A comparison of machine learning methods for knowledge extraction model in A LoRa-Based waste bin monitoring system
IJAIN (International Journal of Advances in Intelligent Informatics)(2024)
摘要
Knowledge Extraction Model (KEM) is a system that extracts knowledge through an IoT-based smart waste bin emptying scheduling classification. Classification is a difficult problem and requires an efficient classification method. This research contributes in the form of the KEM system in the classification of scheduling for emptying waste bins with the best performance of the Machine Learning method. The research aims to compare the performance of Machine Learning methods in the form of Decision Tree, Naïve Bayes, K-Nearest Neighbor, Support Vector Machine, and Multi-Layer Perceptron, which will be recommended in the KEM system. Performance testing was performed on accuracy, recall, precision, F-Measure, and ROCS curves using the cross-validation method with ten observations. The experimental results show that the Decision Tree performs best for accuracy, recall, precision, and ROCS curve. In contrast, the K-NN method obtains the highest F-measure performance. KEM can be implemented to extract knowledge from data sets created in various other IoT-based systems.
更多查看译文
关键词
iot,knowledge extraction model,lora,machine learning,waste management
AI 理解论文
溯源树
样例
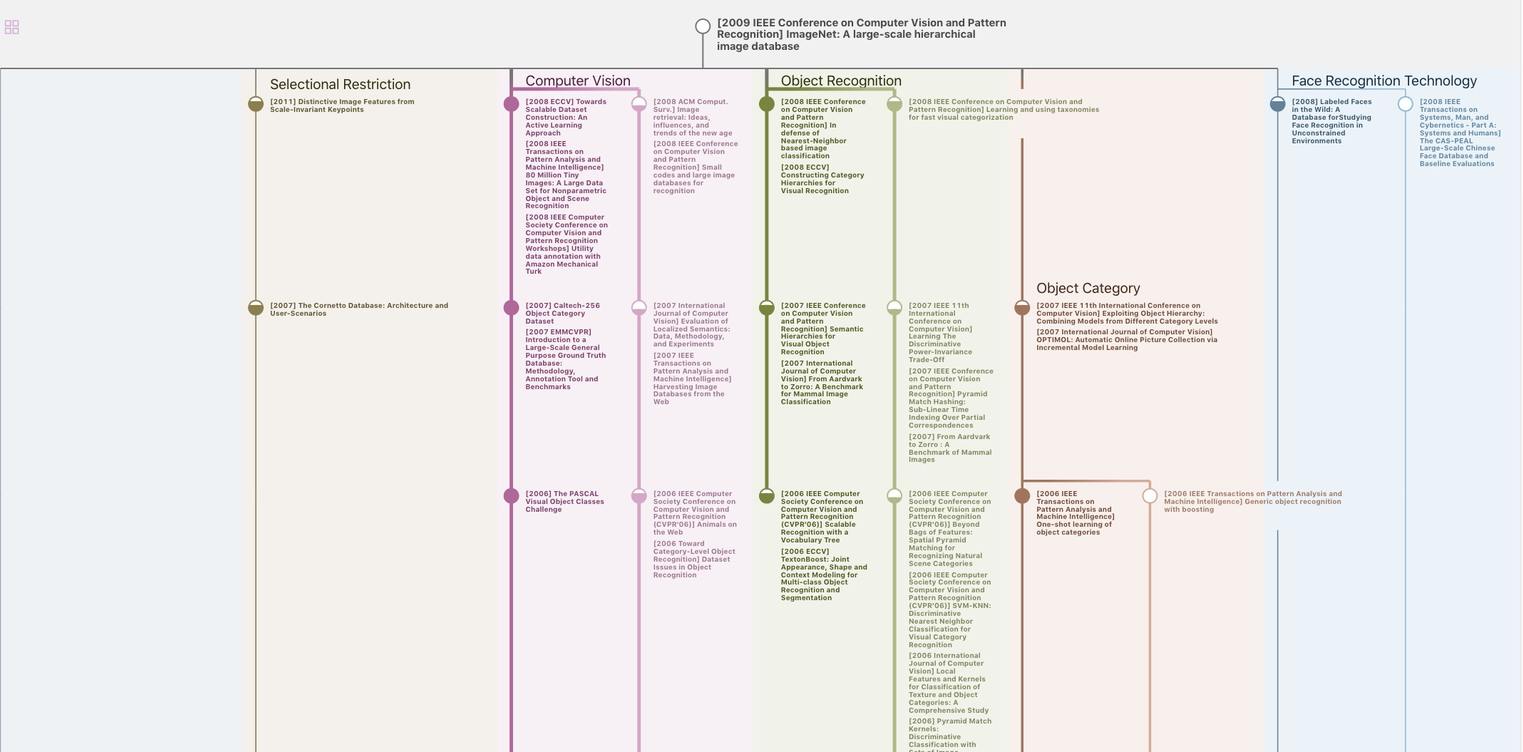
生成溯源树,研究论文发展脉络
Chat Paper
正在生成论文摘要