How Does Different Collected Data Influence Data-Driven Chiller Model – Analysis of Collected Data Distribution Using Causal Discovery Method
Building Simulation Conference Proceedings Proceedings of Building Simulation 2023 18th Conference of IBPSA(2023)
摘要
Chillers are widely used in commercial building. It is important to study impact of data distribution on the generalization performance of data-driven chiller models. We apply a causal discovery algorithm to analyze the multidimensional variable correlations in 138 simulated chiller operation datasets, consisting of one randomized controlled trial (RCT) and 137 observational experiments. Our workflow includes experiment data generation, variable correlation analysis, causal analysis, and causal graph similarity and chiller model generalizability analysis. The results demonstrate that variable correlations exist in the actual building's chiller operation data that differ from the experimental bench data. Furthermore, our study reveals that the chiller model trained with data from a richer control strategy exhibits better generalization performance. Our findings shed light on the importance of variable correlation in the dataset and provide insights into the effects of data distribution on chiller model performance.
更多查看译文
AI 理解论文
溯源树
样例
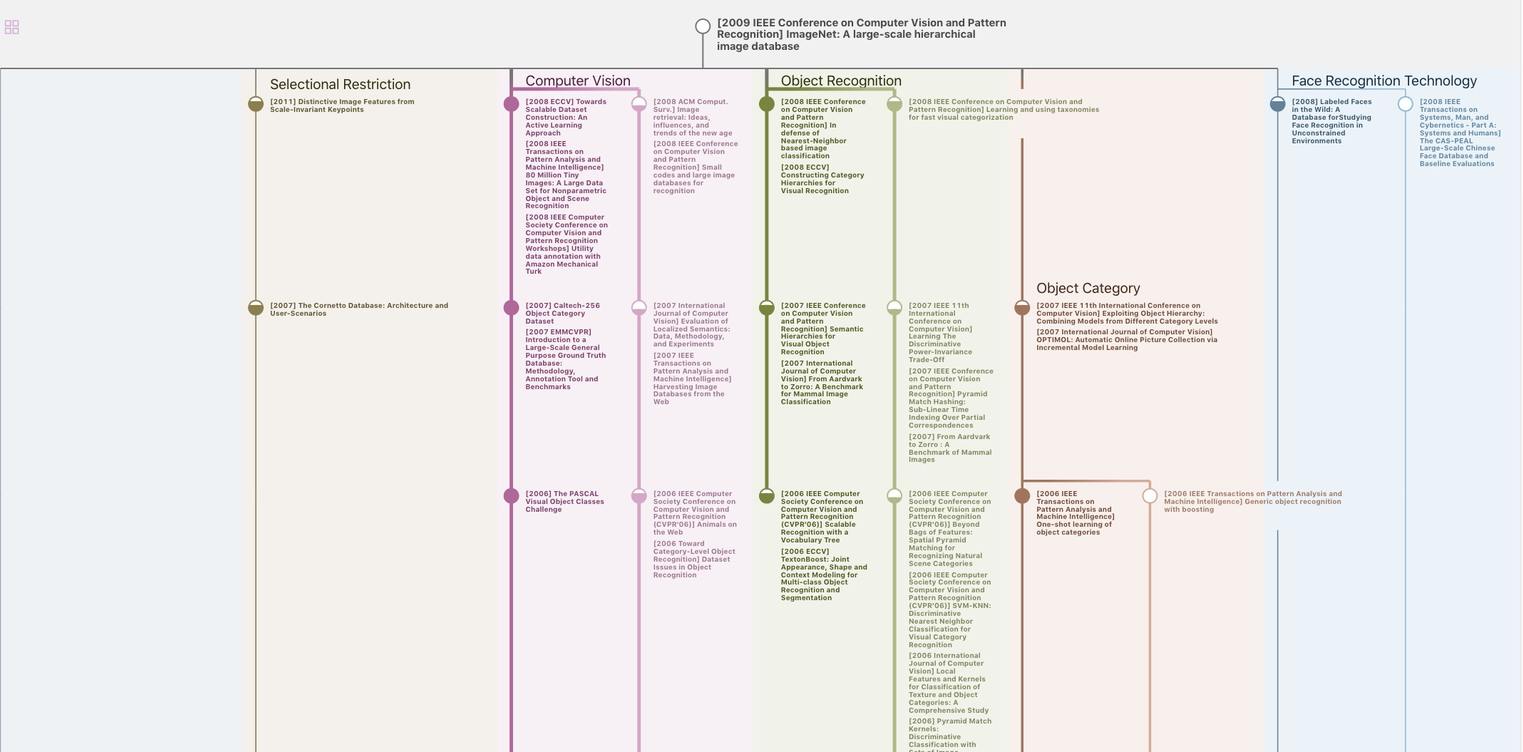
生成溯源树,研究论文发展脉络
Chat Paper
正在生成论文摘要