Geometric Neural Network based on Phase Space for BCI decoding
arxiv(2024)
摘要
The integration of Deep Learning (DL) algorithms on brain signal analysis is
still in its nascent stages compared to their success in fields like Computer
Vision, especially in Brain-Computer Interface (BCI), where the brain activity
is decoded to control external devices without requiring muscle control.
Electroencephalography (EEG) is a widely adopted choice for designing BCI
systems due to its non-invasive and cost-effective nature and excellent
temporal resolution. Still, it comes at the expense of limited training data,
poor signal-to-noise, and a large variability across and within-subject
recordings. Finally, setting up a BCI system with many electrodes takes a long
time, hindering the widespread adoption of reliable DL architectures in BCIs
outside research laboratories. To improve adoption, we need to improve user
comfort using, for instance, reliable algorithms that operate with few
electrodes. Approach: Our research aims to develop a DL algorithm that
delivers effective results with a limited number of electrodes. Taking
advantage of the Augmented Covariance Method with SPDNet, we propose the
SPDNet_ψ architecture and analyze its performance and computational
impact, as well as the interpretability of the results. The evaluation is
conducted on 5-fold cross-validation, using only three electrodes positioned
above the Motor Cortex. The methodology was tested on nearly 100 subjects from
several open-source datasets using the Mother Of All BCI Benchmark (MOABB)
framework. Main results: The results of our SPDNet_ψ
demonstrate that the augmented approach combined with the SPDNet significantly
outperforms all the current state-of-the-art DL architecture in MI decoding.
Significance: This new architecture is explainable, with a low number
of trainable parameters and a reduced carbon footprint.
更多查看译文
AI 理解论文
溯源树
样例
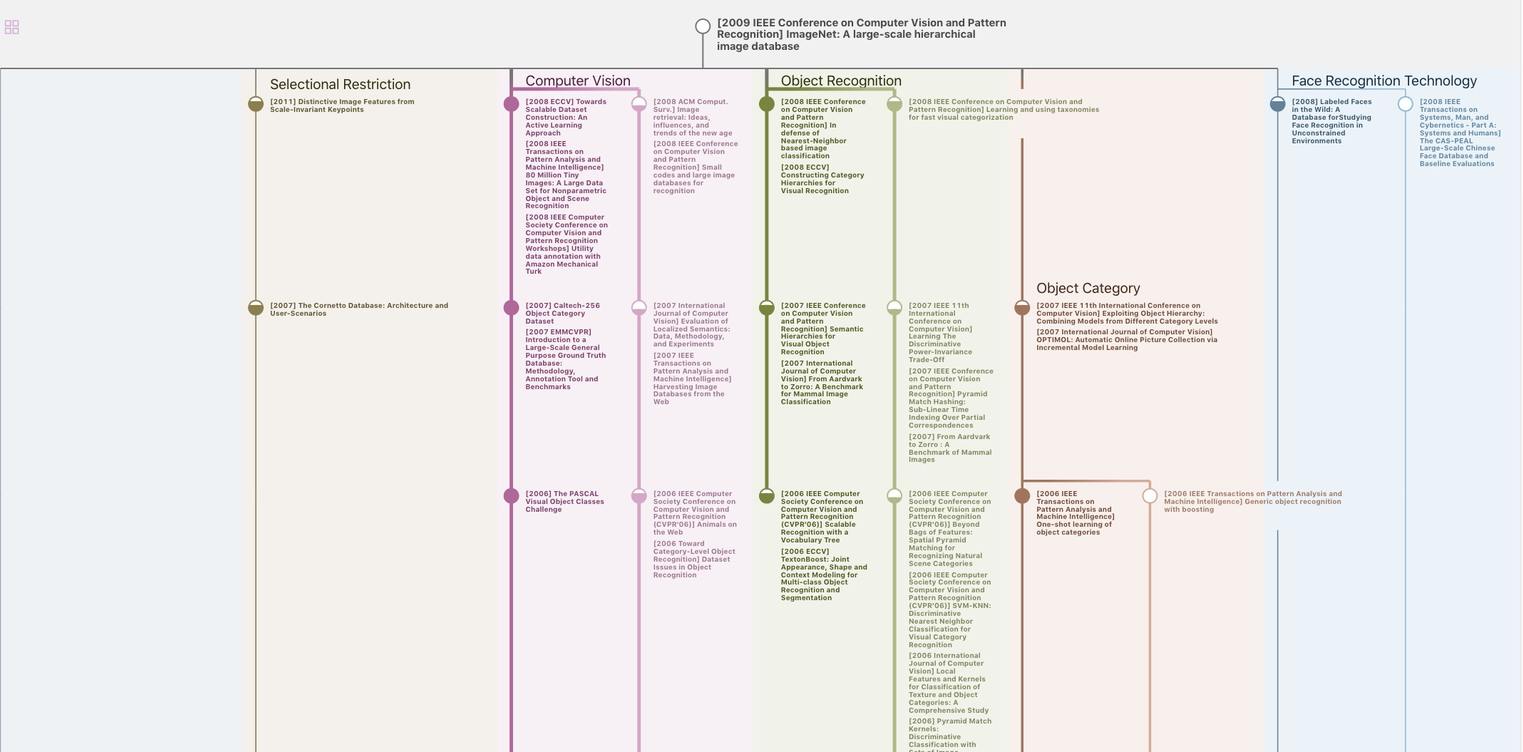
生成溯源树,研究论文发展脉络
Chat Paper
正在生成论文摘要