Towards sub-daily monitoring of polynya dynamics using deep-learning enhanced calibrated multi-mission thermal-infrared imagery
crossref(2024)
摘要
Antarctic coastal and flaw-lead polynyas are recurring areas of thin ice and open water which are generally formed by divergent ice motion during winter and are considered important hot spots for sea-ice production, deep-water formation and gas ventilation of the ocean. Due to the dependency of ocean-to-atmosphere heat flux on the ice thickness and the nature of thermodynamic ice growth by release of latent heat at the ice/ocean interface, high rates of ice production occur predominately under thin-ice/frazil-ice areas as well as in open water areas during winter time. Several Arctic and Antarctic studies use Moderate Resolution Imaging Spectroradiometer (MODIS) thermal-infrared data to monitor polynya area and associated sea-ice production in polynyas and compare well to or even outperform studies using passive-microwave satellite data in certain regions. However, especially positive temperature-anomaly features such as large warm open-water areas through sea-ice polynyas pose a problem for the MODIS cloud mask and result in frequent misclassification of these areas as cloud cover. Furthermore, the frequent presence of cloud cover in polar regions limits the use of MODIS and similar instruments for the investigation and monitoring of sea-ice polynyas compared to passive-microwave-based sensors. The very low thermal contrast between present clouds and the sea-ice surface in combination with the lack of available visible and near-infrared channels during polar nighttime results in deficiencies in the cloud masking and data gaps that degrade downstream data products. Here, a novel dataset on polynya area and sea-ice production from three thermal-infrared sensors (MODIS, SLSTR, VIIRS) is presented using a deep-learning-based approach. This approach utilizes a set of Convolutional Neural Networks that are able to reliably discriminate between clouds, sea-ice, and open-water and/or thin-ice areas in a given swath solely from thermal-infrared channels and derived additional information. Furthermore, this work aims to implement an image inpainting approach using a conditional Generative Adversarial Network (GAN) to fill cloud-induced data gaps. In its final version, this data set aims to covers the major Antarctic coastal polynyas for the last 24 years (2000–2023) for the austral winter (1 April to 30 September) period with all polynya parameters being derived from sub-daily cloud-cover corrected thin-ice thickness images and daily composites.
更多查看译文
AI 理解论文
溯源树
样例
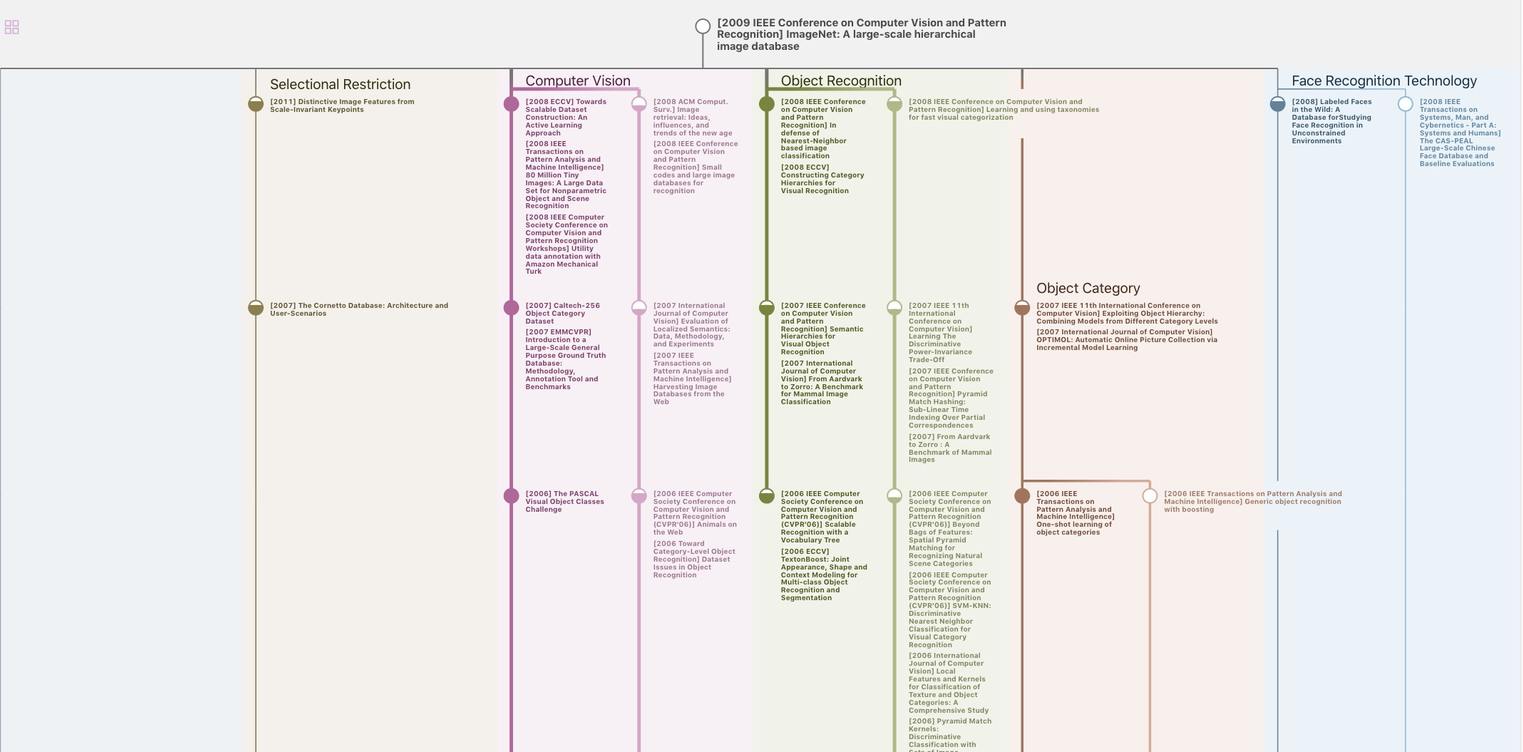
生成溯源树,研究论文发展脉络
Chat Paper
正在生成论文摘要