Soil organic carbon dynamics after land-use change – combining process-based modelling with machine learning
crossref(2024)
摘要
Land-use changes affect soil organic carbon (SOC) stocks over decades. However, IPCC default for greenhouse gas emissions reporting suggests a simple linear SOC stock change over 20 years only. Using process-based modelling approaches such as RothC to describe SOC dynamics after land-use change requires model validation. However, there are only few long-term field experiments where SOC stocks have been observed long enough to get sufficient data for such a model validation. This lack of data makes validating models for large-scale use challenging. Based on empirical data from over 3000 sites from the German Agricultural Soil Inventory we selected 204 sites with land-use change history within the last 60 years and created an artificial data-set using a reciprocal modeling approach. This approach utilizes machine learning models trained on sites under permanent land use to predict SOC stocks for similar sites where the land use had been changed. In addition, we extracted further empirical data from over 30 sites with land-use change in the temperate zone from a comprehensive meta-analysis. These two datasets were used to test the ability of the well-known SOC model RothC to simulate land-use change effects on SOC stocks. In these tests, we use the observed or predicted SOC stocks assumed at equilibrium to model the carbon input under permanent land use and corresponding SOC dynamics after land-use change. These modelled SOC dynamics are then compared with observed SOC stocks after land-use change. We will discuss opportunities and challenges of using process-based models to describe SOC dynamics after land-use change on regional to national scale.
更多查看译文
AI 理解论文
溯源树
样例
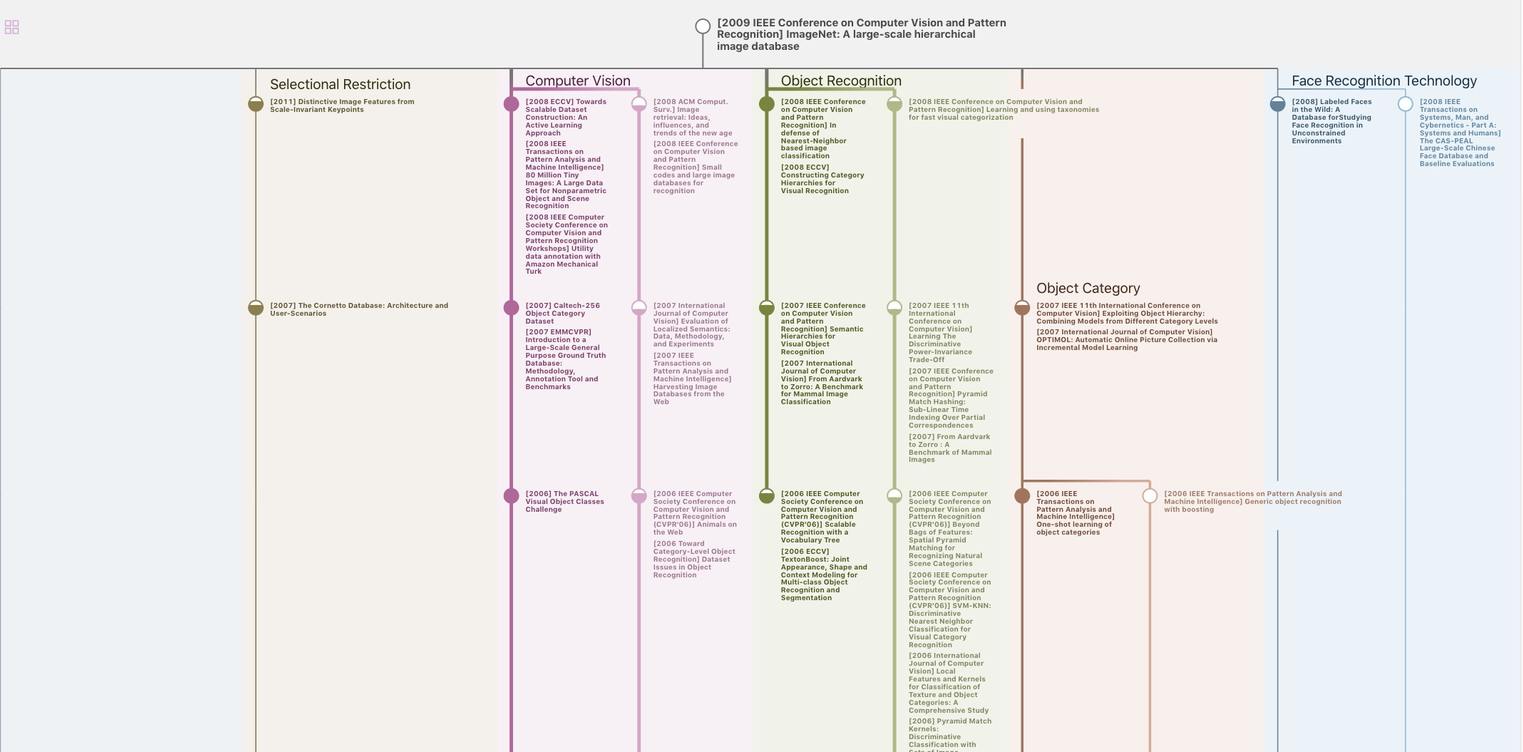
生成溯源树,研究论文发展脉络
Chat Paper
正在生成论文摘要