Early Stage Tumor Segmentation in Breast MRI Using Shape Enhanced U-Net
Biomedical Signal Processing and Control(2024)
摘要
Breast cancer is one of the most common cancers in women. Early diagnosis using MRI can significantly increase the cure rate. However, breast tumor in early stage is small whose edge is ambiguous, leading to false detection and missing detection. To this end, we propose a shape enhanced U-Net with Transformer encoder layer (called SETE-UNet) for automatically segmenting the tumor from breast cancer. First, Transformer encoder layer is introduced to provide global self-attention mechanisms, achieving obvious promotion for tumor segmentation. Second, a discriminative representation is calculated by the proposed shape enhanced branch, which introduces a novel branch to learn a shape boundary with additional supervision. This proposed module can help our algorithm focus on the relevant boundary and also achieve further performance improvement for tiny tumor. Furthermore, we construct an MRI dataset of breast tumors in early stage for breast tumor segmentation, containing 260 cases, including 243 women and 17 men. We compare the proposed method with the popular algorithms on our dataset of breast cancer in early stage, which shows that our method presents excellent segmentation results on breast MRI containing various organs with respect to MIoU, Precision, Accuracy, Dice, Sensitivity, Specificity, AUC, Hausdorff Distance.
更多查看译文
关键词
Breast cancer,U-Net,Segmentation,Transformer
AI 理解论文
溯源树
样例
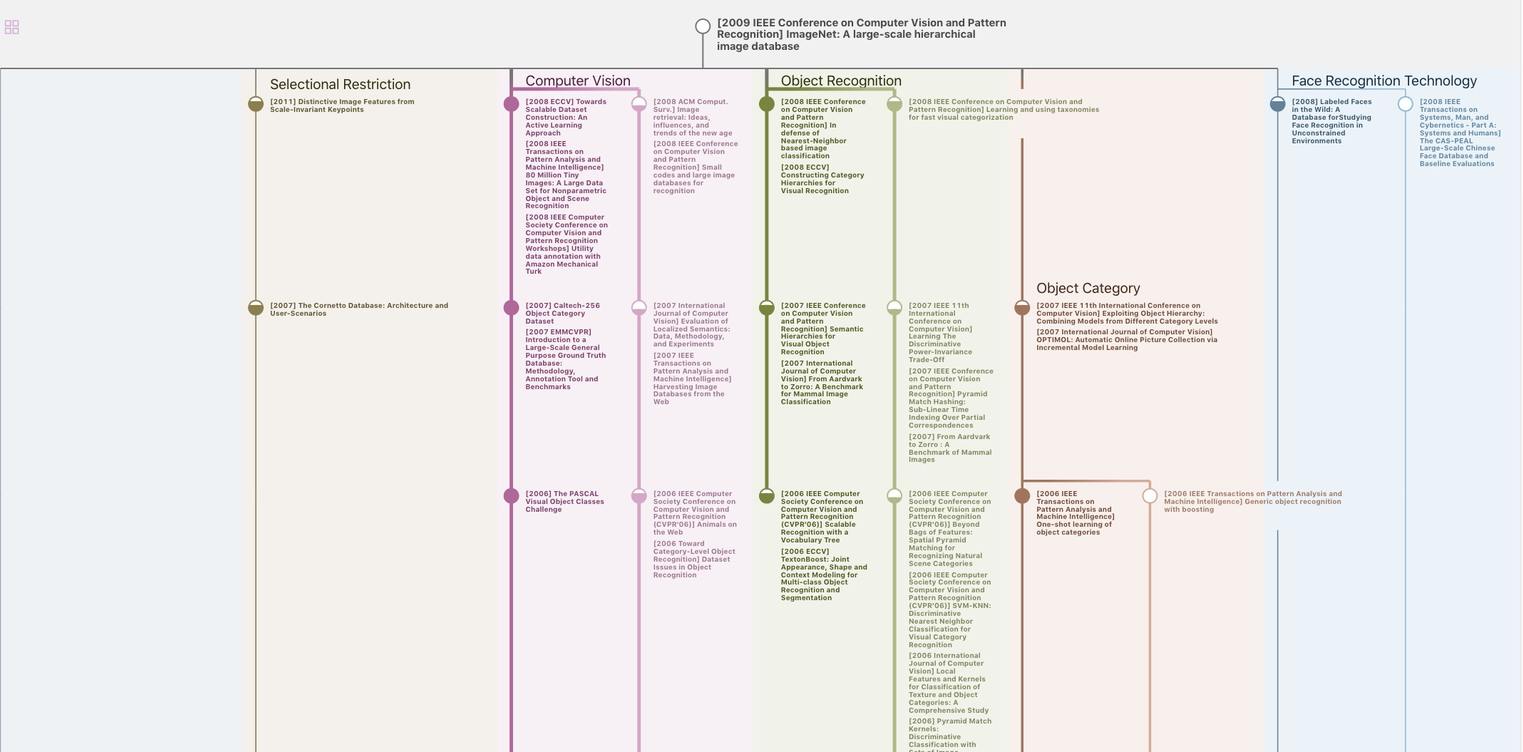
生成溯源树,研究论文发展脉络
Chat Paper
正在生成论文摘要