Understanding Expressivity of Neural KG Reasoning from Rule Structure Learning
ICLR 2024(2024)
摘要
Knowledge graph (KG) reasoning refers to the task of deducing new facts from the existing facts in KG, which has been applied in many fields. Recently, Graph Neural Networks (GNNs) with tail entity scoring achieve the state-of-the-art performance on KG reasoning. However, the theoretical understandings for these GNNs are either lacking or focusing on single-relational graphs, leaving what the kind of rule structures these GNNs can learn an open problem. We propose to fill the above gap in this paper. Specifically, GNNs with tail entity scoring are unified into a common framework. Then, we analyze their expressivity by formally describing the rule structures they can learn and theoretically demonstrating their superiority. These results further inspire us to propose a novel labeling strategy to learn more rule structures in KG reasoning. Experimental results are consistent with our theoretical findings and verify the effectiveness of our proposed method.
更多查看译文
关键词
Graph Neural Networks,KG reasoning,Link prediction,Rule structure learning,Expressivity
AI 理解论文
溯源树
样例
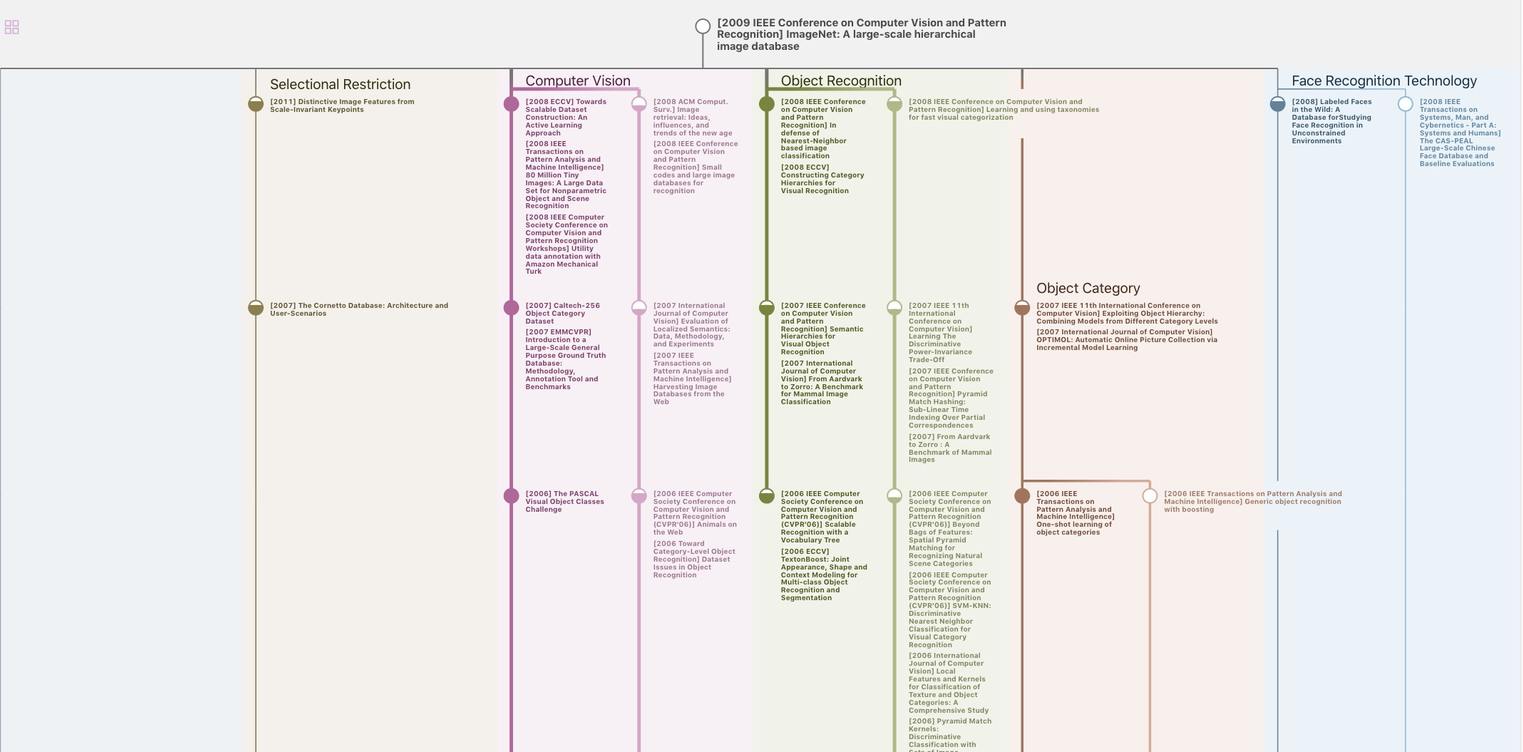
生成溯源树,研究论文发展脉络
Chat Paper
正在生成论文摘要