Learning Grounded Action Abstractions from Language
ICLR 2024(2024)
摘要
Long-horizon planning is dauntingly hard -- it requires modeling relevant aspects of the environment and searching over large, complex action spaces. \textit{Hierarchical planning} approaches make complex problems more tractable using temporal \textit{action abstractions}, decomposing hard tasks into smaller abstract subproblems that can be solved modularly. However, actually learning useful action abstractions has long posed significant challenges without human expert knowledge. Here, we introduce a system that leverages background information in language to learn a \textit{library of symbolic action abstractions and accompanying low-level policies} that can be composed to solve increasingly complex tasks. Our approach queries large language models (LLMs) as a prior for proposing useful symbolic action definitions, but integrates these proposals into a formal hierarchical planning system to ground and verify proposed actions. On two language-guided interactive planning domains (\textit{Mini Minecraft} and the \textit{ALFRED Household Tasks} benchmark), our approach far outperforms other baseline approaches that use LLMs in planning, enabling far more accurate planning and enable better generalization to more complex tasks.
更多查看译文
关键词
planning abstractions,hierarchical planning,library learning,learning from language
AI 理解论文
溯源树
样例
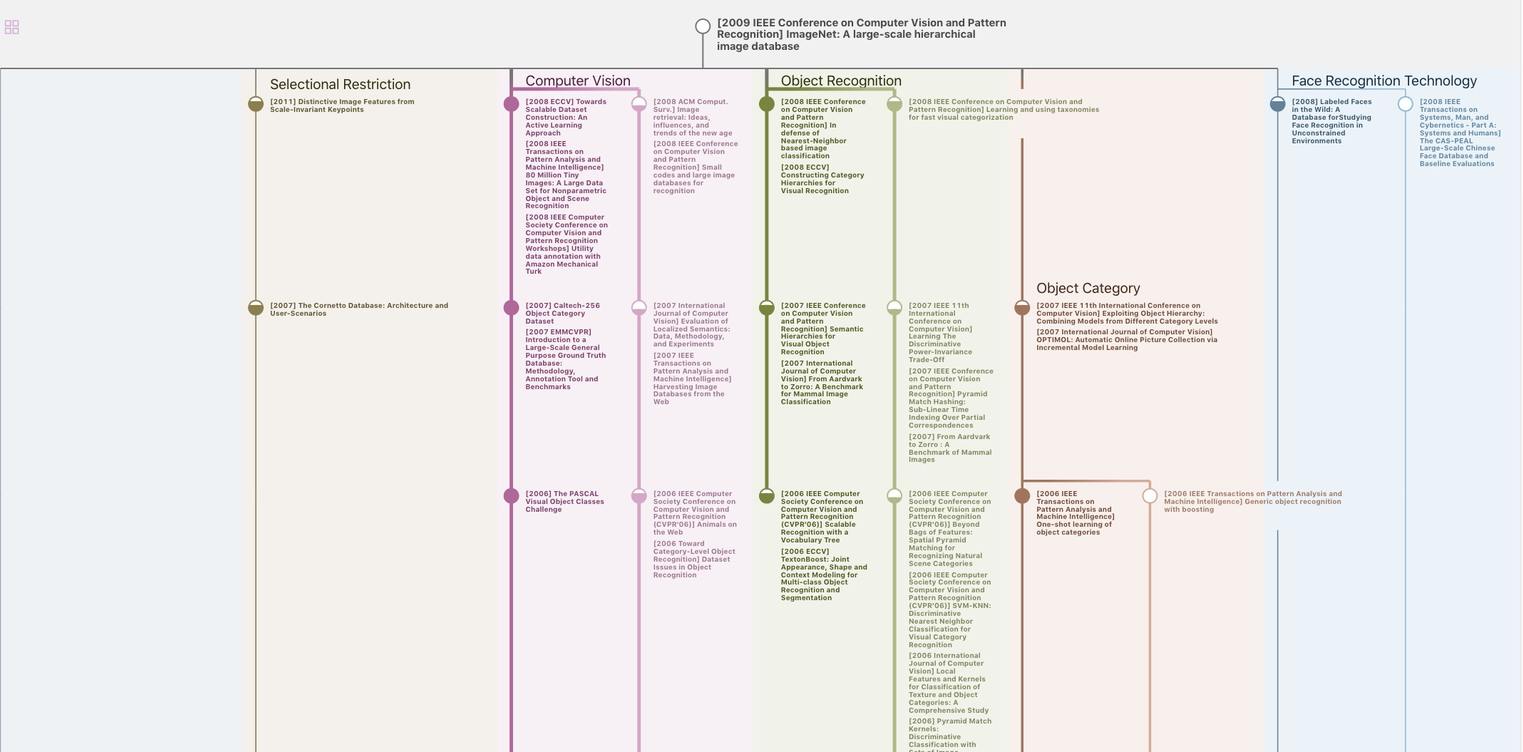
生成溯源树,研究论文发展脉络
Chat Paper
正在生成论文摘要