SIRST-5K: Exploring Massive Negatives Synthesis with Self-supervised Learning for Robust Infrared Small Target Detection
IEEE Transactions on Geoscience and Remote Sensing(2024)
摘要
Single-frame infrared small target (SIRST) detection aims to recognize small
targets from clutter backgrounds. Recently, convolutional neural networks have
achieved significant advantages in general object detection. With the
development of Transformer, the scale of SIRST models is constantly increasing.
Due to the limited training samples, performance has not been improved
accordingly. The quality, quantity, and diversity of the infrared dataset are
critical to the detection of small targets. To highlight this issue, we propose
a negative sample augmentation method in this paper. Specifically, a negative
augmentation approach is proposed to generate massive negatives for
self-supervised learning. Firstly, we perform a sequential noise modeling
technology to generate realistic infrared data. Secondly, we fuse the extracted
noise with the original data to facilitate diversity and fidelity in the
generated data. Lastly, we proposed a negative augmentation strategy to enrich
diversity as well as maintain semantic invariance. The proposed algorithm
produces a synthetic SIRST-5K dataset, which contains massive pseudo-data and
corresponding labels. With a rich diversity of infrared small target data, our
algorithm significantly improves the model performance and convergence speed.
Compared with other state-of-the-art (SOTA) methods, our method achieves
outstanding performance in terms of probability of detection (Pd), false-alarm
rate (Fa), and intersection over union (IoU).
更多查看译文
关键词
Infrared small target detection,self-supervised learning,noise displacement,negative sample augmentation
AI 理解论文
溯源树
样例
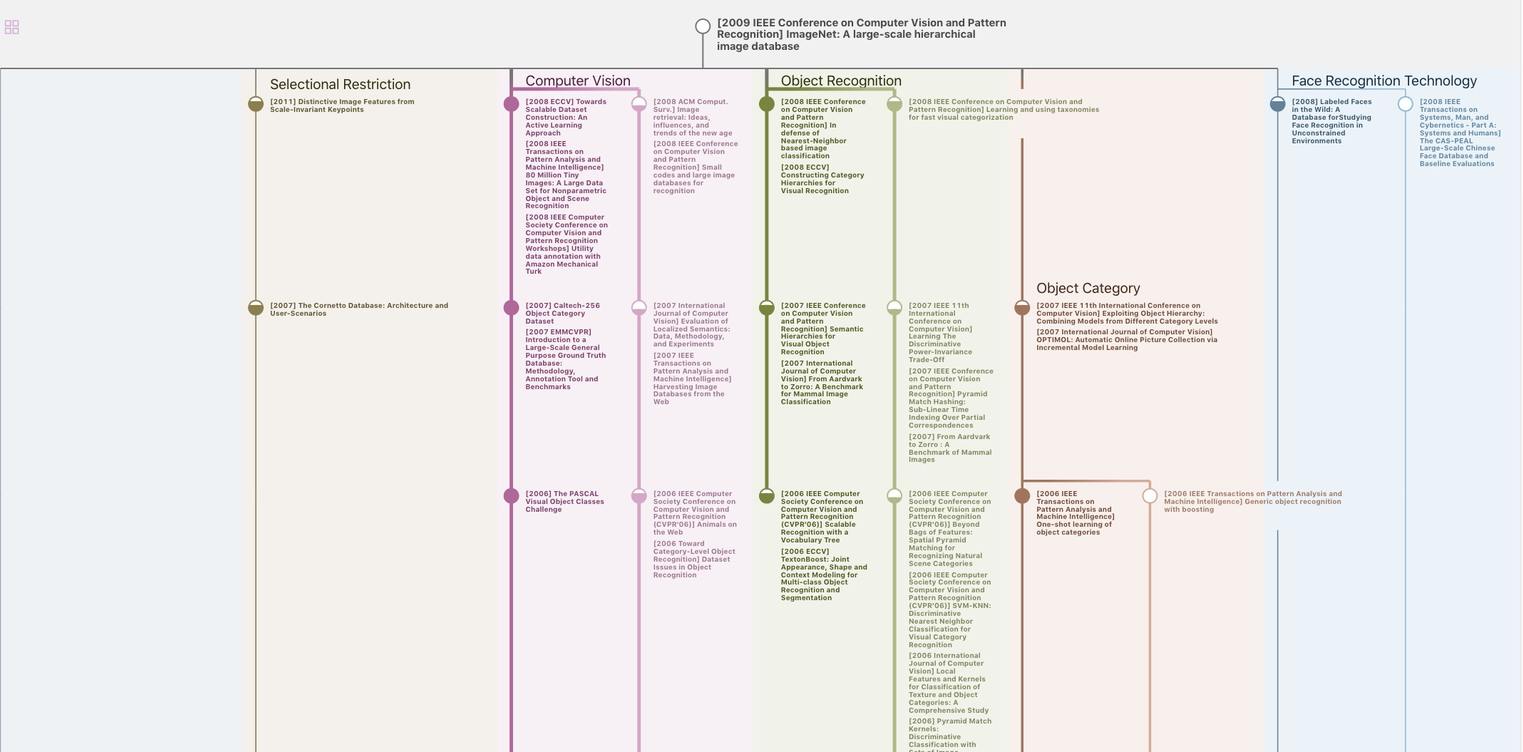
生成溯源树,研究论文发展脉络
Chat Paper
正在生成论文摘要