Developing Machine Learning Algorithms to Quantify Carbon Export Fluxes in the Ocean
crossref(2024)
摘要
Quantifying the ocean carbon export and sequestration is essential to understand not only the marine carbon cycle but also the impact of the biological pump (BP) on global carbon cycle. The BP includes the different mechanisms by which atmospheric carbon is transferred from the surface to the deep ocean in a process started by the carbon synthetization of phytoplankton and followed by the formation and sinking of the marine snow, enabling the storage of carbon for long periods of time. Particulate organic carbon (POC) downward flux is a key and necessary parameter to characterize the BP and the ocean carbon cycle models. In order to estimate POC flux, radioactive pairs (238U-234Th or 210Pb-210Po) disequilibrium and sediment traps are robust and accurate methods; however, they generally present low spatial-temporal resolution. In situ optical observations have shown a big potential to generate a wide database of POC fluxes. Artificial intelligence (AI) and machine learning (ML) algorithms have already shown their potential in the last years to improve the estimations of oceanic POC concentration. It can be obtained as a satellite-derived product using colour remote sensing data, as POC is correlated with optical properties and water components (suspended particulate matter and chlorophyll-a). In contrast, ML have just only recently started to be used to evaluate POC export fluxes, partly because the lack of consistent and extensive datasets combining POC fluxes and ancillary parameters. Here, we review the state of art of the use of ML techniques for POC concentration predictions as the cornerstone for estimations of POC export fluxes. The potential of ML methodologies to generate global reconstructions of particle fluxes in the ocean, using the current available POC flux databases, will be discussed and described. We will finally include general guidelines to analyse POC export evaluations using ML and comprehensive databases.
更多查看译文
AI 理解论文
溯源树
样例
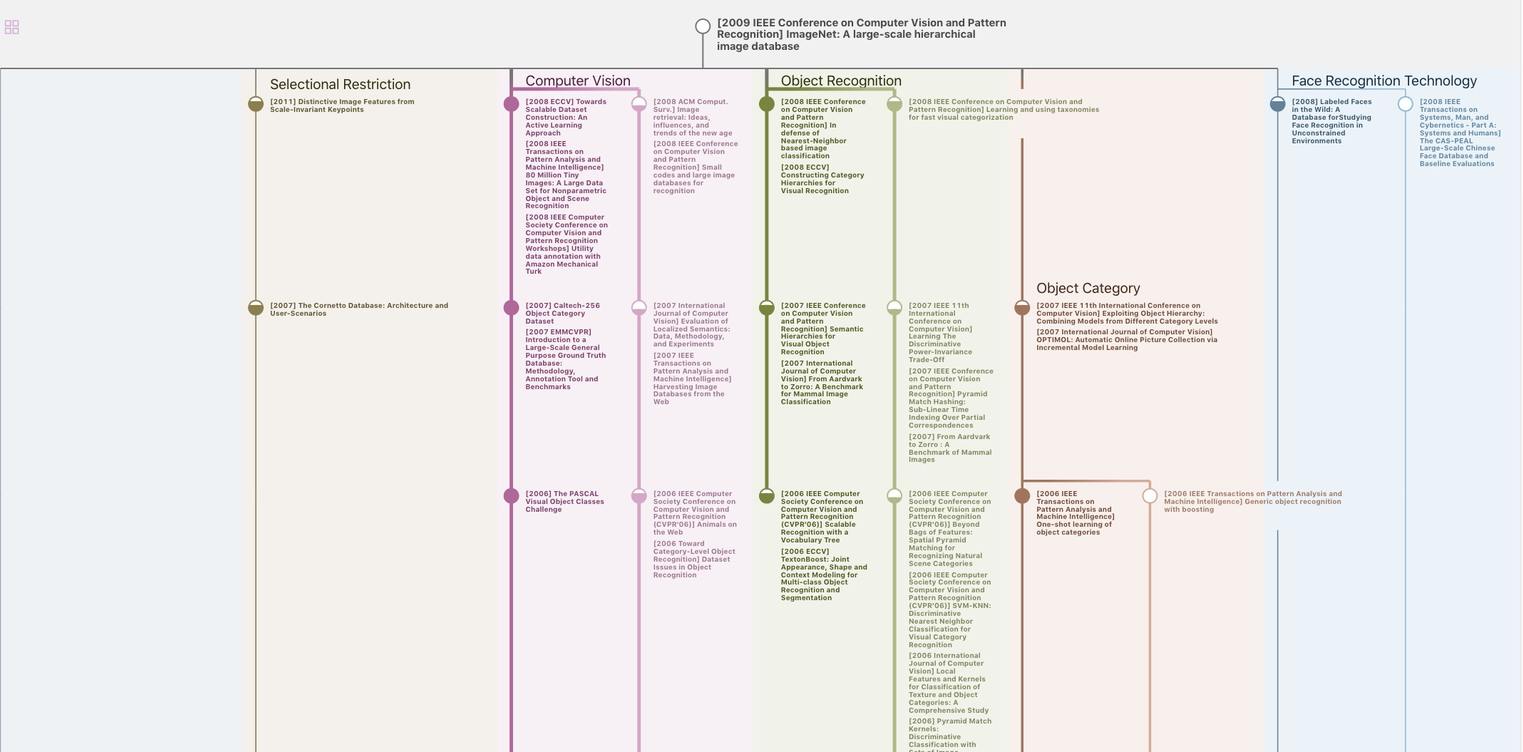
生成溯源树,研究论文发展脉络
Chat Paper
正在生成论文摘要