High-throughput measurement method for rice seedling based on improved UNet model
COMPUTERS AND ELECTRONICS IN AGRICULTURE(2024)
摘要
Seedling growth is essential for the subsequent development and yield formation. Monitoring the status of seedlings is essential for plant factories and population assessments. However, current methods lack the ability to rapidly, efficiently, and concurrently evaluate the characteristics of whole seedlings. In this study, we have developed a high-throughput measurement method (https://github.com/no6233470/Seedling_Measure) for evaluating rice seedling traits. We employed an improved UNet model to decrease time and costs, after comparing the performances of PSPNet, DeepLab V3+, and UNet using a dataset of 515 collected images of rice seedlings. To improve the ability of UNet model to effectively capture global contextual information, we incorporated Coordinate Attention (CA) and Vision Transformer (ViT) blocks. We have developed the CA(UP)UNet and CA(UP)-ViT-UNet models, which enhance model accuracy with minimal and moderate computational overhead, respectively. Compared to standard UNet, the CA(UP)-UNet model showed an increase of 1.38% in aboveground IoU (Intersection Over Union) and 1.45% in seed IoU. Similarly, the CA(UP)-ViT-UNet model demonstrated an increase of 1.63% in aboveground IoU and 2.21% in seed IoU. Based on the CA(UP)-UNet model, the high-throughput measurement method demonstrated excellent consistency and achieved a 13.7fold increase in efficiency compared to traditional measurement methods. We applied the method to evaluate the effects of brassinosteroid (BR) treatment on rice seedlings and found significant increases in plant height, aboveground length, and pixel number. Our research provides an efficient tool for analyzing plants using images and for nondestructively monitoring seedling growth. The high-throughput measurement method has the potential to revolutionize phenotype testing, population assessment, and crop variety selection in agriculture.
更多查看译文
关键词
Rice seedling traits,High-throughput phenotyping,Deep learning,Semantic segmentation,Attention mechanism
AI 理解论文
溯源树
样例
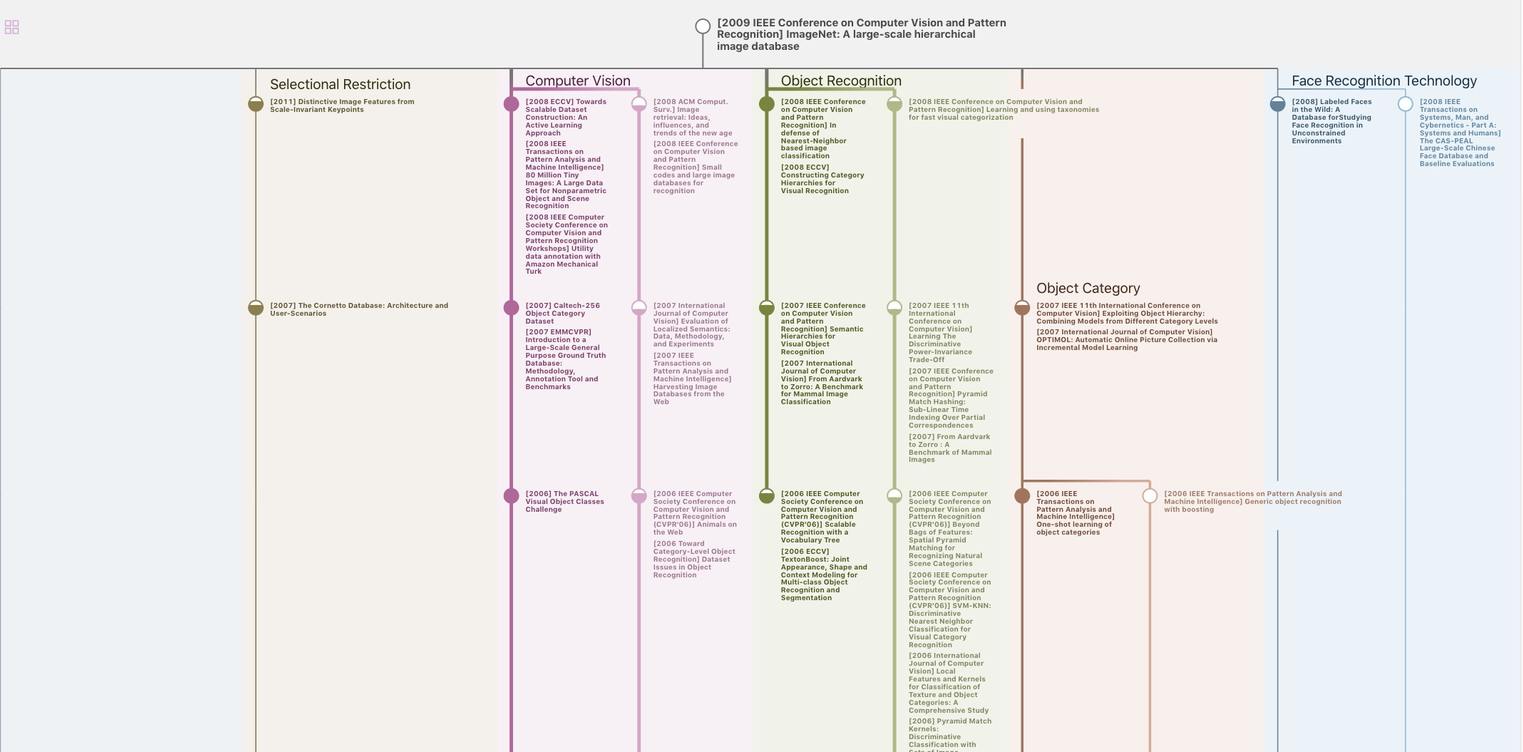
生成溯源树,研究论文发展脉络
Chat Paper
正在生成论文摘要