Spatio-Temporal Approximation: A Training-Free SNN Conversion for Transformers
ICLR 2024(2024)
摘要
Spiking neural networks (SNNs) are energy-efficient and hold great potential for large-scale inference. Since training SNNs from scratch is costly and has limited performance, converting pretrained artificial neural networks (ANNs) to SNNs is an attractive approach that retains robust performance without additional training data and resources. However, while existing conversion methods work well on convolution networks, emerging Transformer models introduce unique mechanisms like self-attention and test-time normalization, leading to non-causal non-linear interactions unachievable by current SNNs. To address this, we approximate these operations in both temporal and spatial dimensions, thereby providing the first SNN conversion pipeline for Transformers. We propose \textit{Universal Group Operators} to approximate non-linear operations spatially and a \textit{Temporal-Corrective Self-Attention Layer} that approximates spike multiplications at inference through an estimation-correction approach. Our algorithm is implemented on a pretrained ViT-B/32 from CLIP, inheriting its zero-shot classification capabilities, while improving control over conversion losses. To our knowledge, this is the first direct training-free conversion of a pretrained Transformer to a purely event-driven SNN, promising for neuromorphic hardware deployment.
更多查看译文
关键词
Spiking Neural Network,Spike Calibration,Transformer
AI 理解论文
溯源树
样例
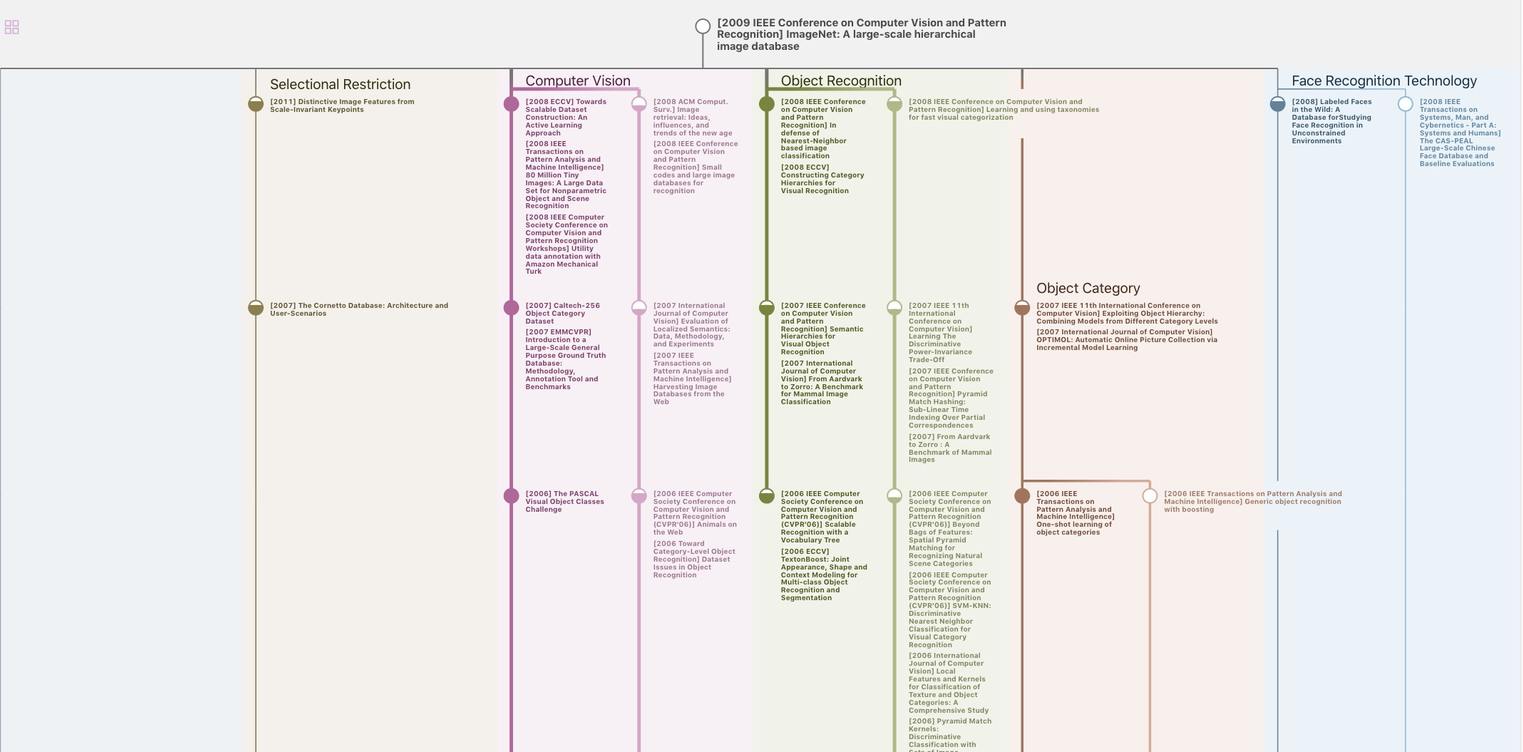
生成溯源树,研究论文发展脉络
Chat Paper
正在生成论文摘要