Uncertainty Quantification of Contrail Climate Impacts using Non-Intrusive Polynomial Chaos
crossref(2024)
摘要
Contrails are currently estimated to be one of the largest contributors to aviation climate forcing, with a cumulative effect roughly equivalent to that of the CO2 emitted by aviation.[3] Contrail properties are impacted by chemical and thermodynamic conditions in the exhaust and the meteorological environment. Currently, the state of meteorological and emissions data contains significant uncertainty.[4][2] As such, resulting contrail predictions and impact models are uncertain. This uncertainty is a detriment to aviation impacts reduction analysis. Meteorological data such as relative humidity (RH) is a key determining factor for the lifetime and subsequent radiative forcing (RF) of the contrail. Mitigation strategies necessitate consistent predictions of when and where contrails are formed, which contrails persist, and their respective RF effects.[2] Identifying model parameter sensitivities to formation and meteorological condition uncertainty will improve the effectiveness of policies designed to reduce contrail climate impacts. To address this problem we examine two existing models: the Aircraft Plume Chemistry, Emissions, and Microphysics Model (APCEMM), and the Contrail Cirrus Prediction Model (CoCiP).[1][5] Both model the chemical and physical evolution of an aircraft plume, using differing methods. Comparison of the models and their outcomes under different conditions will contribute to the characterization of current contrail models and identification of areas most sensitive to input parameters. Inputs of interest are soot concentration, sulfur concentration, and the RH field. Uncertainty quantification (UQ) for the existing models is limited, and largely relies on Monte-Carlo analysis. Computationally expensive models such as APCEMM cannot be appropriately characterized by this type of analysis. A non-intrusive Polynomial Chaos Expansion (PCE) method is implemented to better characterize the relationship between uncertain inputs and resulting impacts predicted by APCEMM and CoCiP. This technique is commonly used for UQ of results obtained from expensive computational models. PCE is a data-driven regression-based technique that relies on spectral polynomials as basis-functions. Outputs of few (expensive) numerical simulations estimate the PCE coefficients within a regression framework combined with regularization techniques. The resulting surrogate efficiently predicts outcomes given novel inputs, circumventing the use of the expensive model to produce analysis. In this work we introduce a new method of UQ for contrail impacts, applied to two existing contrail impact models. The resulting surrogates explore the impact of uncertainty in soot and sulfur concentrations as well as RH field. Our goal in this work is to establish quantitative relationships between input parameter uncertainty and climate impacts, identifying output sensitivity to input perturbations. We present a set of tests on the 5 most travelled flight paths for the 5 most common aircraft, including spatially dependent and independent uncertain variables. [1]Thibaud Fritz et. al. The role of plume-scale processes in long-term impacts of aircraft emissions. 10.5194/acp-20-5697-2020 [2]Klaus Gierens et al. How Well Can Persistent Contrails Be Predicted? 10.3390/aerospace7120169 [3]D. Lee et al. The contribution of global aviation to anthropogenic climate forcing for 2000 to 10.1016/j.atmosenv.2020.117834 [4]Vincent Meijer et al. Contrail coverage over the United States before and during the COVID-19 pandemic. 10.1088/1748-9326/ac26f0 [5]Ulrich Schumann. A contrail cirrus prediction model. 10.5194/gmd-5-543-2012
更多查看译文
AI 理解论文
溯源树
样例
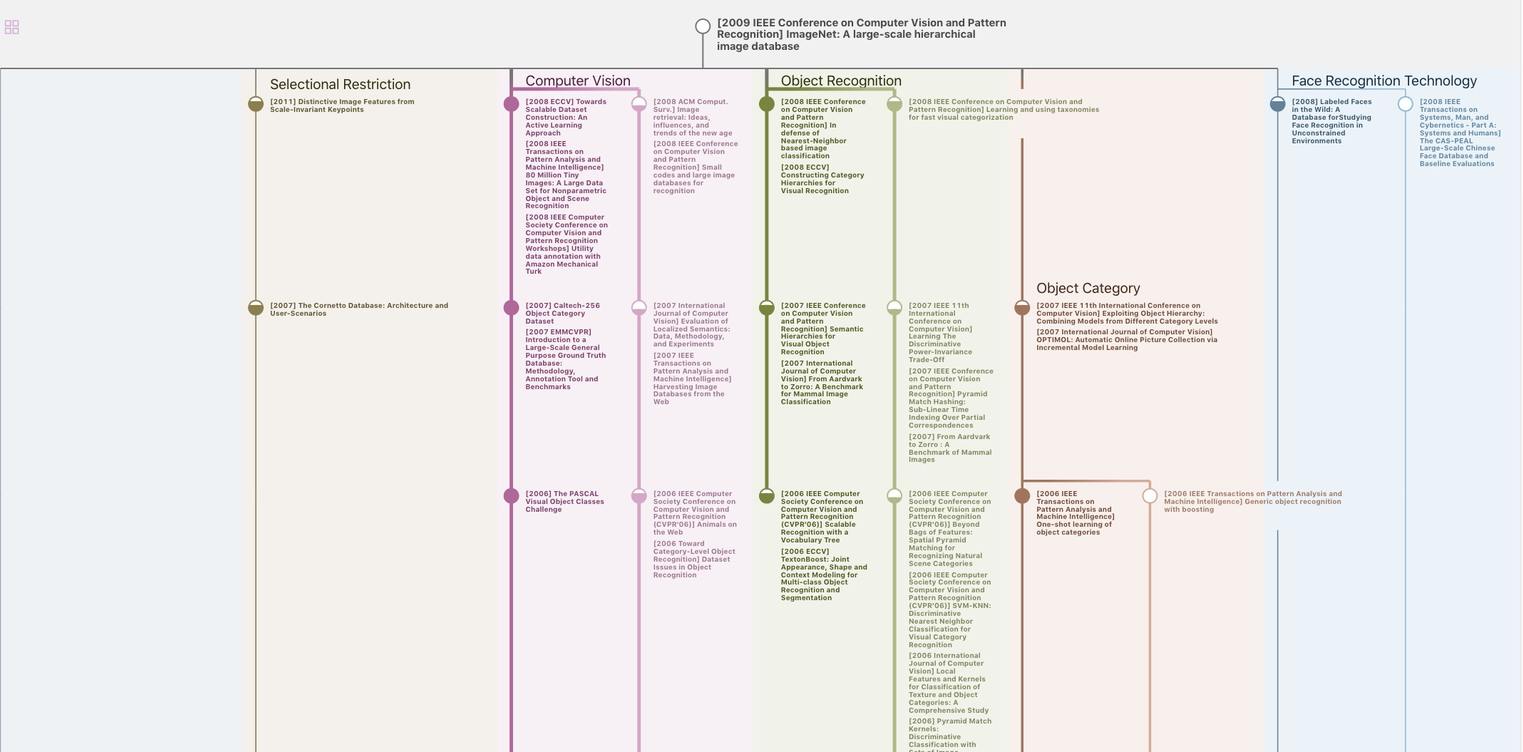
生成溯源树,研究论文发展脉络
Chat Paper
正在生成论文摘要