Gradient-free Neural Topology Optimization: Towards Effective Fracture-Resistant Designs
arxiv(2024)
摘要
Gradient-free optimizers allow for tackling problems regardless of the smoothness or differentiability of their objective function, but they require many more iterations to converge when compared to gradient-based algorithms. This has made them unviable for topology optimization due to the high computational cost per iteration and the high dimensionality of these problems. We propose a gradient-free neural topology optimization method using a pre-trained neural reparameterization strategy that addresses two key challenges in the literature. First, the method leads to at least one order of magnitude decrease in iteration count to reach minimum compliance when optimizing designs in latent space, as opposed to the conventional gradient-free approach without latent parameterization. This helps to bridge the large performance gap between gradient-free and gradient-based topology optimization for smooth and differentiable problems like compliance optimization, as demonstrated via extensive computational experiments in- and out-of-distribution with the training data. Second, we also show that the proposed method can optimize toughness of a structure undergoing brittle fracture more effectively than a traditional gradient-based optimizer, delivering an objective improvement in the order of 30 configurations. Although gradient-based topology optimization is more efficient for problems that are differentiable and well-behaved, such as compliance optimization, we believe that this work opens up a new path for problems where gradient-based algorithms have limitations.
更多查看译文
AI 理解论文
溯源树
样例
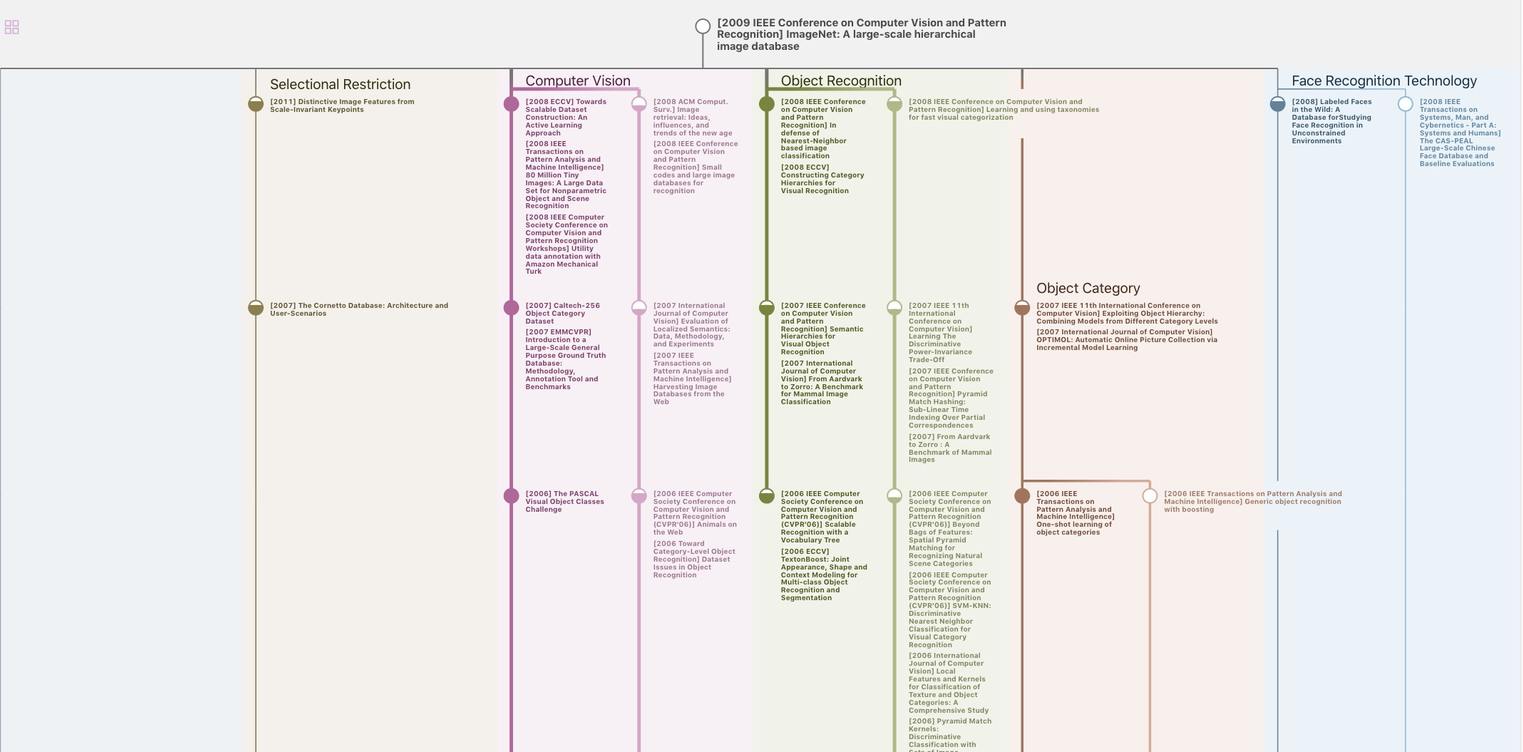
生成溯源树,研究论文发展脉络
Chat Paper
正在生成论文摘要