Introducing a fully differentiable, fully distributed Rainfall-Runoff Model
crossref(2024)
摘要
Traditional hydrology simulates rainfall-runoff dynamics by means of process-based models (PBMs), which are derived from physical laws. The models exhibitrealistic behavior. Their internal states can by directly interpreted, because theyreflect the modeled current state of the hydrological dynamics. Natural processesin general are very complex, though, such that is is simply impossible to modelevery aspect in detail. In the case of hydrology, for example, anthropological in-fluences, such as the exact influence of the sewer system, as well as natural factors,such as soil and rock types and structures, are extremely hard to model in all theirdetails. As a result, high uncertainty remains about the models’ necessary compo-nents and their parameterizations, leaving room for improvement Sit et al. [2020],Nearing et al. [2021]. Data-driven approaches, like deep neural networks (DNNs),offer an alternative. They are trained on large amounts of data by gradient descentvia automatic differentiation. This enables them to automatically discover rela-tionships in the training data, which often leads to superior performance Kratzertet al. [2018], Shen [2018]. Due to the DNNs’ complexity, however, these rela-tionships are hard to investigate and often fail to respect physical laws. Hybridmodeling combines both approaches in order to benefit from their respective ad-vantages. In this work, we present a physics-inspired, fully differentiable and fullydistributed rainfall-runoff model to predict river discharge from precipitation. OurDNN architecture consists of a land module and a river module. The land mod-ule receives RADOLAN-based precipitation data and propagates runoff laterallyover a regular grid (1km2 grid size) taking land surface structure information intoaccount. Runoff is then captured as input to the river module, which mimics theactual river network by means of a graph neural network. Due to the involved,physically motivated inductive biases, our model can be trained end-to-end fromthe RADOLAN data as the main input and sparse discharge data as output. Weshowcase our model on the Neckar river catchment in South Germany, achiev-ing NSE values of 0.88 and 0.84 when we predict 1 and 10 days into the future,respectively. In contrast, persistence yields NSE values of 0.5 and 0.06 for the cor-responding forecast horizons. Due to our model’s differentiability we expect to beable to infer the origin of measured discharge or turbidity—and thus erosion—inthe near future. We thus hope that this information could be used to create policiesthat mitigate both the danger of floods and extreme erosion.
更多查看译文
AI 理解论文
溯源树
样例
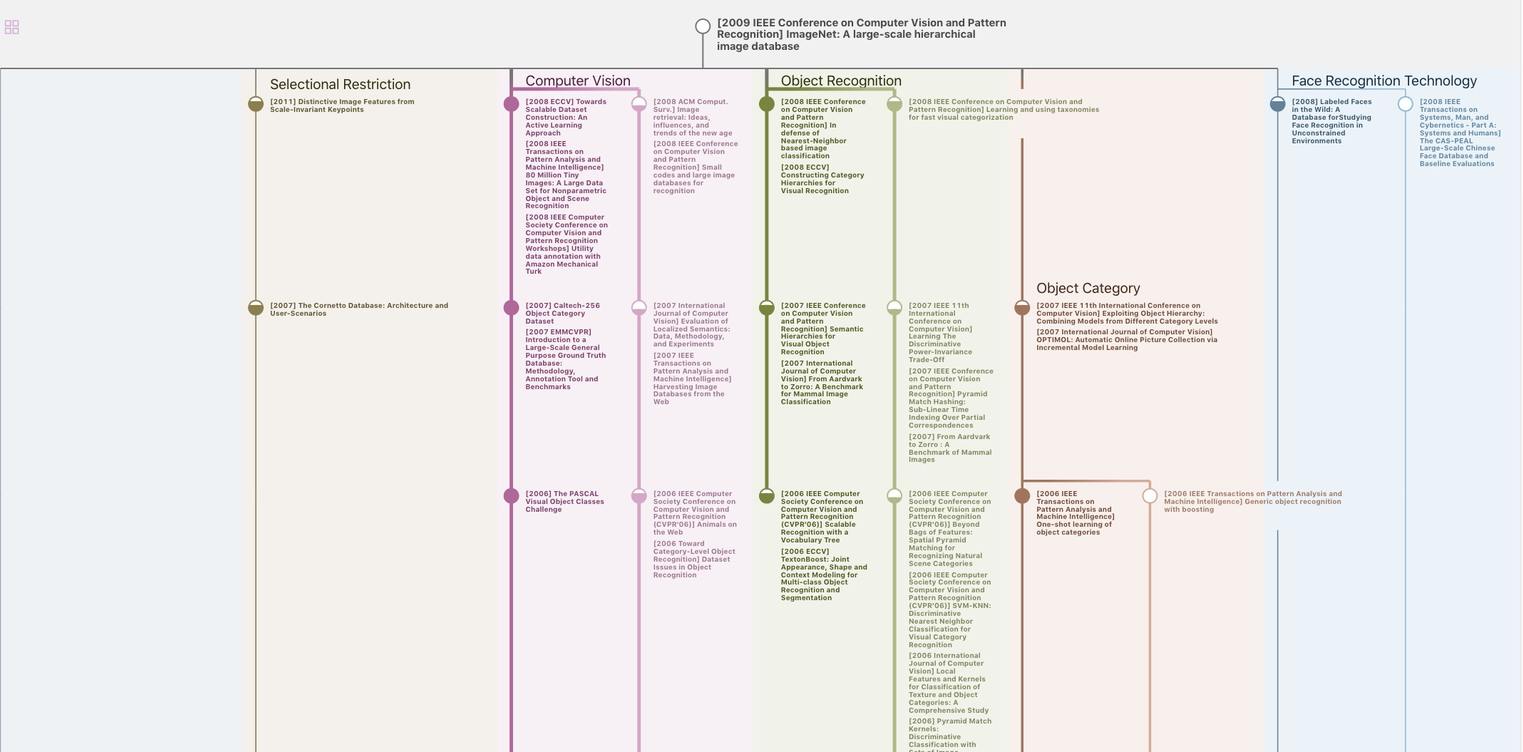
生成溯源树,研究论文发展脉络
Chat Paper
正在生成论文摘要