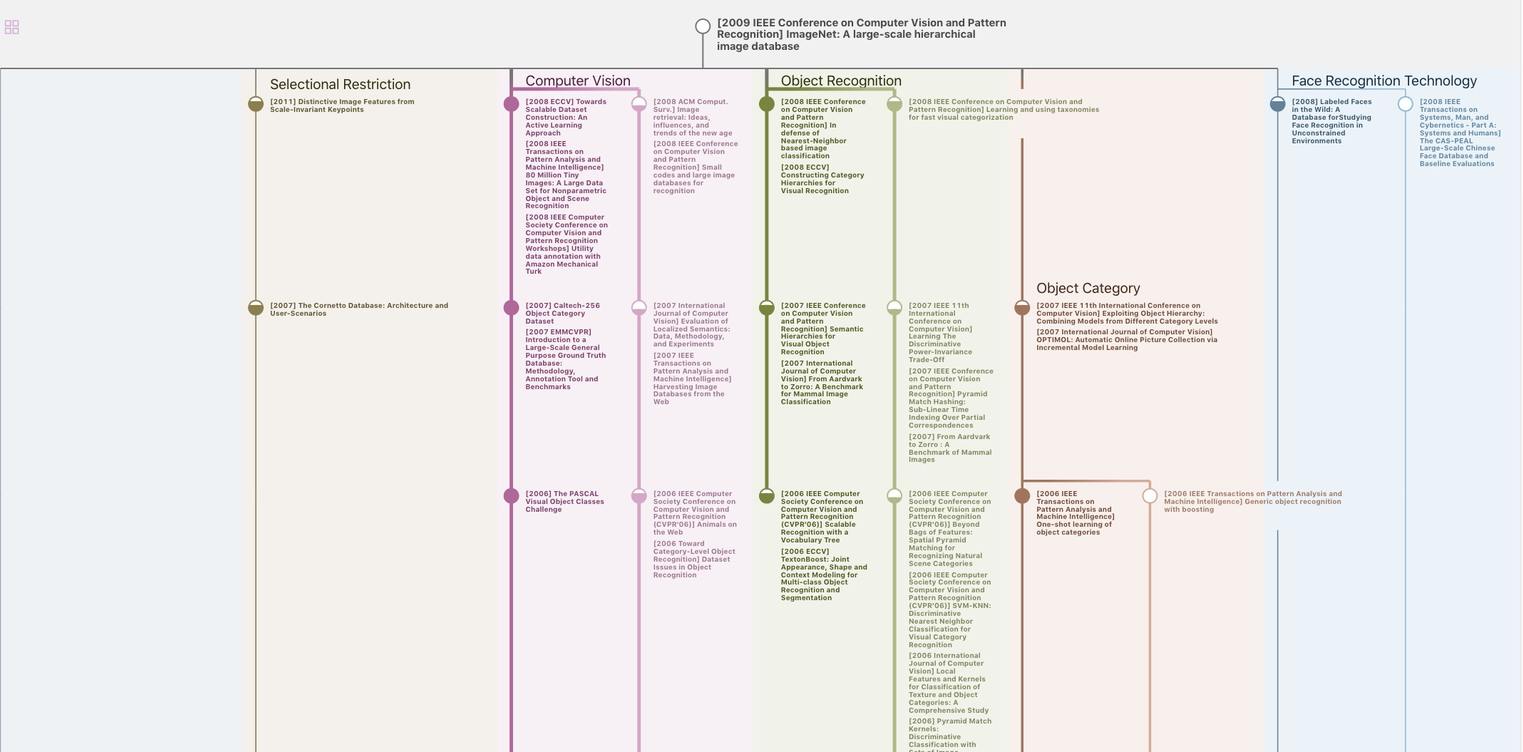
生成溯源树,研究论文发展脉络
Chat Paper
正在生成论文摘要