Encoding the drag-based model in the artificial intelligence training process to predict CMEs’ travel times
crossref(2024)
摘要
The study of space weather impacts of coronal mass ejections (CMEs) requires the formulation, implementation and validation of predictive approaches to address issues such as the arrival of CMEs to Earth and, if so, its arrival time and speed. The problem of predicting the CMEs’ travel times has been addressed by means of empirical models, physics-based models, and artificial intelligence techniques. In this talk, we propose a physics-driven artificial intelligence (AI) method, in which we encode physical information into the process of neural network training. Specifically we include the drag-based model in the definition of the loss functions to minimize during the training process of a cascade of two neural networks fed with both remote sensing and in situ data. We show that including physical information in the AI architecture improves its predictive capabilities and the proposed physics-driven AI method leads to more accurate and robust results for the CMEs’ travel time prediction with respect to the purely-data driven AI approach.
更多查看译文
AI 理解论文
溯源树
样例
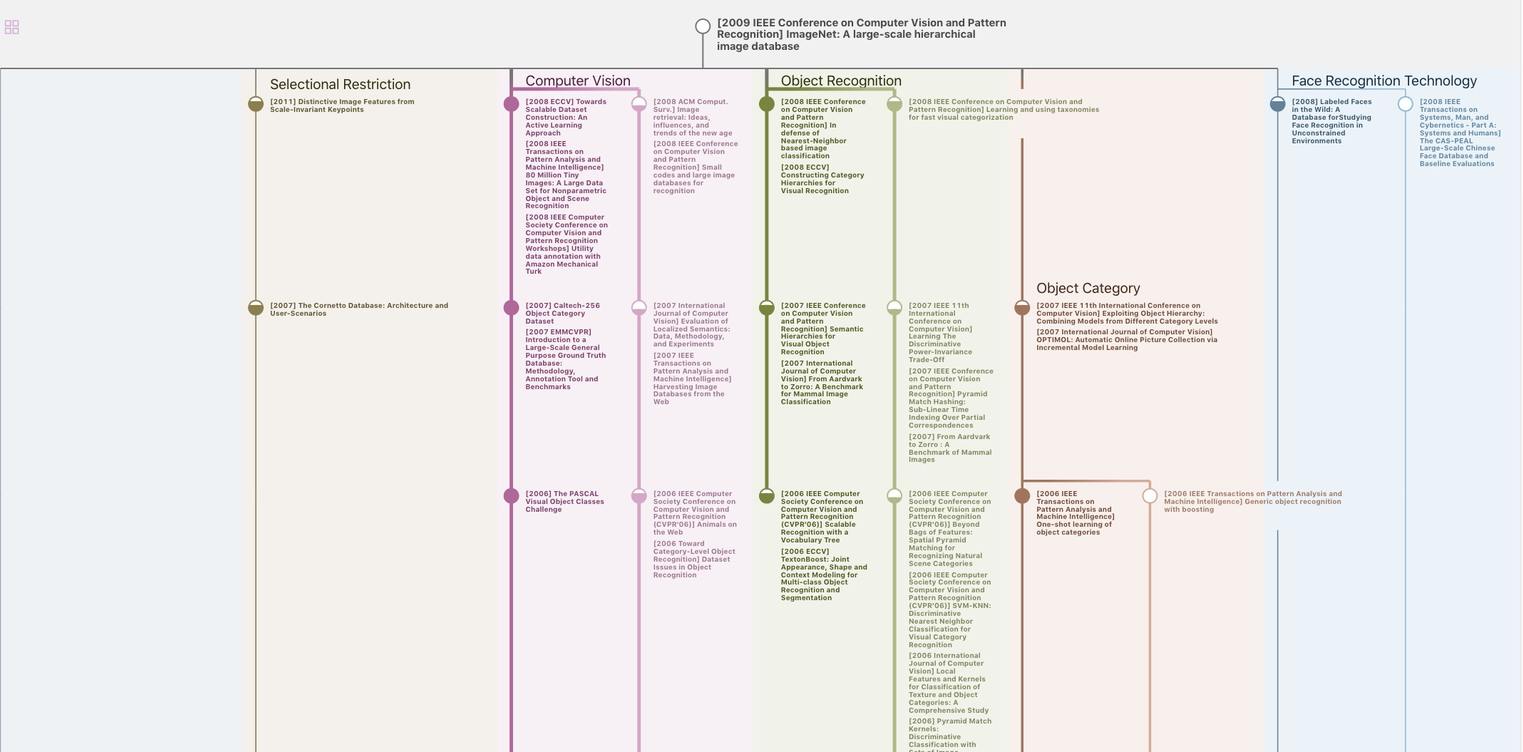
生成溯源树,研究论文发展脉络
Chat Paper
正在生成论文摘要