A Machine Learning application towards a better representation of Madrid’s urban climate
crossref(2024)
摘要
Cities are considered local “hotspots” of climate change. Urban areas concentrate a large fraction of global population, wealth, and emissions, exposing their inhabitants to climate change impacts. Therefore, the improvement of urban present climate description and future projections are paramount for designing adaptation and mitigation strategies. Global Climate Models are state-of-the-art tools for projecting future climate. However, most of the simulations have coarse resolutions and do not have physical urban parametrisations to adequately represent the physical properties and processes at the urban scale. The advantage of applying a machine learning approach – Extreme Gradient Boosting (XGBoost) – is explored for better describing Madrid’s urban climate. Namely, the ability to reproduce present and future climates: 2-m air temperature (Tmax, Tmin); surface temperature (LST); urban heat island (UHI) and surface urban heat island (SUHI) effects. XGBoost is evaluated at monthly and daily scales for local ground temperatures and, also at hourly scale, to represent the spatial structure of land surface temperature w.r.t. remote sensing data. Firstly, for present climate, XGBoost is trained with a set of ERA5 predictors (0.25º), ground stations, and Land Surface Temperature observations. Secondly, a number of sensitivity cases are performed to assess the results dependency to predictors and their resolution. Thirdly, the learned relationships between the set of predictors and predictands is applied to Earth System Global Climate Models (ESGCM) predictors, providing historical and future climate projections for the 21st century under four emission scenarios. Overall, XGBoost results reveal a good performance and significant added value against ERA5 and the ESGCM. XGBoost greatly improves the reproduction of the present climate Tmax, Tmin, LST, and more importantly, the UHI (-0.5ºC and +3ºC for Tmax and Tmin), and the SUHI (+1ºC and +2ºC for Tmax and Tmin). For future climate, XGBoost significantly corrects the ESGCM UHI misrepresentation but seems to underestimate the expected Madrid’s local warming (3.5ºC anomaly). This work was funded by the Portuguese Fundação para a Ciência e a Tecnologia (FCT) I.P./MCTES through national funds (PIDDAC) – UIDB/50019/2020-IDL. Angelina Bushenkova was supported by a grant through the project “ELABORAÇÃO DA ESTRATÉGIA REGIONAL DE ADAPTAÇÃO ÀS ALTERAÇÕES CLIMÁTICAS DO ALENTEJO – ERAACA”.Frederico Johannsen was supported by FCT with the doctoral grant UI/BD/151498/2021. Daniela C.A. Lima was supported by the Scientific Employment Stimulus 5th edition from Fundação para a Ciência e a Tecnologia (FCT, 2022.03183.CEECIND).
更多查看译文
AI 理解论文
溯源树
样例
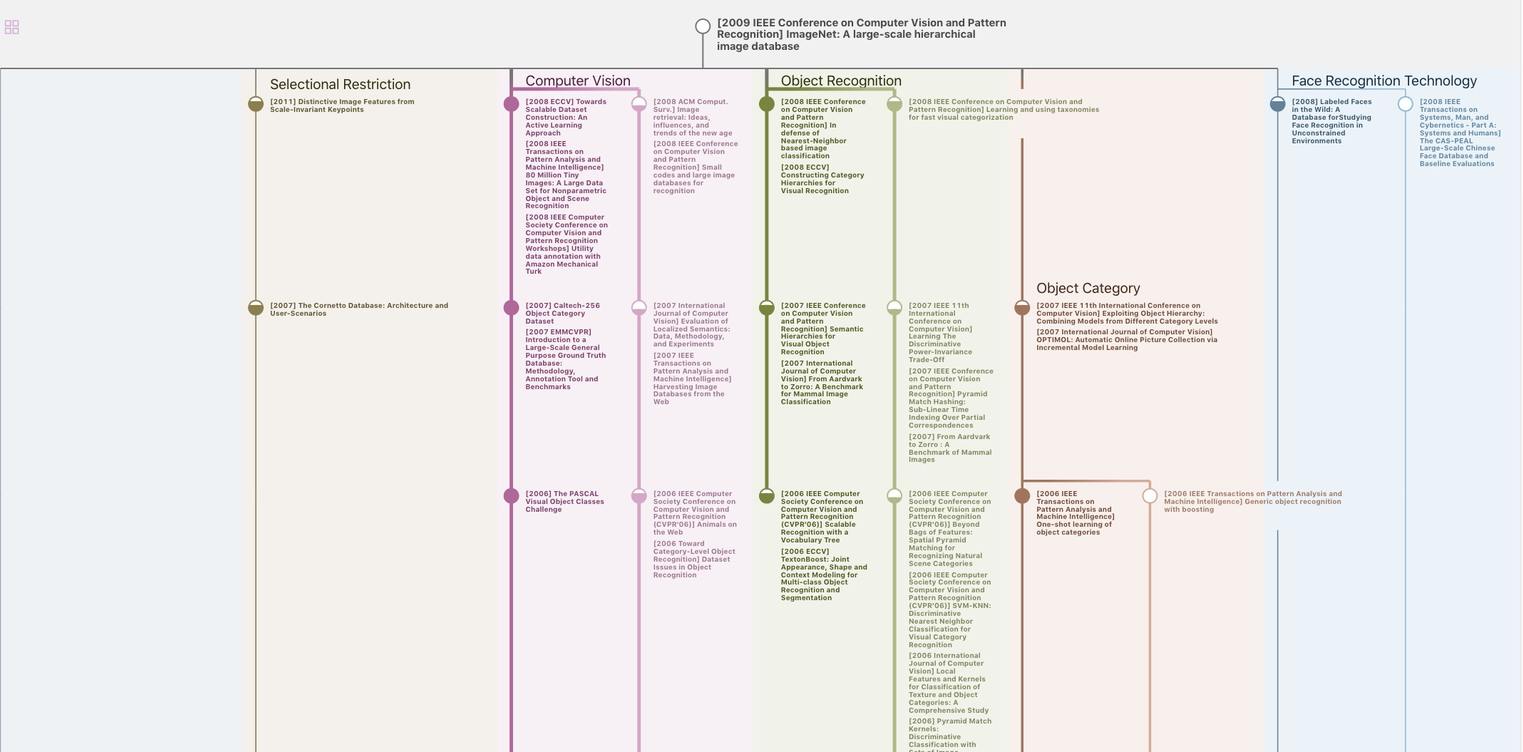
生成溯源树,研究论文发展脉络
Chat Paper
正在生成论文摘要