Dynamic normalization supervised contrastive network with multiscale compound attention mechanism for gearbox imbalanced fault diagnosis
ENGINEERING APPLICATIONS OF ARTIFICIAL INTELLIGENCE(2024)
摘要
Deep learning has gained significant success in fault diagnosis. However, the number of gearbox health samples is inevitably much larger than that of fault samples in real -world engineering, which severely limits the diagnostic performance of such methods. Thus, this paper put forward a dynamic normalization supervised contrastive network (DNSCN) with a multiscale compound attention mechanism to recognize imbalanced gearbox faults. First, a multiscale adaptive feature extractor (MAFE) possessing branch weight adjustment capability has been devised to serve as a contrastive learning backbone to effectively mine signal features. Second, a multiscale compound attention mechanism is designed to reweight the features from the MAFE, thus improving the accuracy and confidence of fault recognition. Third, a dynamic normalized supervised contrastive loss function for imbalanced scenarios is presented. It balances the contributions of minority and hard -to -classify samples in the loss function using class normalization and dynamic adjustment based on the training accuracy, respectively. DNSCN achieved accuracies of 91.58% and 90.96% on two gearbox datasets with extreme imbalance ratios, which proved the superior performance of this approach.
更多查看译文
关键词
Gearbox fault diagnosis,Data class imbalance,Multiscale adaptive feature extractor,Attention mechanism,Dynamic normalization supervised contrastive,learning,Yan et al.,2023).
AI 理解论文
溯源树
样例
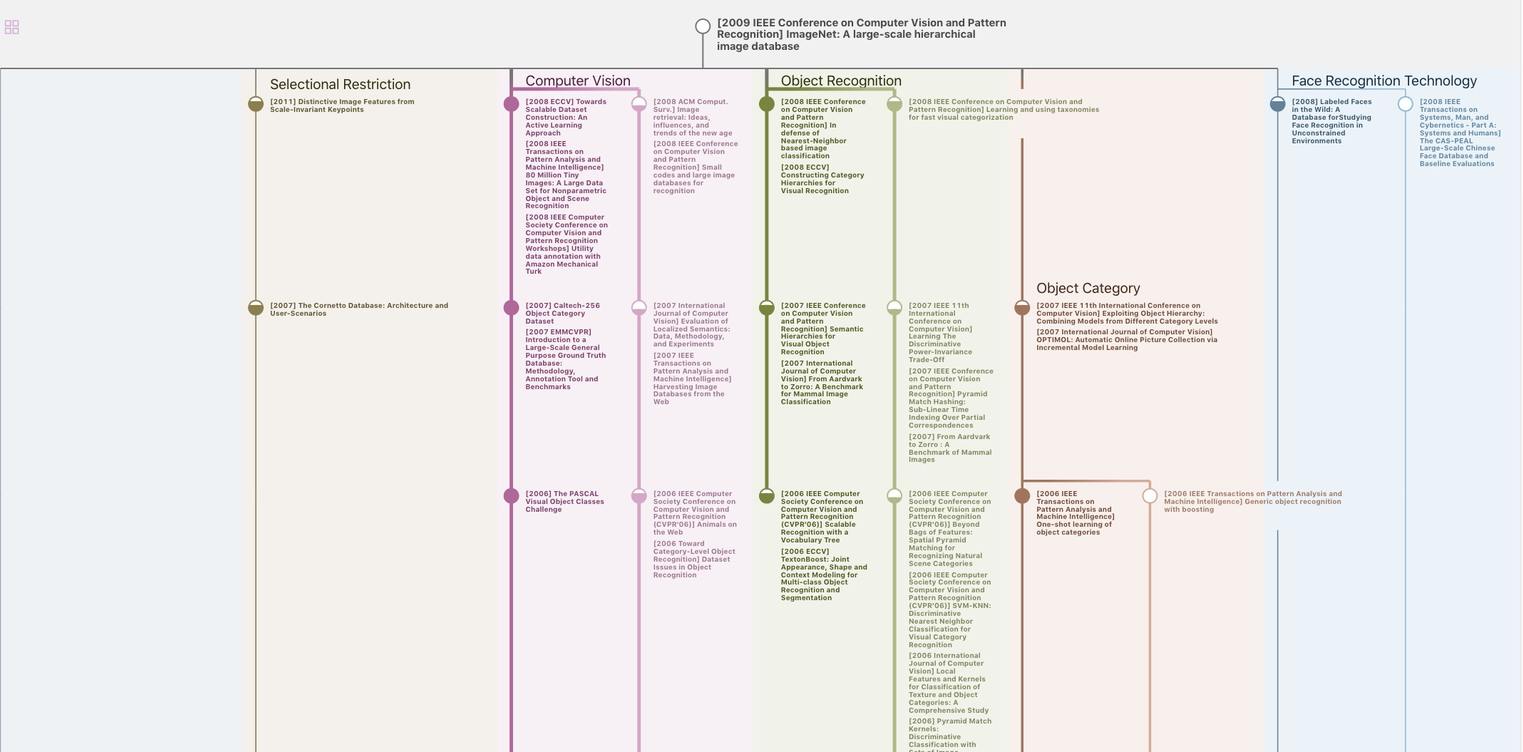
生成溯源树,研究论文发展脉络
Chat Paper
正在生成论文摘要