Statelets extraction-guided contrastive learning for detection of control performance degradation with varying degrees
ADVANCED ENGINEERING INFORMATICS(2024)
摘要
Detection of control performance degradation (CPD) in time series plays a vital role in modern industry. However, most CPD detection methods tend to neglect the following recognitions: 1) control performance is often assessed over small segments of the time series rather than the long-term entire one, and 2) CPD may exhibit different degrees in practice, resulting in the time-varying data distribution problem. These two considerations necessitate the development of algorithms that can extract information-rich small segments, defined as statelets, to discriminate the control performance with varying degrees. To this end, a Statelets Extraction-based Contrastive Learning (SECL) model is proposed for the detection of CPD. First, a varying-length statelet generation algorithm is developed to split the original multivariate time series into multiple statelet candidates with different time lengths, which can avoid the problem of information-rich small segments being obscured by the long-term trend of the time series. A contrastive learning encoder is then constructed to map statelet candidates into a uniform feature space with the designed triplet loss, keeping features of similar statelets tight and features of dissimilar statelets far away. In this way, we can extract discriminative feature candidates to distinguish the control performance with varying degrees. An information entropy-based selection strategy is proposed to choose information-rich statelet features that can best identify different control performances. After that, a statelets distance-based classifier is designed by computing the match distance between features of a testing sample and the extracted statelet features. The proposed SECL model focuses on the extracted statelets that can significantly reveal changes related to control performance rather than comparing the entire time series, which is more accurate and robust in detecting CPD with varying degrees. The effectiveness of the proposed method is illustrated through a real-world thermal power plant process. The experiment results show that SECL can capture the discriminative features and achieve prominent detection performance.
更多查看译文
关键词
Contrastive Learning,Control Performance Degradation,Statelet Distance,Power Generation
AI 理解论文
溯源树
样例
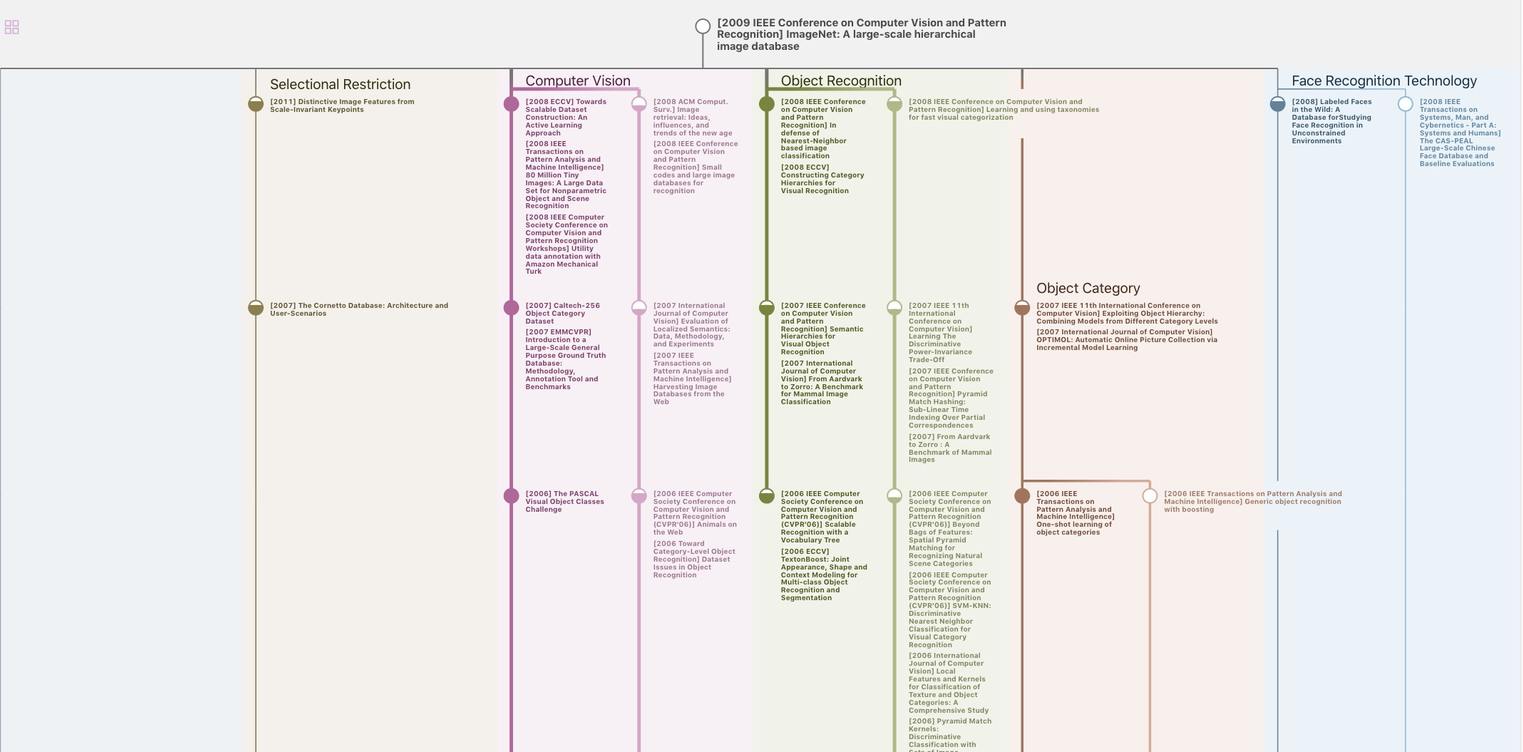
生成溯源树,研究论文发展脉络
Chat Paper
正在生成论文摘要