Water Depth Prediction in Combined Sewer Networks, Application of Generative Adversarial Networks
Deleted Journal(2024)
Abstract
This paper addresses the pressing issue of combined sewer overflows (CSOs) in urban areas, which pose significant environmental and public health threats. CSOs occur when combined sewer systems become overwhelmed during heavy rainfall, leading to untreated sewage and stormwater being discharged into nearby water bodies. To effectively manage and mitigate CSO effects, accurate predictions of CSOs are crucial for real-time control measures. This study introduces an innovative approach that utilizes Generative Adversarial Networks (GANs) to augment data and improve the accuracy of data-driven models for predicting water depth in combined sewer systems. Apart from data augmentation, the paper addresses scenarios where rare patterns, such as extreme events, are infrequently observed regardless of dataset size. It proposes a specialized generative model based on GANs designed to augment datasets targeting these rare patterns. This innovation involves adding a tail-focused loss function to the adversarial objective of the GAN training pipeline. The research is exemplified through a case study in Kaiserslautern, Germany. The results indicate an improvement in water depth predictions. Also, it was found that the model trained solely with synthetic data is comparable to that trained with real data. Graphical Abstract
MoreTranslated text
Key words
Machine learning,Generative adversarial networks,Urban drainage,Combined sewer overflow,Time-series prediction
AI Read Science
Must-Reading Tree
Example
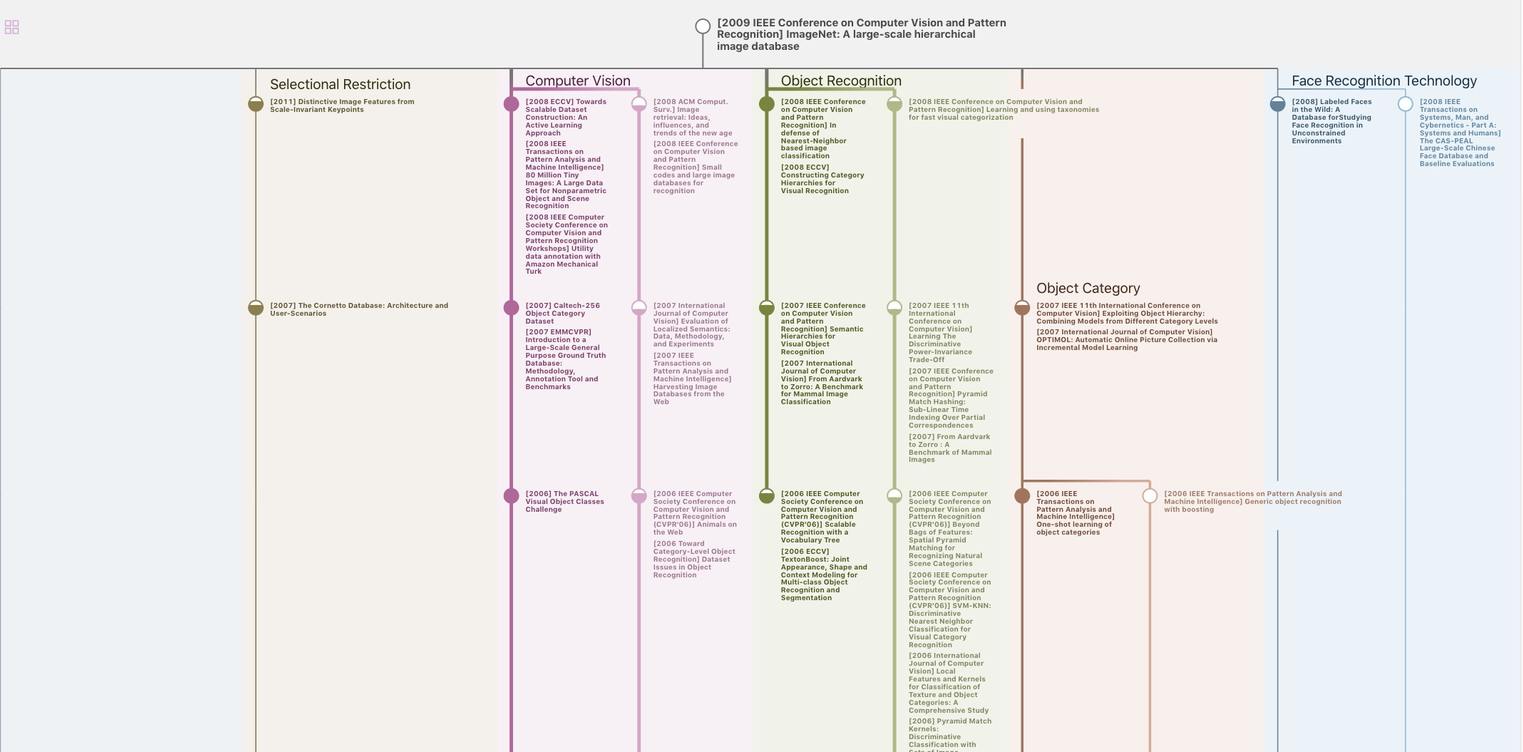
Generate MRT to find the research sequence of this paper
Chat Paper
Summary is being generated by the instructions you defined