A Progressive Training Framework for Spiking Neural Networks with Learnable Multi-hierarchical Model
ICLR 2024(2024)
摘要
Spiking Neural Networks (SNNs) have garnered considerable attention due to their energy efficiency and unique biological characteristics. However, the widely adopted Leaky Integrate-and-Fire (LIF) model, as the mainstream neuron model in current SNN research, has been revealed to exhibit significant deficiencies in deep-layer gradient calculation and capturing global information on the time dimension. In this paper, we propose the Learnable Multi-hierarchical (LM-H) model to address these issues by dynamically regulating its membrane-related factors. We point out that the LM-H model fully encompasses the information representation range of the LIF model while offering the flexibility to adjust the extraction ratio between historical and current information. Additionally, we theoretically demonstrate the effectiveness of the LM-H model and the functionality of its internal parameters, and propose a progressive training algorithm tailored specifically for the LM-H model. Furthermore, we devise an efficient training framework for our novel advanced model, encompassing hybrid training and time-slicing online training. Through extensive experiments on various datasets, we validate the remarkable superiority of our model and training algorithm compared to previous state-of-the-art approaches. Code is available at [https://github.com/hzc1208/STBP_LMH](https://github.com/hzc1208/STBP_LMH).
更多查看译文
关键词
Spiking Neural Networks,Learnable Multi-hierarchical Model,Spatio-Temporal Back-propagation
AI 理解论文
溯源树
样例
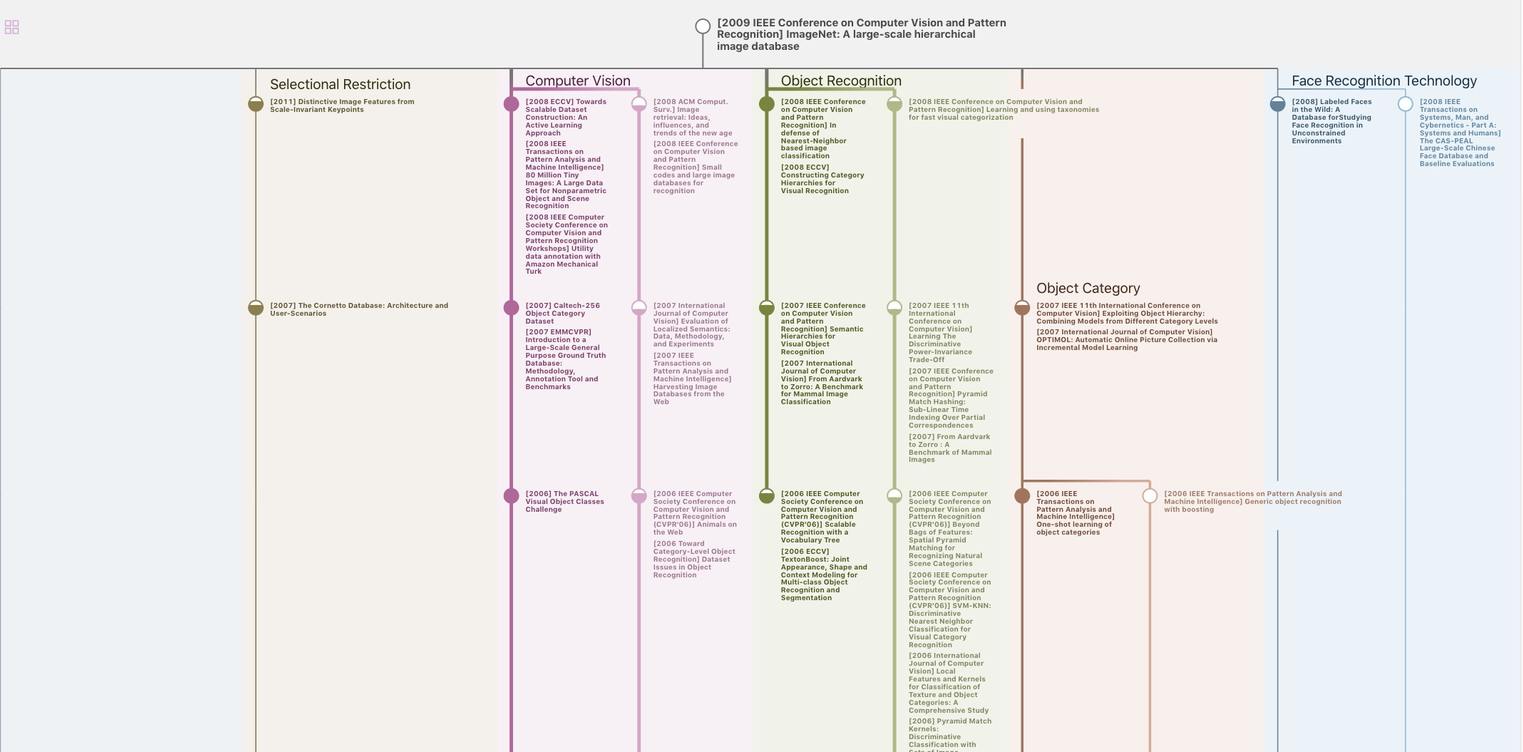
生成溯源树,研究论文发展脉络
Chat Paper
正在生成论文摘要