An Efficient Low-Perceptual Environmental Sound Classification Adversarial Method Based on GAN
Multimedia tools and applications(2024)
摘要
By incorporating additive perturbations to real samples, adversarial examples have notably exhibited the capability to deceive deep neural networks. Although the existing audio adversarial methods can successfully attack environmental sound classification (ESC) models, these generated perturbations can be easily perceived by humans. And the perturbations cannot be generated efficiently because of the large adversarial perturbation search space in audio. To address the problems, this paper proposes a Short-time Spectrum Generative Adversarial Network-based (StS-GAN) attack method to improve the performance of generated adversaries. In this method, a GAN is implemented to generate the magnitude spectrum perturbations with real signal magnitude spectra as inputs, and adversarial magnitude spectra are obtained as the superposition of the real signal magnitude spectra and the perturbations. Additionally, a short-time processing scheme is adopted to flexibly adjust the input length of the generator to balance computational complexity and attack performance. Through adversarial training, StS-GAN learns to generate adversarial examples with temporal-spectral characteristics similar to those of real signals. The learned perturbations tend to have smaller energies, making them less significant and less distinguishable by human perception. Thorough experiments show that, compared to existing adversarial attack methods, the proposed method achieves a higher Attack Success Rate (ASR) and efficiency, and the generated perturbations are less likely to be perceived by humans. The average ASR reaches 97% while maintaining a mean energy ratio of above 30 dB between the real signal and the generated perturbation, demonstrating the effectiveness of the proposed method.
更多查看译文
关键词
Environmental sound classification,Deep learning,Generative Adversarial Network,Short-time spectrum,Adversarial example
AI 理解论文
溯源树
样例
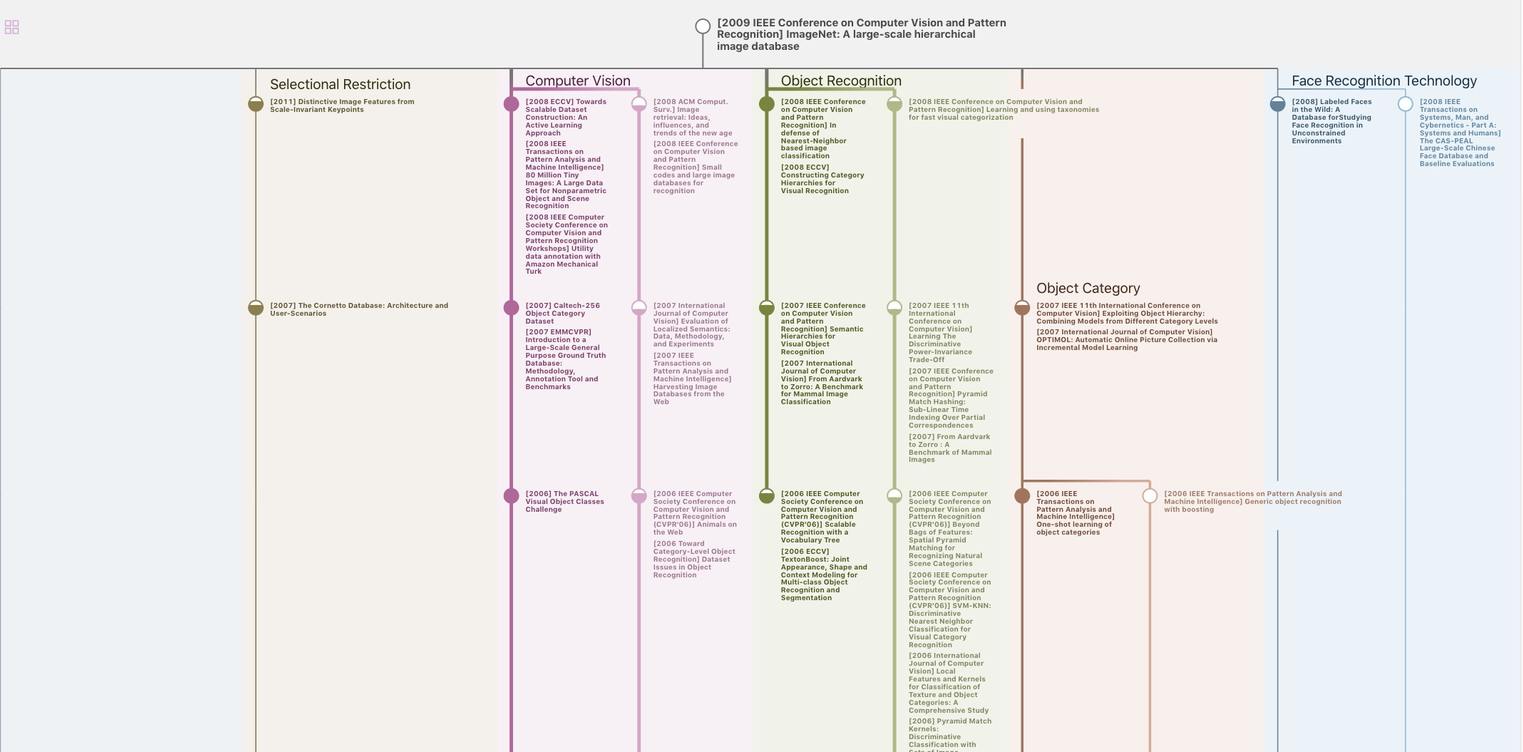
生成溯源树,研究论文发展脉络
Chat Paper
正在生成论文摘要