On the equivalence of direct and indirect data-driven predictive control approaches
arxiv(2024)
摘要
Recently, several direct Data-Driven Predictive Control (DDPC) methods have
been proposed, advocating the possibility of designing predictive controllers
from historical input-output trajectories without the need to identify a model.
In this work, we show that these approaches are equivalent to an indirect
approach. Reformulating the direct methods in terms of estimated parameters and
covariance matrices allows us to give new insights into how they work in
comparison with, for example, Subspace Predictive Control (SPC). In particular,
we show that for unconstrained problems the direct methods are equivalent to
SPC with a reduced weight on the tracking cost. Via a numerical experiment,
motivated by the reformulation, we also illustrate why the performance of
direct DDPC methods with fixed regularization tends to degrade as the number of
training samples increases.
更多查看译文
AI 理解论文
溯源树
样例
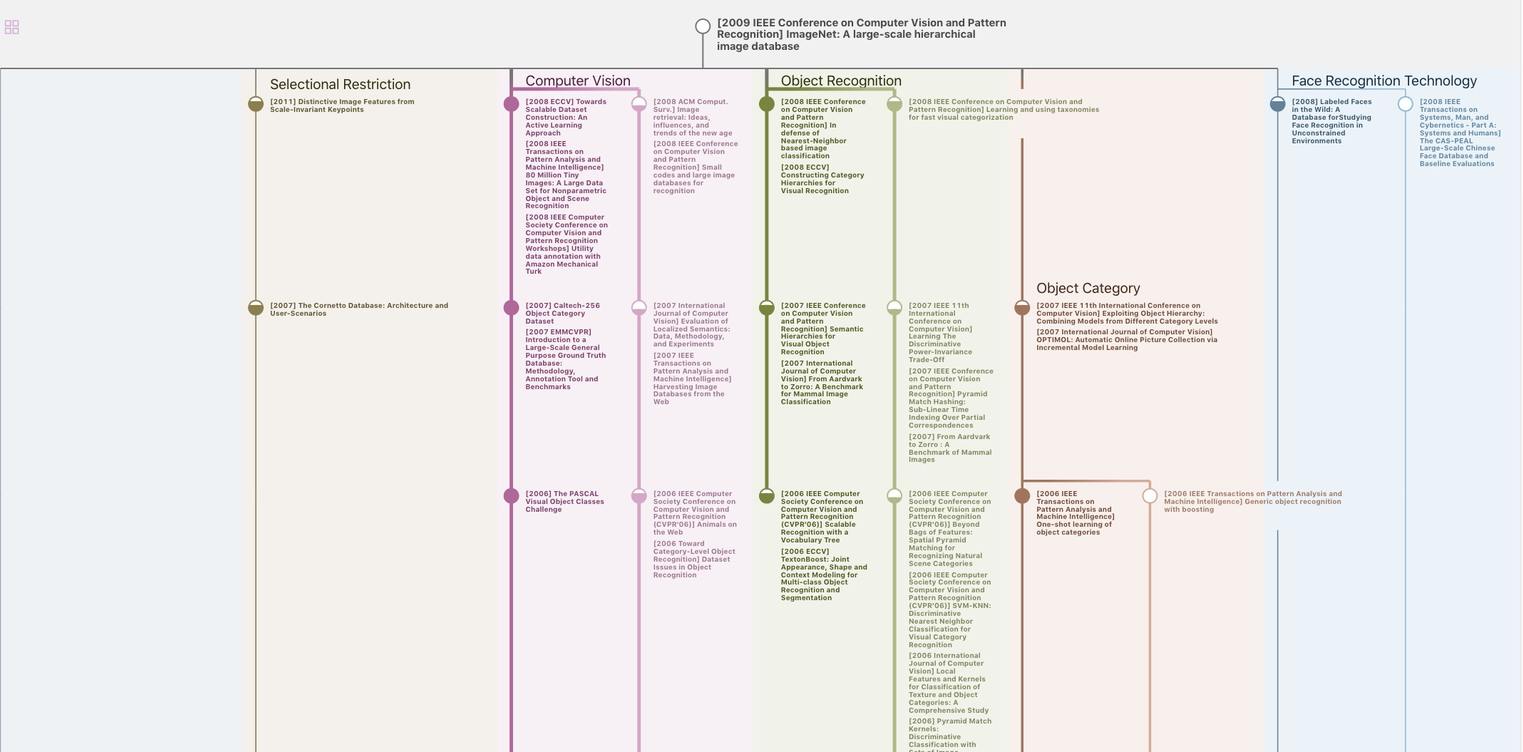
生成溯源树,研究论文发展脉络
Chat Paper
正在生成论文摘要