Improved detection of low-frequency within-host variants from deep sequencing: A case study with human papillomavirus
VIRUS EVOLUTION(2024)
摘要
High-coverage sequencing allows the study of variants occurring at low frequencies within samples, but is susceptible to false-positives caused by sequencing error. Ion Torrent has a very low single nucleotide variant (SNV) error rate and has been employed for the majority of human papillomavirus (HPV) whole genome sequences. However, benchmarking of intrahost SNVs (iSNVs) has been challenging, partly due to limitations imposed by the HPV life cycle. We address this problem by deep sequencing three replicates for each of 31 samples of HPV type 18 (HPV18). Errors, defined as iSNVs observed in only one of three replicates, are dominated by C -> T (G -> A) changes, independently of trinucleotide context. True iSNVs, defined as those observed in all three replicates, instead show a more diverse SNV type distribution, with particularly elevated C -> T rates in CCG context (CCG -> CTG; CGG -> CAG) and C -> A rates in ACG context (ACG -> AAG; CGT -> CTT). Characterization of true iSNVs allowed us to develop two methods for detecting true variants: (1) VCFgenie, a dynamic binomial filtering tool which uses each variant's allele count and coverage instead of fixed frequency cut-offs; and (2) a machine learning binary classifier which trains eXtreme Gradient Boosting models on variant features such as quality and trinucleotide context. Each approach outperforms fixed-cut-off filtering of iSNVs, and performance is enhanced when both are used together. Our results provide improved methods for identifying true iSNVs in within-host applications across sequencing platforms, specifically using HPV18 as a case study.
更多查看译文
关键词
deep sequencing,intrahost single nucleotide variant (iSNV),machine learning,sequencing error,VCFgenie
AI 理解论文
溯源树
样例
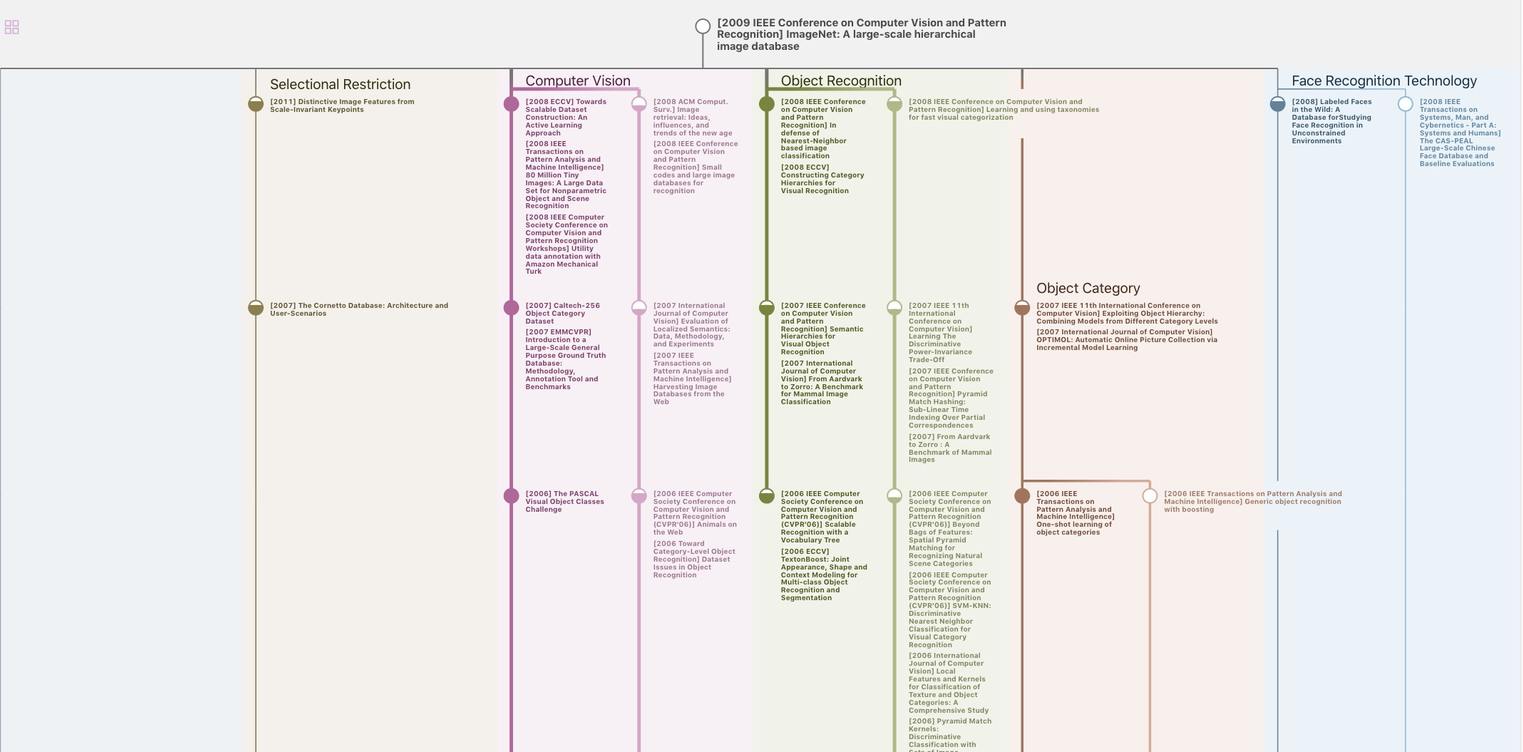
生成溯源树,研究论文发展脉络
Chat Paper
正在生成论文摘要