An interpretable 3D multi-hierarchical representation-based deep neural network for environmental, health and safety properties prediction of organic solvents
GREEN CHEMISTRY(2024)
摘要
The interpretability and accuracy of deep-learning-based predictive models play a pivotal role in accelerating computer-aided green product design considering environmental, health, and safety (EH&S) impacts. Recently, molecular graph-based hybrid representation methods have demonstrated comparable or superior abilities to other molecular representations. However, existing molecular graph-based hybrid representation methods incorporate only 2D-based atom-level, bond-level, or molecule-level features while neglecting the molecular geometry, also known as 3D spatial structure information, which is crucial for determining molecular properties. Moreover, existing molecular graph-based hybrid representations lack consideration of knowledge in the chemistry domain, which can improve the interpretability of the predictive models. To this end, a 3D multi-hierarchical representation-based deep neural network (3D-MrDNN) architecture, simultaneously integrating directed message passing neural network learned representation, chemically synthesizable fragment features, and molecular 3D spatial information, is established for the prediction of EH&S properties. The results of predictive performance and ablation studies indicate that the proposed model exhibits decent predictive ability for EH&S properties. Chemically synthesizable fragments are utilized to integrate chemical knowledge into the proposed 3D-MrDNN architecture, the interpretability of which enables chemists to find the key molecular fragments for designing target products with better EH&S performance. A 3D multi-hierarchical representation-based deep neural network (3D-MrDNN) architecture for prediction of the environmental, health and safety properties of organic solvents.
更多查看译文
AI 理解论文
溯源树
样例
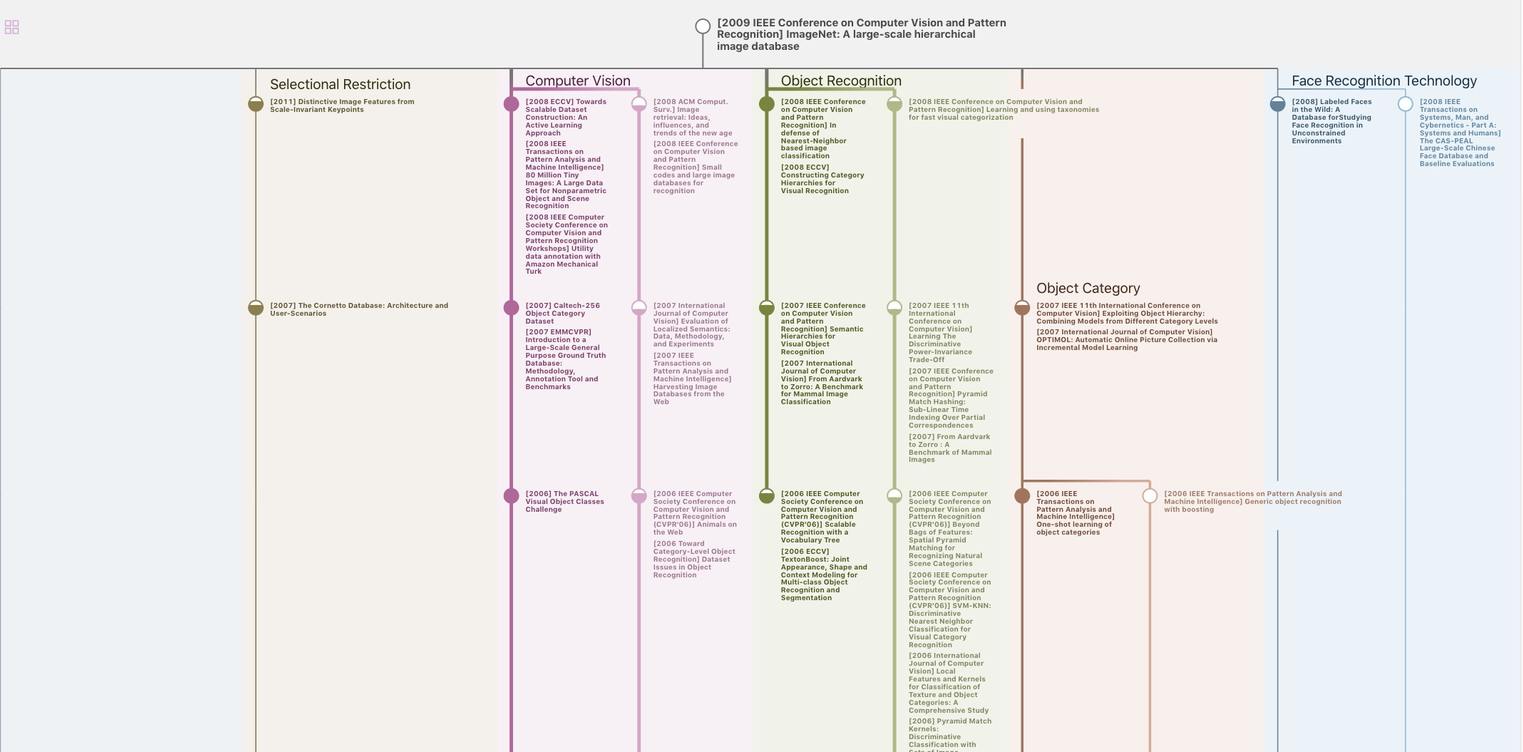
生成溯源树,研究论文发展脉络
Chat Paper
正在生成论文摘要