A new prognostic model for accurate assessment of hepatocellular carcinoma risk using RNA editing data and unsupervised machine learning
NEUROCOMPUTING(2024)
摘要
A-to-I RNA editing is a long-known driving factor to the progression of hepatocellular carcinoma (HCC); however, its importance is often neglected in HCC subtyping and the modeling of prognosis, partially due to the high dimensional nature of the editing data. In this paper, we first obtain a comprehensive A-to-I RNA editing profile for HCC, among which two editing sites, IGFBP7 I387M (chr4:57110120) and SUMF2 A98G (chr7:56078974), are identified as having the driving function potential. Then, we exploit the clinical-related RNA editing profile in combination with the gene expressions to develop a new prognostic model for HCC. To preserve the inner structure of this editing profile in a latent space that has a much lower dimension, we employ variational autoencoder (VAE), an unsupervised learning neural network applied for the first time to compress the RNA editing profile. Empowered by VAE, we are able to cluster the HCC patients efficiently according to their editing profile, among which a group of patients with immune dysregulation and enrichment in cell proliferation and cancer-related pathways are identified. Based on the editing-related subtyping, we develop a prognostic model that consists of an easy-computing risk score and an adaptively trained threshold. Validations using three independent cohorts (LIHC, LIRI, and GSE14520) confirm that the new model is robust, sensitive, and specific. Furthermore, it is more accurate in prediction than six state -of -the -art competitors. The model is also found applicable to 18 more cancers besides HCC, which suggests a strong ability in generalization. To facilitate clinical usage, we develop a nomogram, drug targets, prognosis-associated genes, and relevant pathways are also identified, which pave the way for upcoming studies on HCC's molecular mechanism, therapeutic targets, and many others.
更多查看译文
关键词
Variational autoencoder (VAE),Prognostic model,A-to-I RNA editing,Hepatocellular carcinoma
AI 理解论文
溯源树
样例
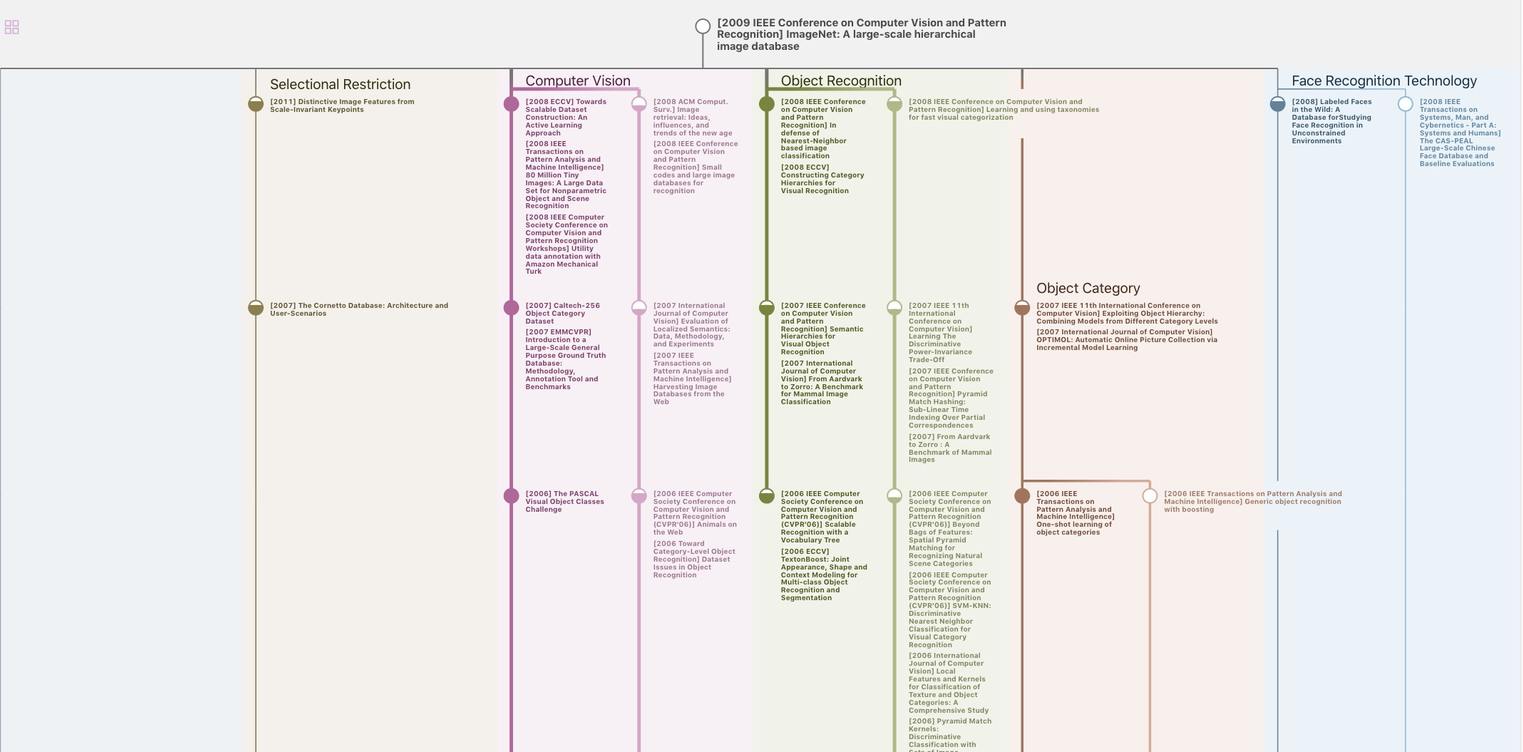
生成溯源树,研究论文发展脉络
Chat Paper
正在生成论文摘要