Satellite fault tolerant attitude control based on expert guided exploration of reinforcement learning agent
JOURNAL OF EXPERIMENTAL & THEORETICAL ARTIFICIAL INTELLIGENCE(2024)
摘要
This research provides a method that accelerates learning and avoids local minima to improve the policy gradient algorithm's learning process. Reinforcement learning has the advantage of not requiring a model. Consequently, it can improve control performance, mainly when a model is generally unavailable, such as when an error occurs. The proposed method efficiently and expeditiously investigates the action space. First, it quantifies the resemblance between agents' and traditional controllers' actions. Then, the principal reward function is modified to reflect this similarity. This reward-shaping mechanism guides the agent to maximize its return via an attractive force during the gradient ascent. To validate our concept, we establish a satellite attitude control environment with a similarity subsystem. The outcomes demonstrate the effectiveness and robustness of our method.
更多查看译文
关键词
Fault-tolerant control,machine learning,reinforcement learning,policy gradient algorithms,reward shaping
AI 理解论文
溯源树
样例
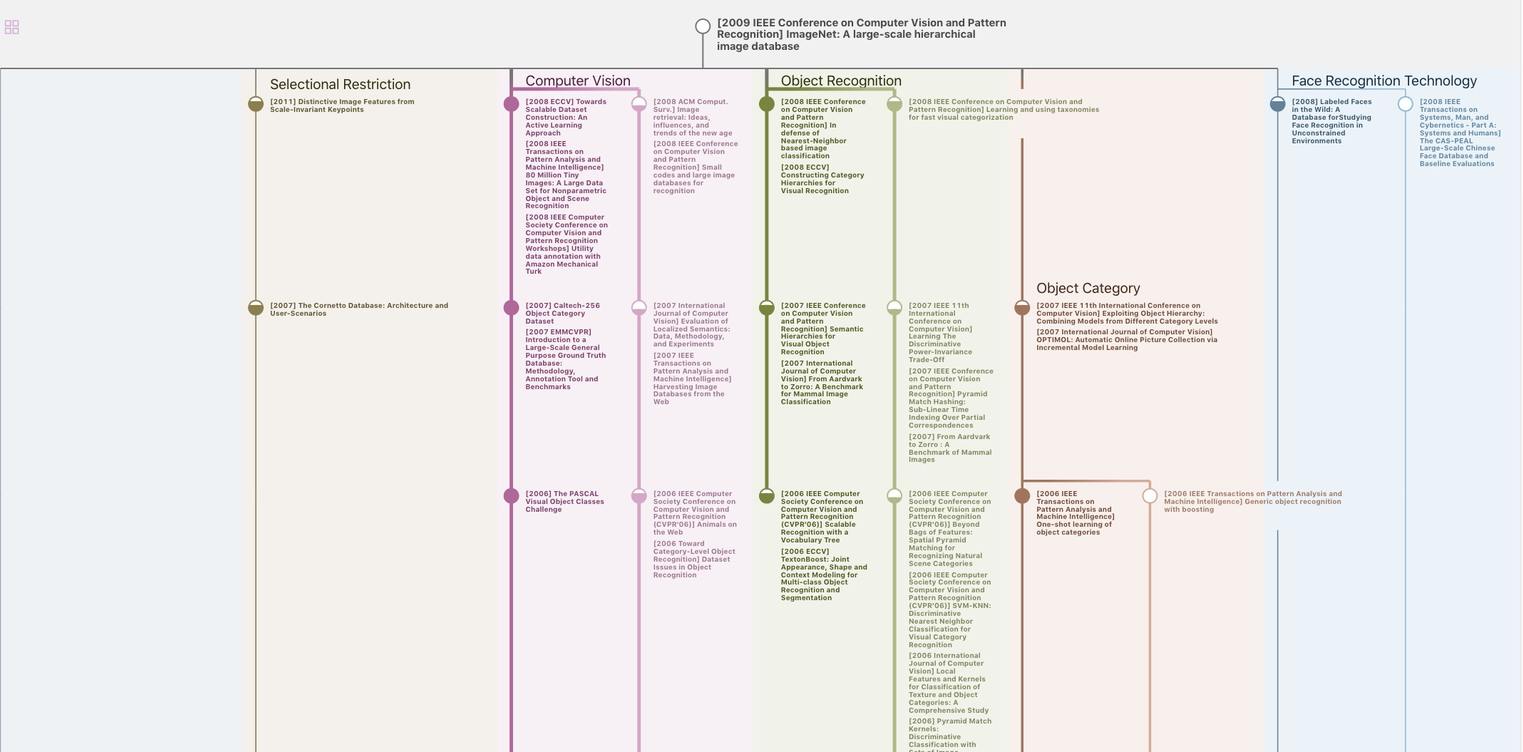
生成溯源树,研究论文发展脉络
Chat Paper
正在生成论文摘要