A New Approach to Medical Image Fusion Based on the Improved Extended Difference-of-gaussians Combined with the Coati Optimization Algorithm
BIOMEDICAL SIGNAL PROCESSING AND CONTROL(2024)
关键词
Coati optimization algorithm (COA),Extended difference-of-Gaussians (XDoG),Structure tensor (ST),Local energy (LE),Weighted mean curvature filter (WMCF)
AI 理解论文
溯源树
样例
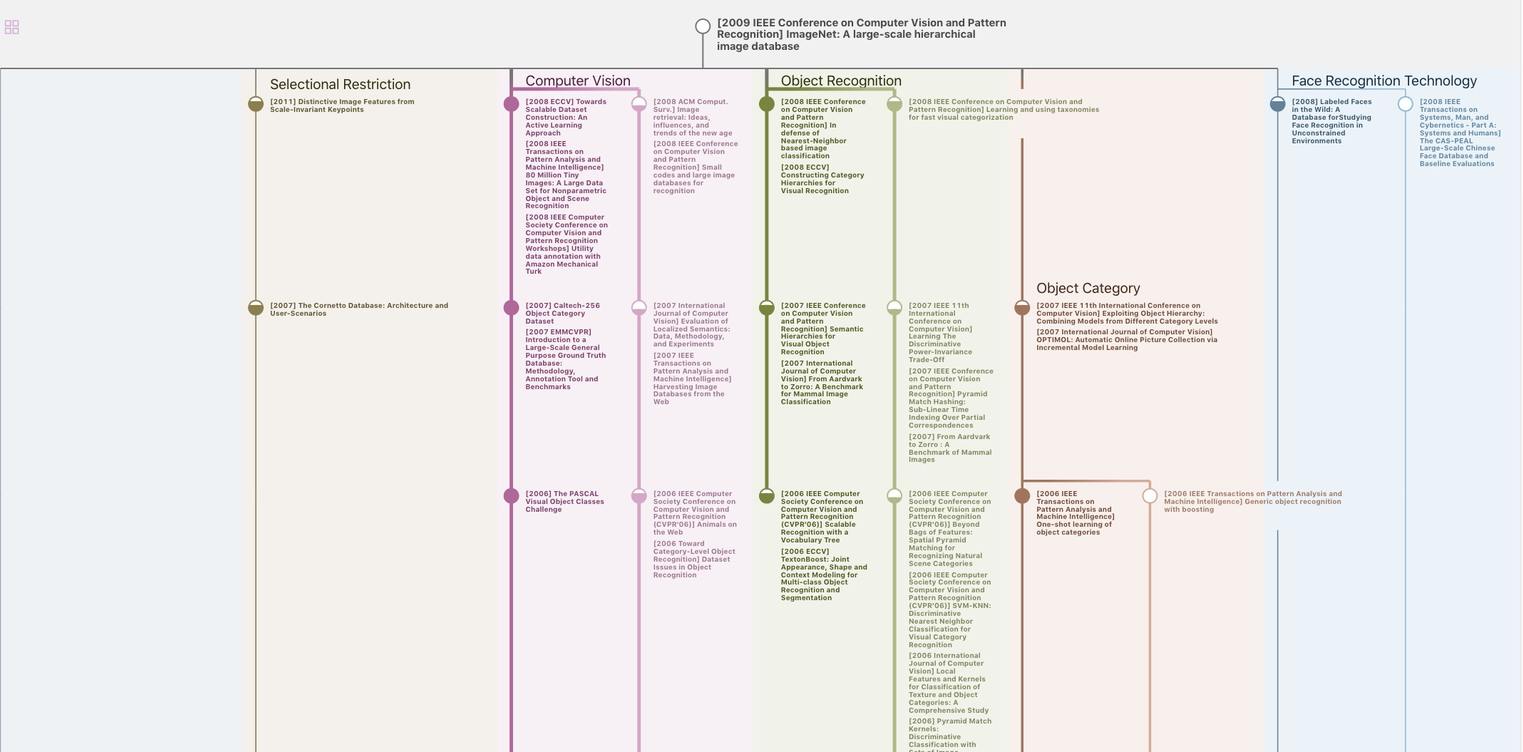
生成溯源树,研究论文发展脉络
Chat Paper
正在生成论文摘要