Optimal blending of ensemble forecasts for probabilistic precipitation nowcasting
crossref(2024)
摘要
Precipitation nowcasting is essential for mitigating the negative effects of severe weather, necessitating accurate and timely forecasts. Our study introduces a novel Local Ensemble Transform Kalman Filter (LETKF)-based blending approach that effectively combines ensemble forecasts to provide cheap probabilistic nowcasts. Our method optimally weights ensemble probabilities by considering precipitation/radar observations as the ground truth. It shifts computed weights in time and refines neighborhood probabilities (NPs). The upscaling step for computing NPs introduces two free parameters, neighborhood size and precipitation rate, which can be selected based on the forecasters needs. Demonstrated within GeoSphere Austria's regional forecasting system using AROME ensemble forecasts over Austria, our technique was especially beneficial for short lead times of up to 2 hours. Longer lead times require to incorporate signal propagation, which is possible by shifting weights in space and time. Our study underscores the effectiveness of data assimilation techniques in enhancing ensemble blending. The proposed approach affordably improves the robustness and accuracy of short-term probabilistic forecasts and holds the potential for extending it to multi-model ensemble blending.
更多查看译文
AI 理解论文
溯源树
样例
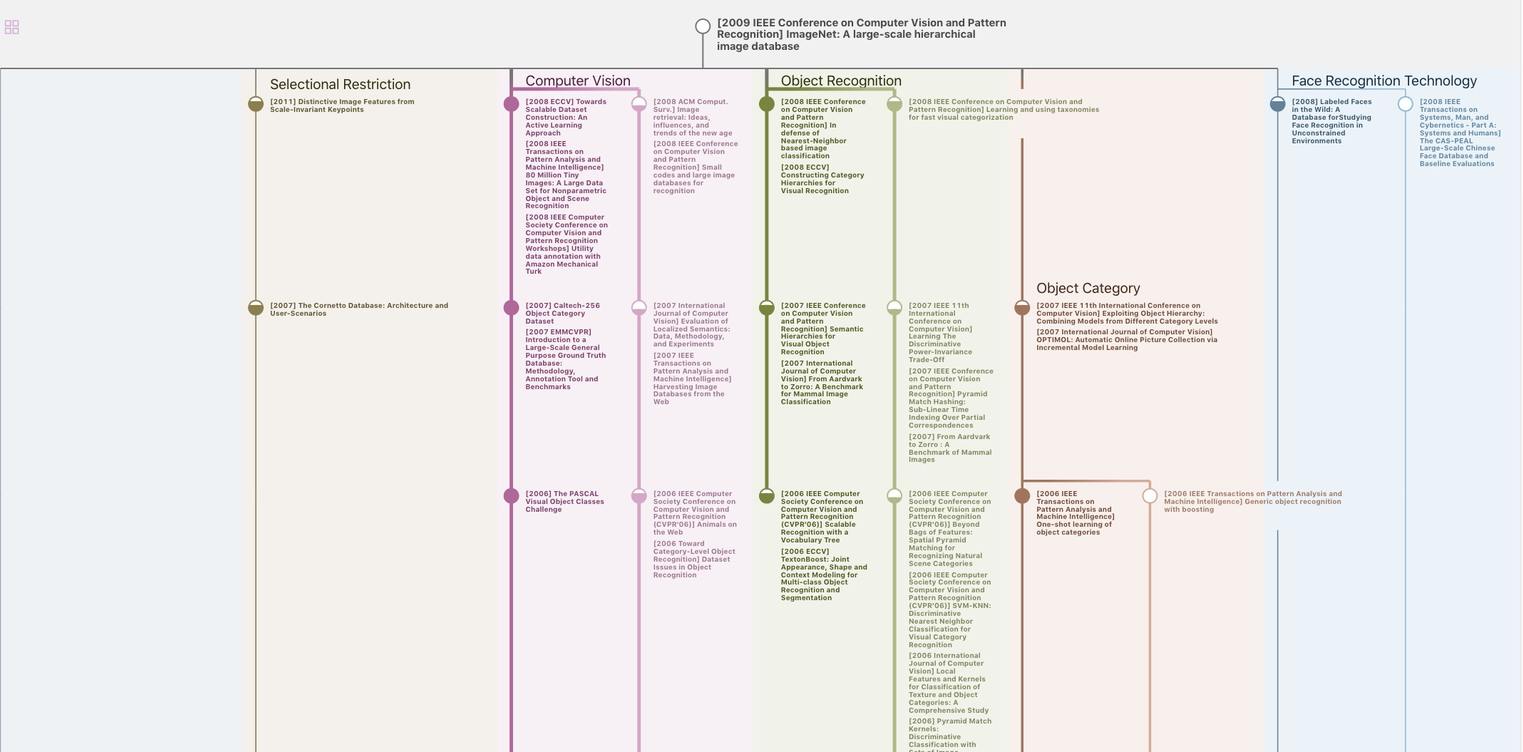
生成溯源树,研究论文发展脉络
Chat Paper
正在生成论文摘要