Language-Interfaced Tabular Oversampling via Progressive Imputation and Self-Authentication
ICLR 2024(2024)
摘要
Tabular data in the wild are frequently afflicted with class-imbalance, biasing machine learning models towards major classes. A straightforward, data-centric approach to this problem is oversampling - where synthetic minority samples are generated to balance the classes. Although tabular generative models are capable of generating synthetic samples, their integrity suffers when the number of minority samples is low. To this end, language models primed with rich prior knowledge are a fitting candidate for the task at hand. However, an oversampling strategy utilizing the extensive capabilities of such language models is yet to emerge. In this paper, we propose a novel tabular oversampling framework to channel the power of language interfaces. By leveraging its conditional sampling capabilities, we synthesize minority samples by progressively masking the important features of the majority class samples and imputing them towards the minority distribution. To reduce the inclusion of imperfectly converted samples, we utilize the power of the language model itself to self-authenticate the labels of the samples generated by itself, sifting out ill-converted samples. Extensive experiments on a variety of datasets and imbalance ratios reveal that the proposed method successfully generates reliable minority samples to boost the performance of machine learning classifiers, even under heavy imbalance ratios.
更多查看译文
关键词
Tabular data,imbalanced learning,language models
AI 理解论文
溯源树
样例
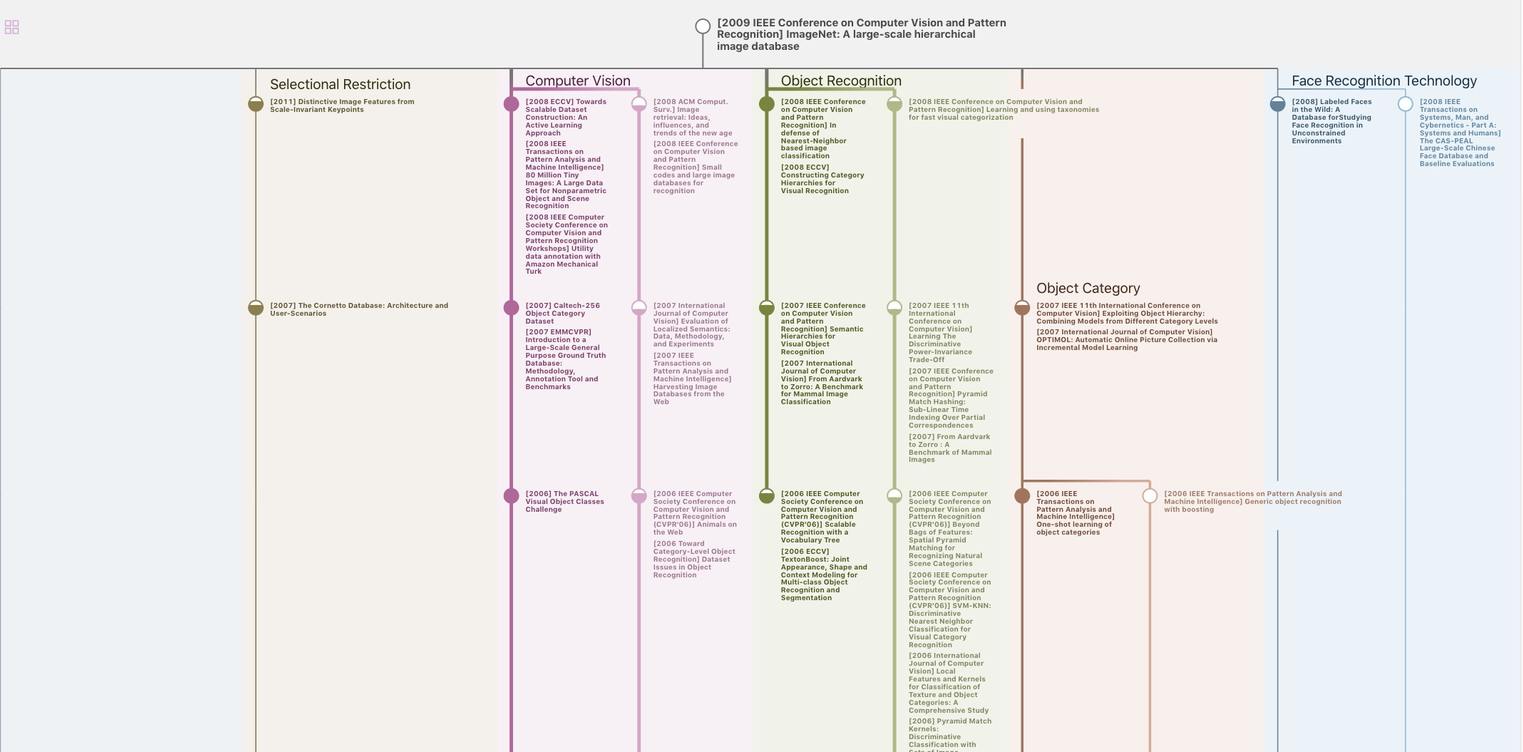
生成溯源树,研究论文发展脉络
Chat Paper
正在生成论文摘要