Incentive-Aware Federated Learning with Training-Time Model Rewards
ICLR 2024(2024)
摘要
In federated learning (FL), incentivizing contributions of training resources (e.g., data, compute) from potentially competitive clients is crucial. Existing incentive mechanisms often distribute post-training monetary rewards, which suffer from practical challenges of timeliness and feasibility of the rewards. Rewarding the clients after the completion of training may incentivize them to abort the collaboration, and monetizing the contribution is challenging in practice. To address these problems, we propose an incentive-aware algorithm that offers differentiated training-time model rewards for each client at each FL iteration. We theoretically prove that such a $\textit{local}$ design ensures the $\textit{global}$ objective of client incentivization. Through theoretical analyses, we further identify the issue of error propagation in model rewards and thus propose a stochastic reference-model recovery strategy to ensure theoretically that all the clients eventually obtain the optimal model in the limit. We perform extensive experiments to demonstrate the superior incentivizing performance of our method compared to existing baselines.
更多查看译文
关键词
Collaborative learning,Incentives,Global-to-local design
AI 理解论文
溯源树
样例
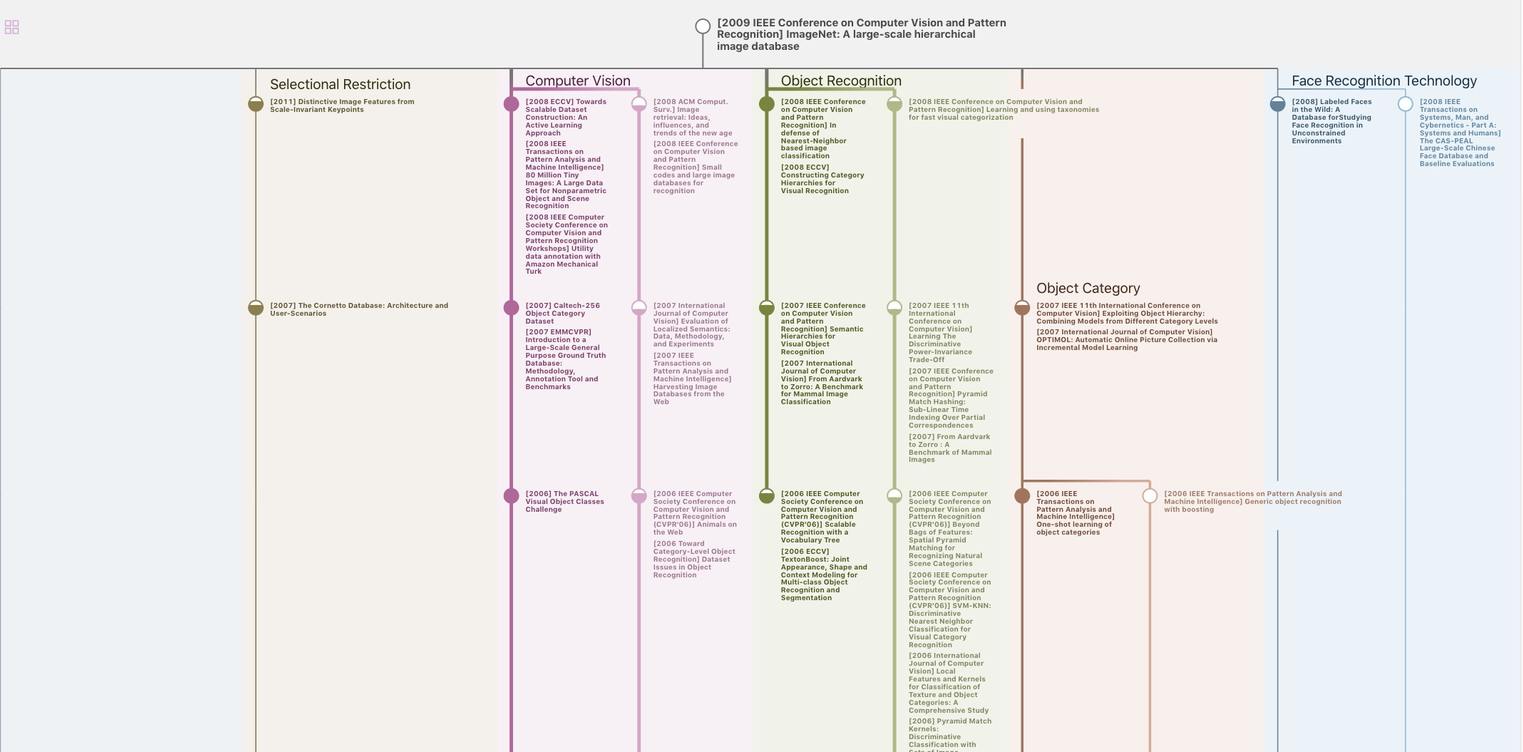
生成溯源树,研究论文发展脉络
Chat Paper
正在生成论文摘要