Identification of circadian rhythm-related gene classification patterns and immune infiltration analysis in heart failure based on machine learning
Heliyon(2024)
摘要
Background
Circadian rhythms play a key role in the failing heart, but the exact molecular mechanisms linking changes in the expression of circadian rhythm-related genes to heart failure (HF) remain unclear.
Methods
By intersecting differentially expressed genes (DEGs) between normal and HF samples in the Gene Expression Omnibus (GEO) database with circadian rhythm-related genes (CRGs), differentially expressed circadian rhythm-related genes (DE-CRGs) were obtained. Machine learning algorithms were used to screen for feature genes, and diagnostic models were constructed based on these feature genes. Subsequently, consensus clustering algorithms and non-negative matrix factorization (NMF) algorithms were used for clustering analysis of HF samples. On this basis, immune infiltration analysis was used to score the immune infiltration status between HF and normal samples as well as among different subclusters. Gene Set Variation Analysis (GSVA) evaluated the biological functional differences among subclusters.
Results
13 CRGs showed differential expression between HF patients and normal samples. Nine feature genes were obtained through cross-referencing results from four distinct machine learning algorithms. Multivariate LASSO regression and external dataset validation were performed to select five key genes with diagnostic value, including NAMPT, SERPINA3, MAPK10, NPPA, and SLC2A1. Moreover, consensus clustering analysis could divide HF patients into two distinct clusters, which exhibited different biological functions and immune characteristics. Additionally, two subgroups were distinguished using the NMF algorithm based on circadian rhythm associated differentially expressed genes. Studies on immune infiltration showed marked variances in levels of immune infiltration between these subgroups. Subgroup A had higher immune scores and more widespread immune infiltration. Finally, the Weighted Gene Co-expression Network Analysis (WGCNA) method was utilized to discern the modules that had the closest association with the two observed subgroups, and hub genes were pinpointed via protein-protein interaction (PPI) networks. GRIN2A, DLG1, ERBB4, LRRC7, and NRG1 were circadian rhythm-related hub genes closely associated with HF.
Conclusion
This study provides valuable references for further elucidating the pathogenesis of HF and offers beneficial insights for targeting circadian rhythm mechanisms to regulate immune responses and energy metabolism in HF treatment. Five genes identified by us as diagnostic features could be potential targets for therapy for HF.
更多查看译文
关键词
Heart failure,Circadian rhythm,Machine learning,Immune infiltration,Unsupervised clustering,Bioinformatics
AI 理解论文
溯源树
样例
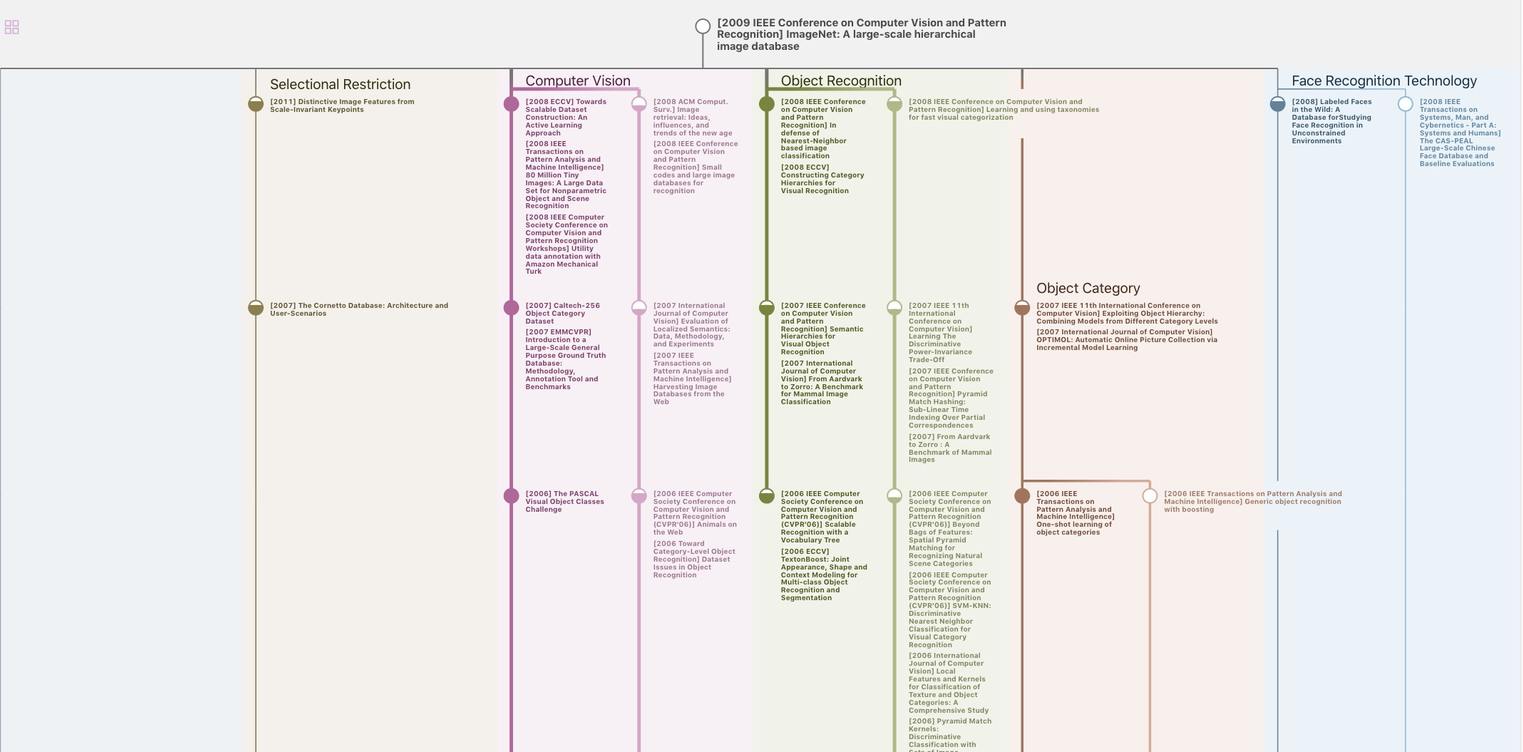
生成溯源树,研究论文发展脉络
Chat Paper
正在生成论文摘要