Applicability of an unsupervised cluster model developed on first wave COVID-19 patients in second/third wave critically ill patients
Medicina Intensiva (English Edition)(2024)
摘要
Objective
To validate the unsupervised cluster model (USCM) developed during the first pandemic wave in a cohort of critically ill patients from the second and third pandemic waves.
Design
Observational, retrospective, multicentre study.
Setting
Intensive Care Unit (ICU).
Patients
Adult patients admitted with COVID-19 and respiratory failure during the second and third pandemic waves.
Interventions
None.
Main variables of interest
Collected data included demographic and clinical characteristics, comorbidities, laboratory tests and ICU outcomes. To validate our original USCM, we assigned a phenotype to each patient of the validation cohort. The performance of the classification was determined by Silhouette coefficient (SC) and general linear modelling. In a post-hoc analysis we developed and validated a USCM specific to the validation set. The model’s performance was measured using accuracy test and area under curve (AUC) ROC.
Results
A total of 2330 patients (mean age 63 [53–82] years, 1643 (70.5%) male, median APACHE II score (12 [9–16]) and SOFA score (4 [3–6]) were included. The ICU mortality was 27.2%. The USCM classified patients into 3 clinical phenotypes: A (n = 1206 patients, 51.8%); B (n = 618 patients, 26.5%), and C (n = 506 patients, 21.7%). The characteristics of patients within each phenotype were significantly different from the original population. The SC was −0.007 and the inclusion of phenotype classification in a regression model did not improve the model performance (0.79 and 0.78 ROC for original and validation model). The post-hoc model performed better than the validation model (SC −0.08).
Conclusion
Models developed using machine learning techniques during the first pandemic wave cannot be applied with adequate performance to patients admitted in subsequent waves without prior validation.
更多查看译文
关键词
Severe SARS-CoV-2 infection,Phenotypes,Risk factors,Prognosis,Machine Learning,Validation,Infección grave por SARS-CoV-2,Fenotipos,Factores de riesgo,Pronóstico,Aprendizaje automático,Validación
AI 理解论文
溯源树
样例
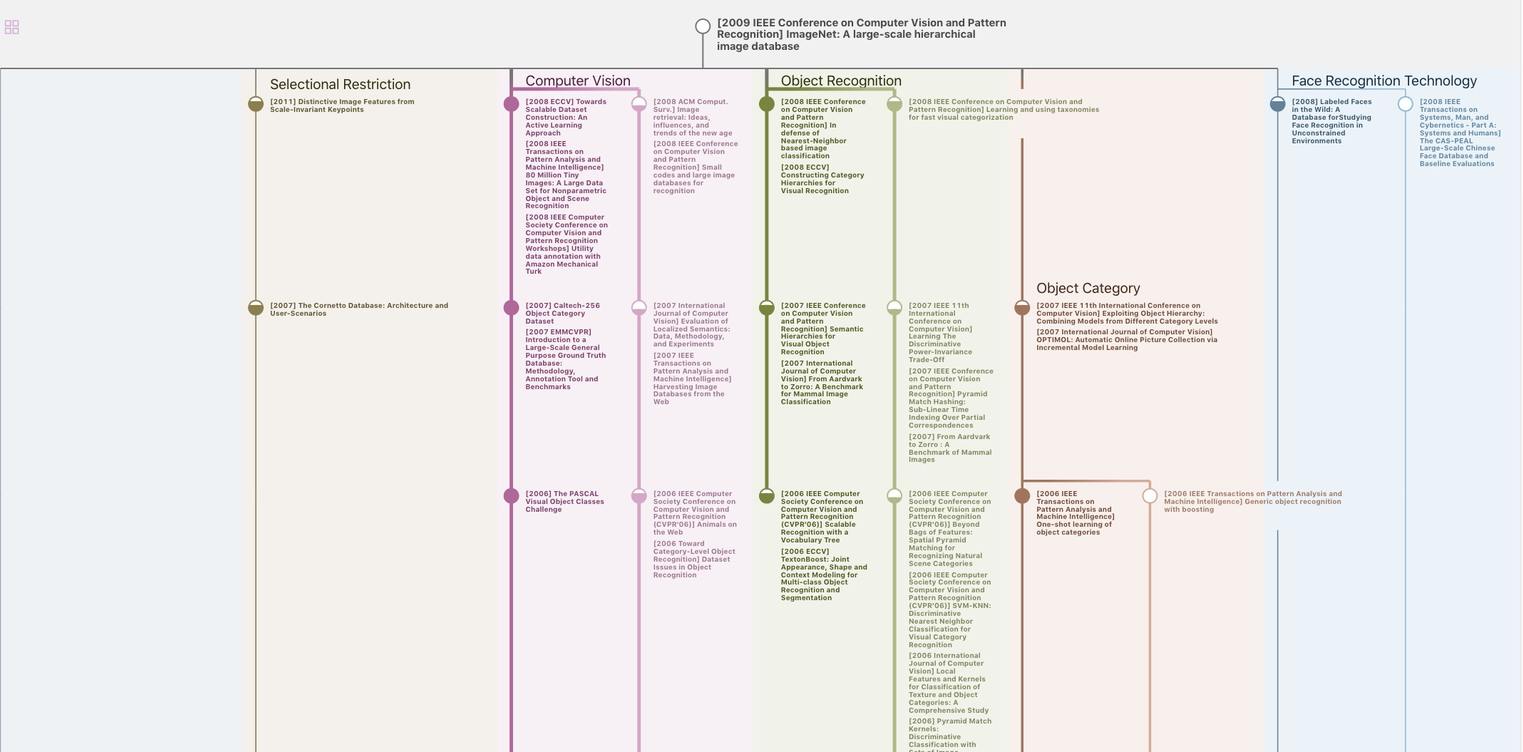
生成溯源树,研究论文发展脉络
Chat Paper
正在生成论文摘要