Confidence-aware Reward Optimization for Fine-tuning Text-to-Image Models
ICLR 2024(2024)
摘要
Fine-tuning text-to-image models using reward functions trained on human feedback data has emerged as a powerful approach for aligning model behavior with human intent. However, excessive optimization with such reward models, which are only proxy objectives, can degrade the performance of the fine-tuned models, a phenomenon commonly referred to as reward overoptimization. We introduce the Text-Image Alignment Assessment (TIA2) benchmark, a diverse collection of text prompts, images, and human annotations, for studying the issue in depth. We evaluate several state-of-the-art reward models for text-to-image generation on our benchmark and find that they are often not well-aligned with human assessment. We empirically demonstrate that overoptimization can occur when a poorly aligned reward model is used as a fine-tuning objective. To address this, we introduce a simple method, TextNorm, for inducing confidence calibration in reward models by normalizing the scores across prompts that are semantically different from the original prompt. We demonstrate that using the confidence-calibrated scores in fine-tuning effectively reduces the risk of overoptimization.
更多查看译文
关键词
text-to-image generation,overoptimization,confidence calibration
AI 理解论文
溯源树
样例
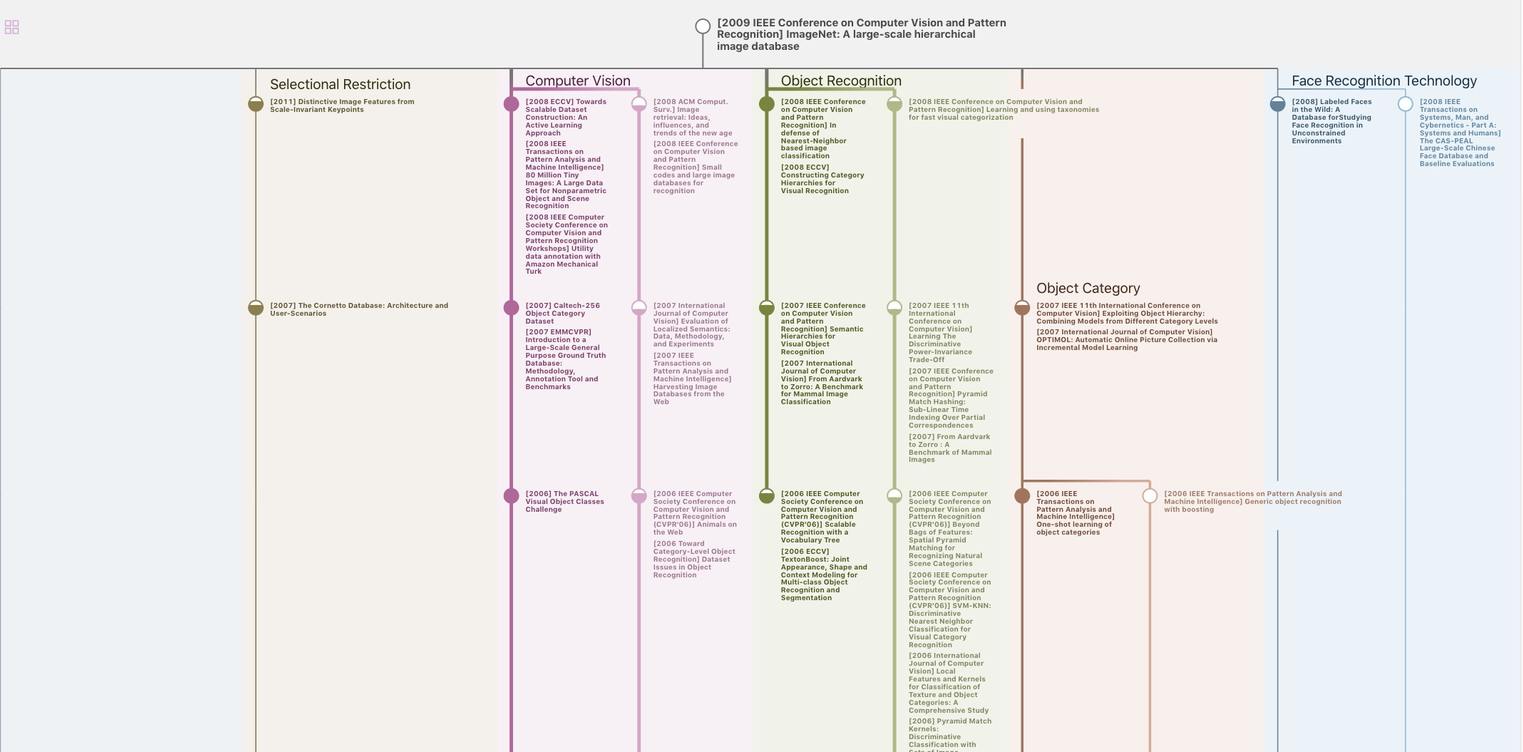
生成溯源树,研究论文发展脉络
Chat Paper
正在生成论文摘要