Breaking Physical and Linguistic Borders: Multilingual Federated Prompt Tuning for Low-Resource Languages
ICLR 2024(2024)
摘要
Pretrained large language models (LLMs) have emerged as a cornerstone in modern natural language processing, with their utility expanding to various applications and languages. However, the fine-tuning of multilingual LLMs, particularly for low-resource languages, is fraught with challenges steming from data-sharing restrictions (the physical border) and from the inherent linguistic differences (the linguistic border). These barriers hinder users of various languages, especially those in low-resource regions, from fully benefiting from the advantages of LLMs.
To overcome these challenges, we propose the Federated Prompt Tuning Paradigm for Multilingual Scenarios, which leverages parameter-efficient fine-tuning in a manner that preserves user privacy. We have designed a comprehensive set of experiments and introduced the concept of "language distance" to highlight the several strengths of this paradigm. Even under computational constraints, our method not only bolsters data efficiency but also facilitates mutual enhancements across languages, particularly benefiting low-resource ones. Compared to traditional local crosslingual transfer tuning methods, our approach achieves a 6.9\% higher accuracy, reduces the training parameters by over 99\%, and demonstrates stronger cross-lingual generalization. Such findings underscore the potential of our approach to promote social equality, ensure user privacy, and champion linguistic diversity.
更多查看译文
关键词
Multilingual Federated Learning,Natural Language Processing
AI 理解论文
溯源树
样例
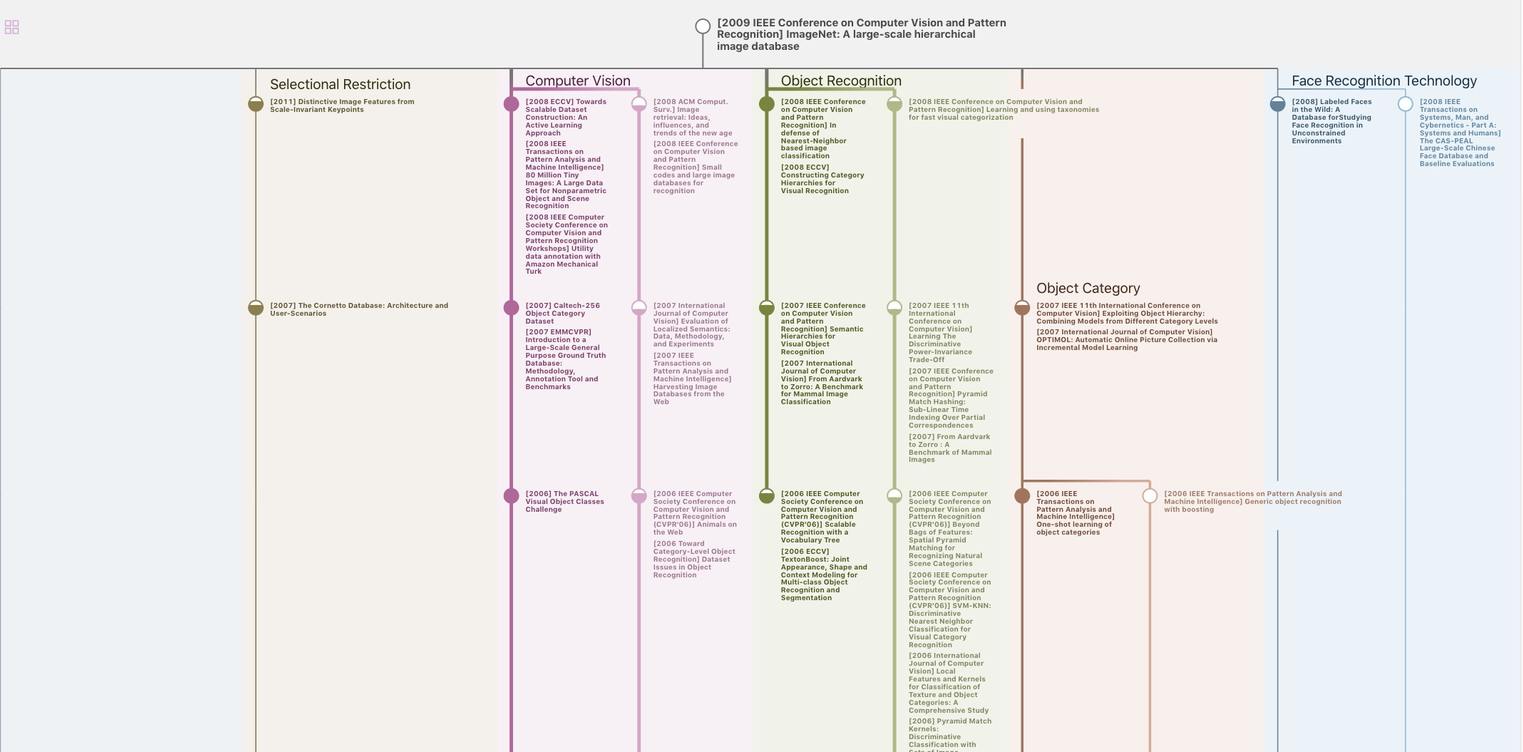
生成溯源树,研究论文发展脉络
Chat Paper
正在生成论文摘要