Assessment of ensemble selection methods aligning decadal climate variability in a perfect-model framework
crossref(2024)
摘要
Multi-model ensembles of climate simulations often fail to correctly represent the climate evolution of the next decades due to the dominance of internal variability uncertainty in the near-term future. With the objective to solve this issue, in recent years new constraining methods have been developed. These methods aim to select members from the ensemble of climate simulations that have their variability and forced response most in phase with an observational or climate prediction reference. Through an evaluation of the performance of the different methods (Befort et al. 2020, Mahmood et al. 2021, Mahmood et al. 2022) against observations, we find that applying them directly to the CMIP6 multi-model ensemble exhibits high sensitivity to variations in the different parameters that define the methods. This complicates the interpretability of the results. We will illustrate the results of a study where we simplify the complexity of the ensemble selection methods by applying them to a single-model large ensemble. We use a perfect-model approach where, in turns, one of the members plays the role of the constraining and evaluation reference. In this study there is only one climate system involved, the one of the climate model chosen, and the differences between models and biases against observations no longer influences the results of the selection methods. Therefore, through this idealized approach, the mechanisms of the different selection methods can be better isolated and studied. Furthermore, this study offers perspectives of the potential improvements that could be expected from these methods and opens the door to finding ways of optimizing the metrics and approaches used to constrain a climate projections ensemble.
更多查看译文
AI 理解论文
溯源树
样例
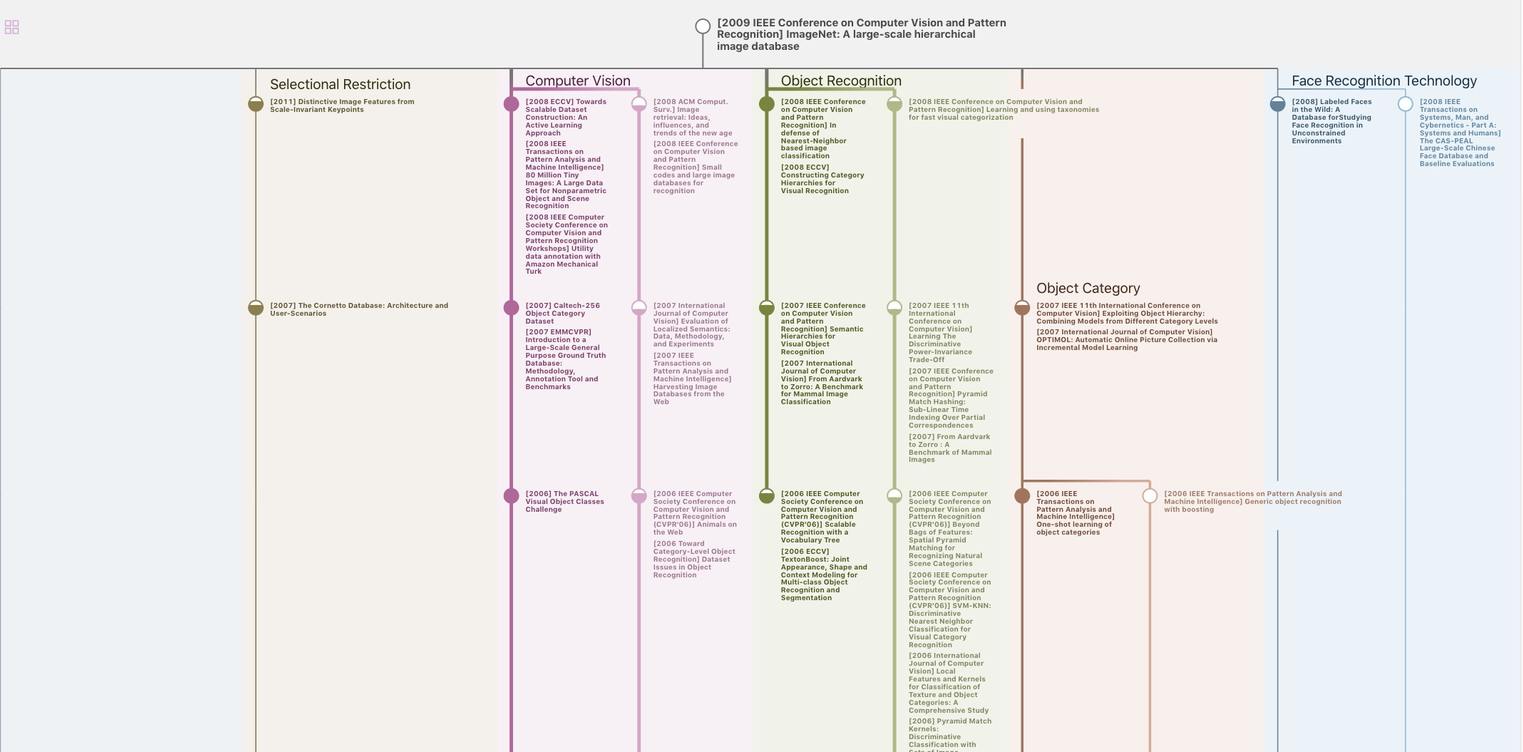
生成溯源树,研究论文发展脉络
Chat Paper
正在生成论文摘要