Medical Speech Symptoms Classification via Disentangled Representation
CoRR(2024)
摘要
Intent is defined for understanding spoken language in existing works. Both
textual features and acoustic features involved in medical speech contain
intent, which is important for symptomatic diagnosis. In this paper, we propose
a medical speech classification model named DRSC that automatically learns to
disentangle intent and content representations from textual-acoustic data for
classification. The intent representations of the text domain and the
Mel-spectrogram domain are extracted via intent encoders, and then the
reconstructed text feature and the Mel-spectrogram feature are obtained through
two exchanges. After combining the intent from two domains into a joint
representation, the integrated intent representation is fed into a decision
layer for classification. Experimental results show that our model obtains an
average accuracy rate of 95
更多查看译文
关键词
medical speech,multi-modal neural network,speech representation disentanglement
AI 理解论文
溯源树
样例
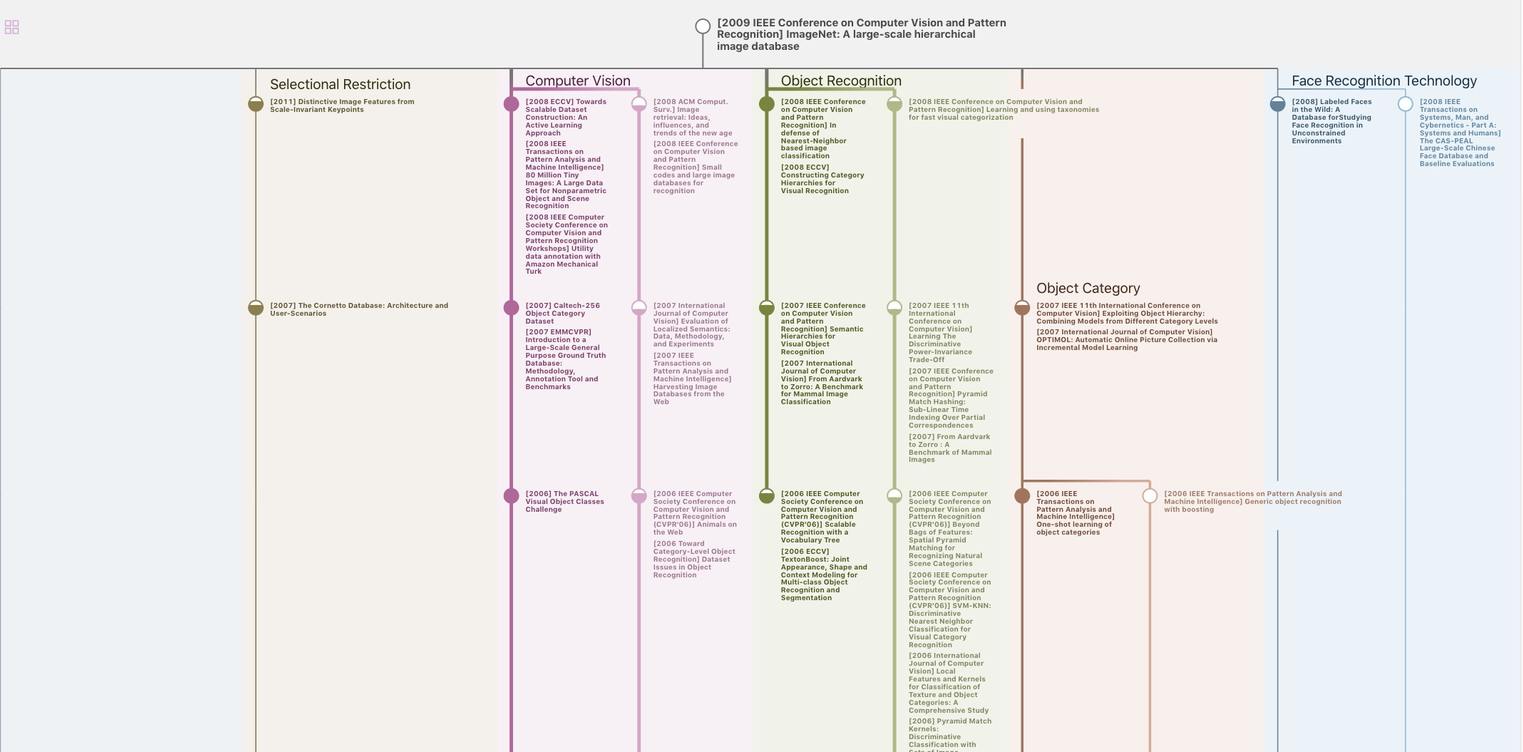
生成溯源树,研究论文发展脉络
Chat Paper
正在生成论文摘要