An Efficient Workflow for Airborne Electromagnetic Data Processing for Advanced Applications.
crossref(2024)
摘要
Airborne electromagnetics made its first successful entry into mineral exploration applications, mainly by interpreting anomalies in the data. Airborne EM methods will increasingly be used for more advanced large-scale applications, such as mapping the fresh saltwater interface. Every step in the data interpretation is crucial in this regard. We believe the state-of-the-art (such as in [1]) is a good starting point for an initial data interpretation, though not the endpoint. We propose a workflow for airborne EM processing, where the current state-of-the-art may be too limited for your advanced applications. It consists out of four steps: 1. First processing with Quasi(or pseudo)-2D/3D inversion (with your favourite or typical smooth inversion scheme, such as in [1]) 2. If the sharpness of the obtained inversion model is inappropriate, perform a tunable regularization with the inversion model from step 1 as the initial model. This can be achieved with wavelet-based regularization schemes [2], where prior information can be incorporated by the choice of the wavelet or, in the absence of prior information, the non-uniqueness can be assessed with the ensemble approach. 3. Where is multidimensionality a possible issue? In steps 1 and 2, we have used a 1D forward approximation, potentially yielding multidimensionality issues. An appraisal method, such as [3], enables the assessment of an inversion model obtained with 1D forward approximation for areas that do not fit the true multidimensionality of the observed data because it deviates from the 1D assumption. 3. Re-interpret zones with significant multidimensionality with either full 3D simulations or a surrogate model [4], replacing the computationally demanding 3D full simulations. [1] Siemon, B., Auken, E., & Christiansen, A. V. (2009). Laterally constrained inversion of helicopter-borne frequency-domain electromagnetic data. Journal of Applied Geophysics, https://doi.org/10.1016/j.jappgeo.2007.11.003. [2] Deleersnyder, W., Maveau, B., Hermans, T., & Dudal, D. (2023). Flexible quasi-2D inversion of time-domain AEM data, using a wavelet-based complexity measure. Geophysical Journal International, https://doi.org/10.1093/gji/ggad032. [3] Deleersnyder, W., Dudal, D., & Hermans, T. (2022). Novel airborne em image appraisal tool for imperfect forward modeling. Remote Sensing, https://doi.org/10.3390/rs14225757. [4] Deleersnyder, W., Dudal, D., & Hermans, T. (2023, February). Machine learning assisted fast forward 3D modelling for time-domain electromagnetic induction data–lessons from a simplified case. In EGU General Assembly 2023, Location: Vienna, Austria, https://doi.org/10.5194/egusphere-egu23-12015.
更多查看译文
AI 理解论文
溯源树
样例
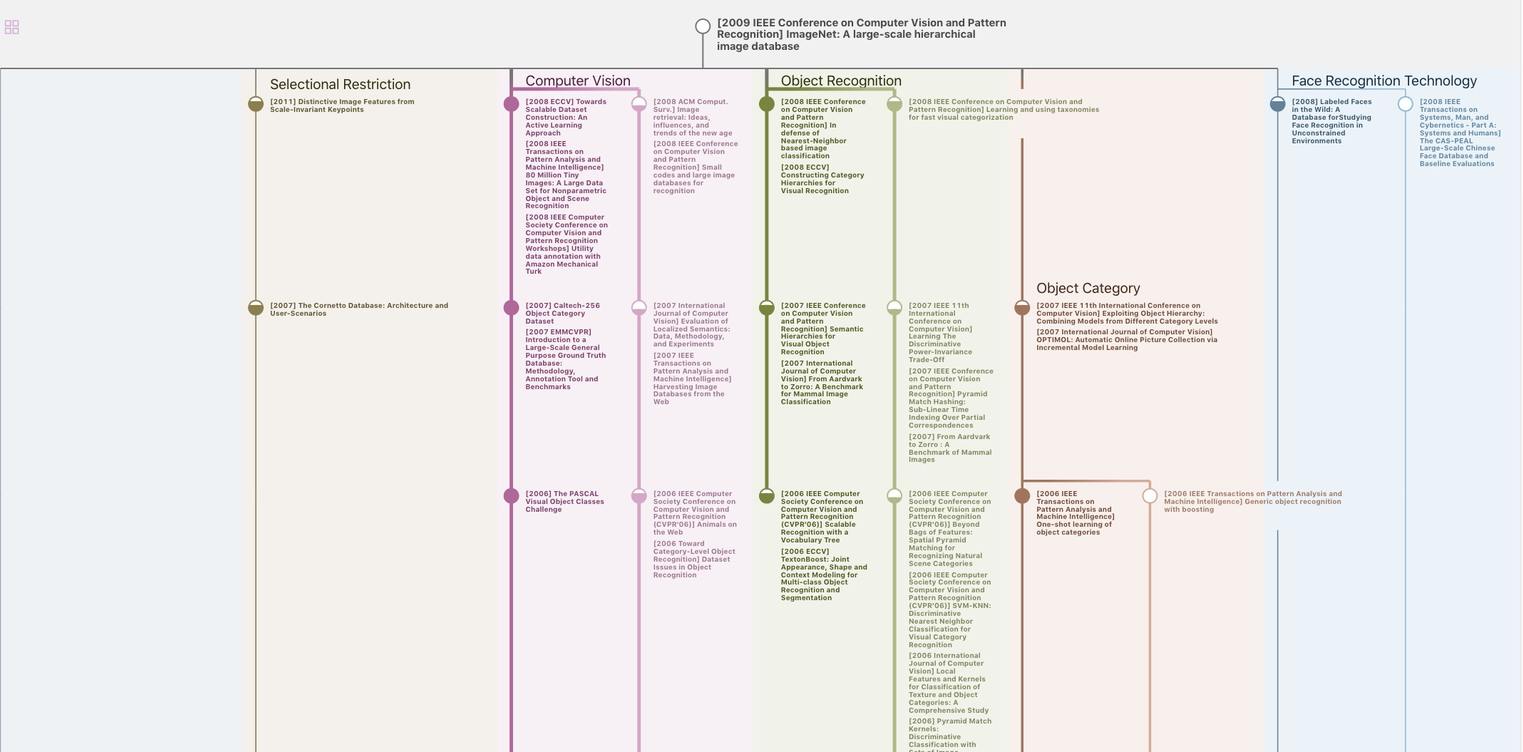
生成溯源树,研究论文发展脉络
Chat Paper
正在生成论文摘要