Global Ionospheric Storm Prediction Based on Deep Learning Methods
crossref(2024)
摘要
In recent years, deep learning algorithms have been widely used for ionospheric prediction, but there are still shortcomings in predicting ionospheric storms, such as insufficient accuracy, poor prediction performance, and high false alarm rates. In order to solve this problem, this article takes the F10.7, Kp, ap, time factor, and historical TEC data as feature input models, and establishes a Mixed CNN-BiLSTM network to predict global TEC. The model is trained using the longest available (23 years) Global Ionospheric Map (GIM)-Total Electron Content (TEC) and evaluated from multiple perspectives to assess the accuracy of ionospheric storm predictions. The results indicate that using historical Total Electron Content (TEC) in the heliocentric coordinate system as input driving data achieves higher prediction accuracy compared to using historical TEC in the geocentric coordinate system. Additionally, by comparing different input parameters, it is found that incorporating the Kp, ap, and Dst as inputs to the model effectively improves its accuracy. Among the different models compared, the Mixed CNN-BiLSTM model achieves the highest prediction accuracy. Furthermore, the inclusion of the CNN module enhances the overall prediction accuracy, while incorporating the DNN module with geomagnetic indices improves the accuracy of long-term predictions. Moreover, a comprehensive evaluation of the prediction results is conducted. In short-term predictions, the model accurately forecasts the occurrence, magnitude, and evolution process of ionospheric storms, as well as the variations in quiet-time TEC. When extending the prediction duration, although there are cases of false alarms, the model still captures the entire process of ionospheric storms in most events. It is also observed that the model performs better in daytime compared to nighttime, and the accuracy is higher in low-latitude regions compared to high-latitude regions. Additionally, the model exhibits higher accuracy in predicting positive storms compared to negative storms.
更多查看译文
AI 理解论文
溯源树
样例
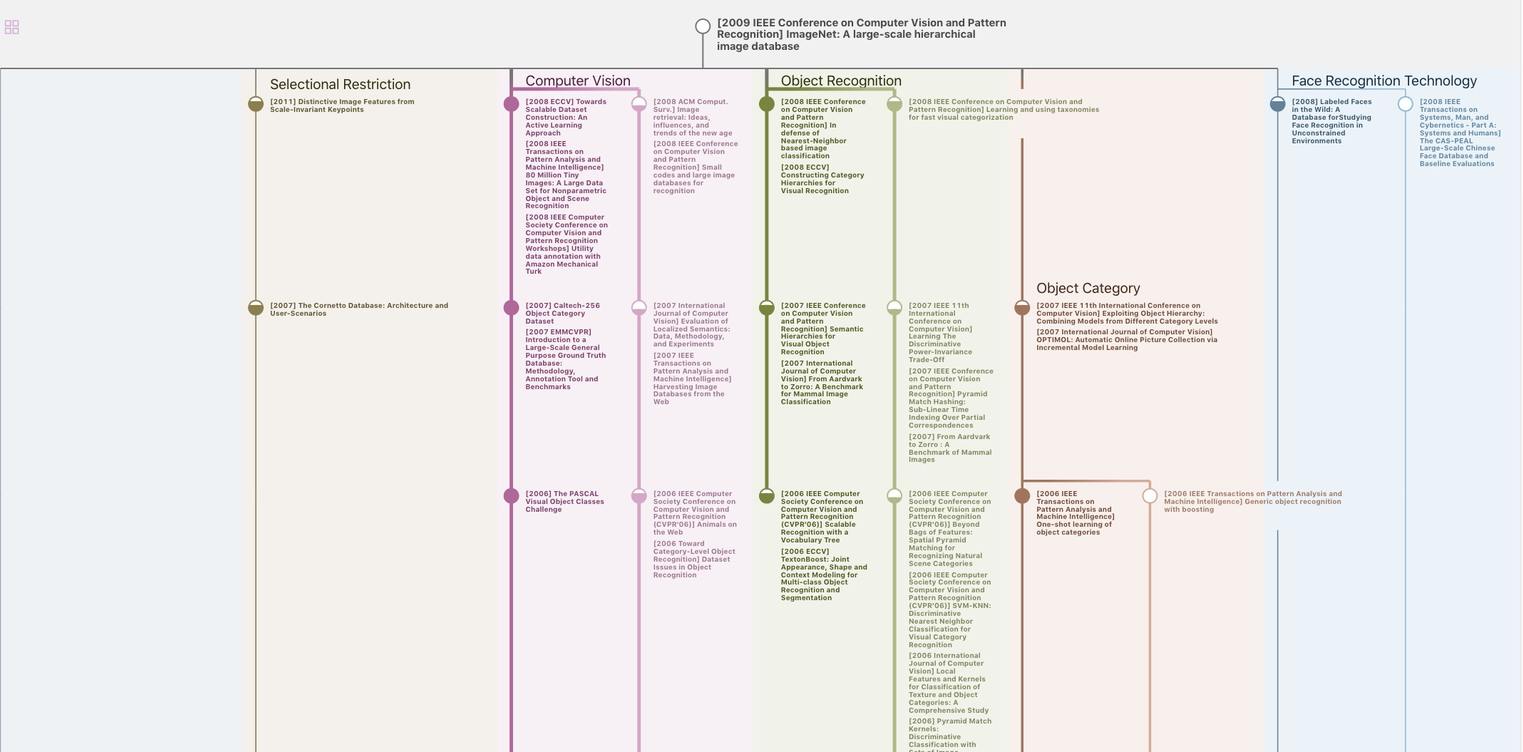
生成溯源树,研究论文发展脉络
Chat Paper
正在生成论文摘要