Rock glacier inventorying and validation across the Hindu Kush Himalaya from deep learning and high-resolution images
crossref(2024)
摘要
Rock glaciers are important to monitor due to their importance (i) as indicators of permafrost distribution, (ii) as integral components of the mountain hydrological systems, (iii) as indicators of permafrost temperature and pore-water pressure reflected in their kinematic behaviour under climate change and (iv) as potential triggers for geohazards such as rockfalls, debris flows, and lake outbursts related to their destabilization. Understanding these aspects requires accurate, systematic and updated rock glacier inventories. Currently, these remain patchy over extensive areas of High Mountain Asia. In a recent study, we presented a deep-learning-based approach for mapping rock glaciers across the Tibetan Plateau based on Deeplabv3+ deep learning network, trained using visually consistent and cloud-free Planet Basemaps and multi-source rock glacier inventories from multiple regions. This resulted in 44,273 rock glaciers covering a total area of ~6,038 km2, including both intact and relict types. In this work, we used the well-trained model to extend the mapping of rock glaciers over the entire Hindu-Kush Himalaya (HKH) range, resulting in an additional 46,425 rock glaciers candidates covering an area of ~5,700 km2. The raw number of rock glaciers mapped is significantly higher than previous estimates based on upscaled samples. We first screened the deep learning output based on AW3D30 elevation data to remove outliers and then validated the remaining candidates over several key regions in HKH (Manaslu, Khumbu and Ladakh regions) using independent satellite data from Pléiades, SPOT etc. The now complete inventory over the Tibetan Plateau-KHK constitutes a significant contribution to the IPA RGIK action group and serves as a benchmark dataset for modeling and monitoring the state of permafrost in a changing climate. Furthermore, this provides an important dataset for training deep learning models for global application.
更多查看译文
AI 理解论文
溯源树
样例
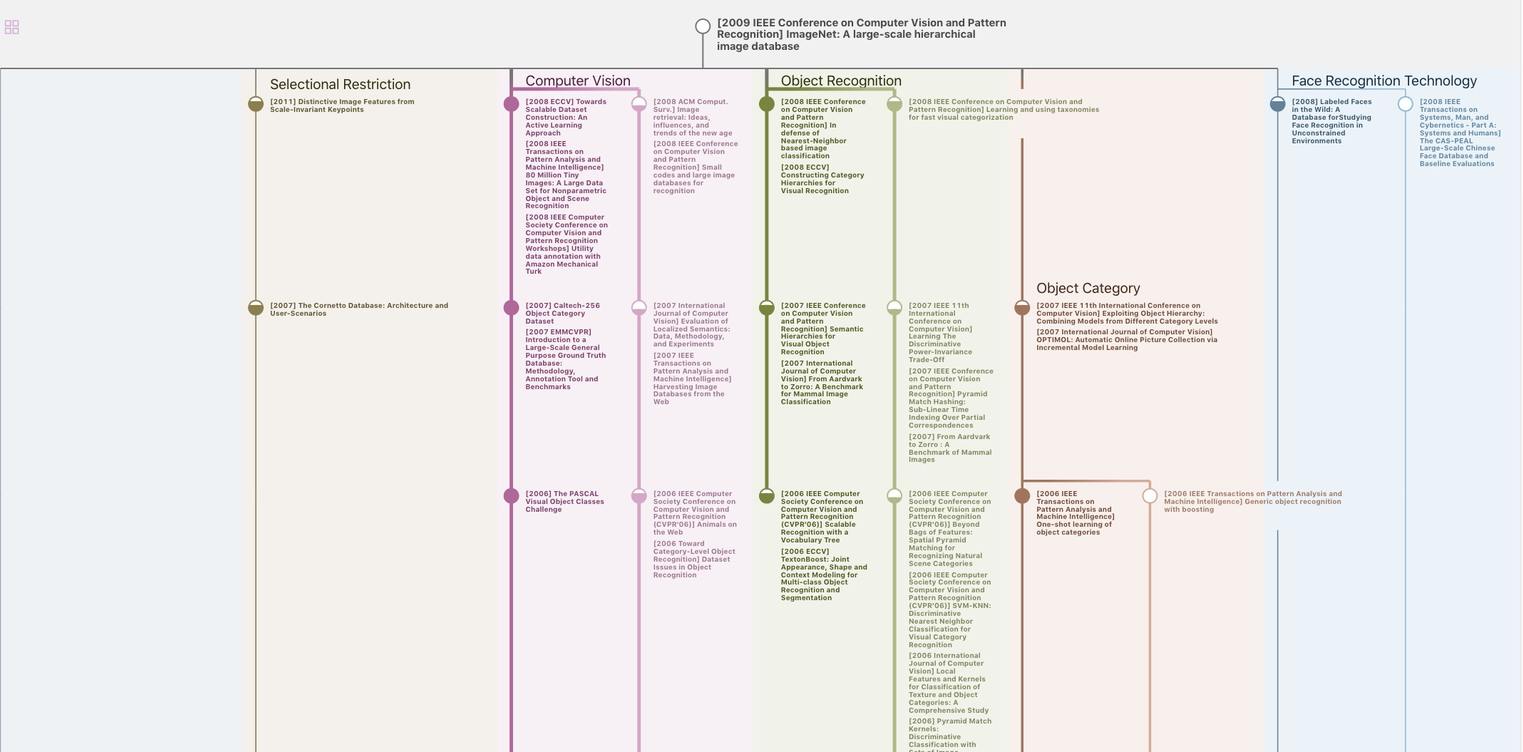
生成溯源树,研究论文发展脉络
Chat Paper
正在生成论文摘要