How does extreme point sampling affect non-extreme simulation in geographical random forest?
Earth Science Informatics(2024)
摘要
Spatial heterogeneity brings numerous uncertainties to training datasets in the modeling process. An arbitrary selection of training samples can result in a biased simulation. Although previous research provides a chance of reducing the degree of spatial variance through homogeneous divisions, detailed information regarding the impact of the configuration of divisions for training remains unknown. Moreover, few studies investigate the cross impact of extreme sampling on non-extreme simulation. Therefore, we extend previous research to investigate the cross impact and further examine whether the divisions of extremely high (EXH) and low (EXL) quantiles contribute equally to the simulation bias when employing the spatial stratified sampling. Statistical assessment demonstrates that the selection of extreme training sample does affect the non-extreme simulation. The model has the best performance (RMSE: 2.735, VE: 7.481, Bias: -0.033) when the least proportion (25
更多查看译文
关键词
Extreme point sampling,Geographical random forest,LiDAR,Canopy height
AI 理解论文
溯源树
样例
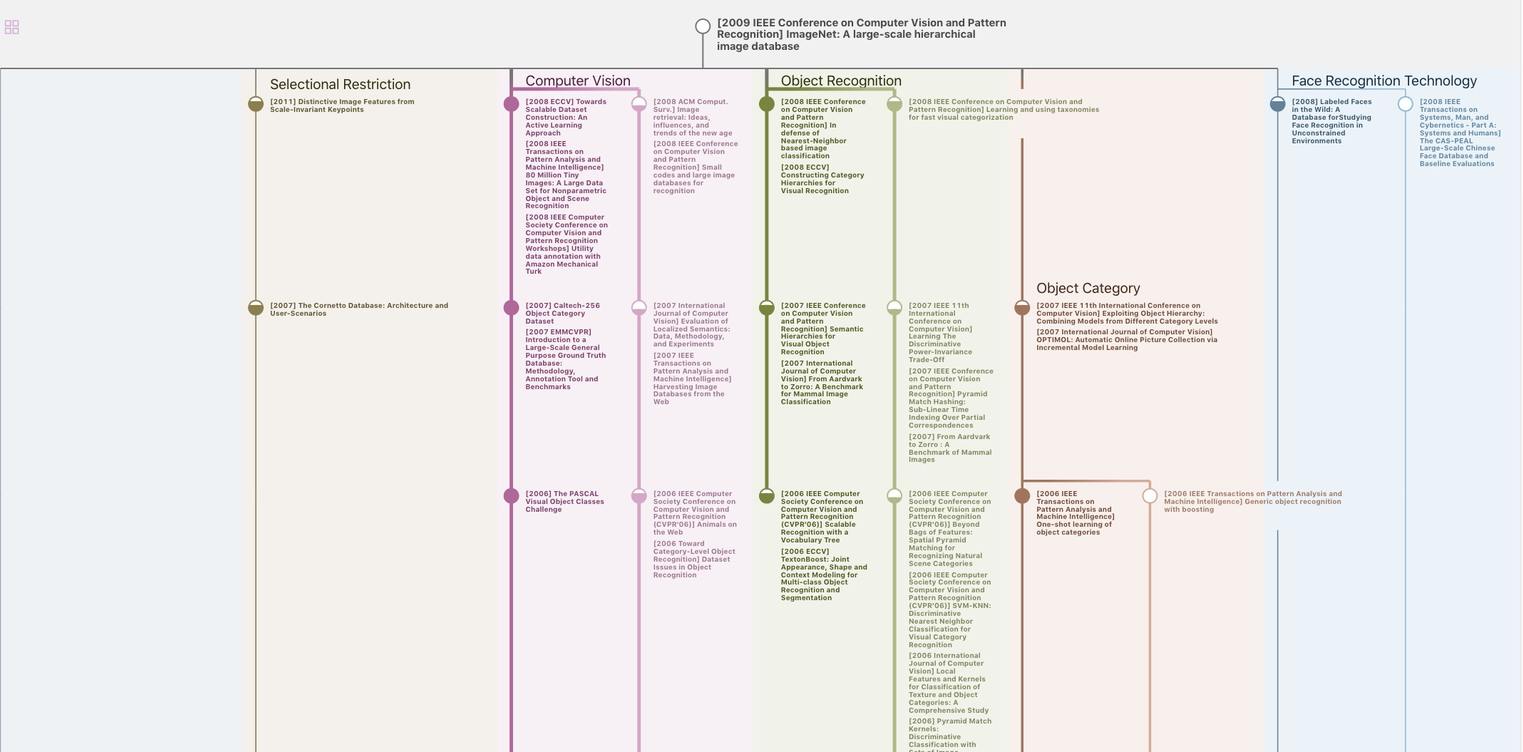
生成溯源树,研究论文发展脉络
Chat Paper
正在生成论文摘要