Almost Optimal Agnostic Control of Unknown Linear Dynamics
arxiv(2024)
摘要
We consider a simple control problem in which the underlying dynamics depend
on a parameter a that is unknown and must be learned. We study three variants
of the control problem: Bayesian control, in which we have a prior belief about
a; bounded agnostic control, in which we have no prior belief about a but
we assume that a belongs to a bounded set; and fully agnostic control, in
which a is allowed to be an arbitrary real number about which we have no
prior belief. In the Bayesian variant, a control strategy is optimal if it
minimizes a certain expected cost. In the agnostic variants, a control strategy
is optimal if it minimizes a quantity called the worst-case regret. For the
Bayesian and bounded agnostic variants above, we produce optimal control
strategies. For the fully agnostic variant, we produce almost optimal control
strategies, i.e., for any ε>0 we produce a strategy that minimizes
the worst-case regret to within a multiplicative factor of (1+ε).
更多查看译文
AI 理解论文
溯源树
样例
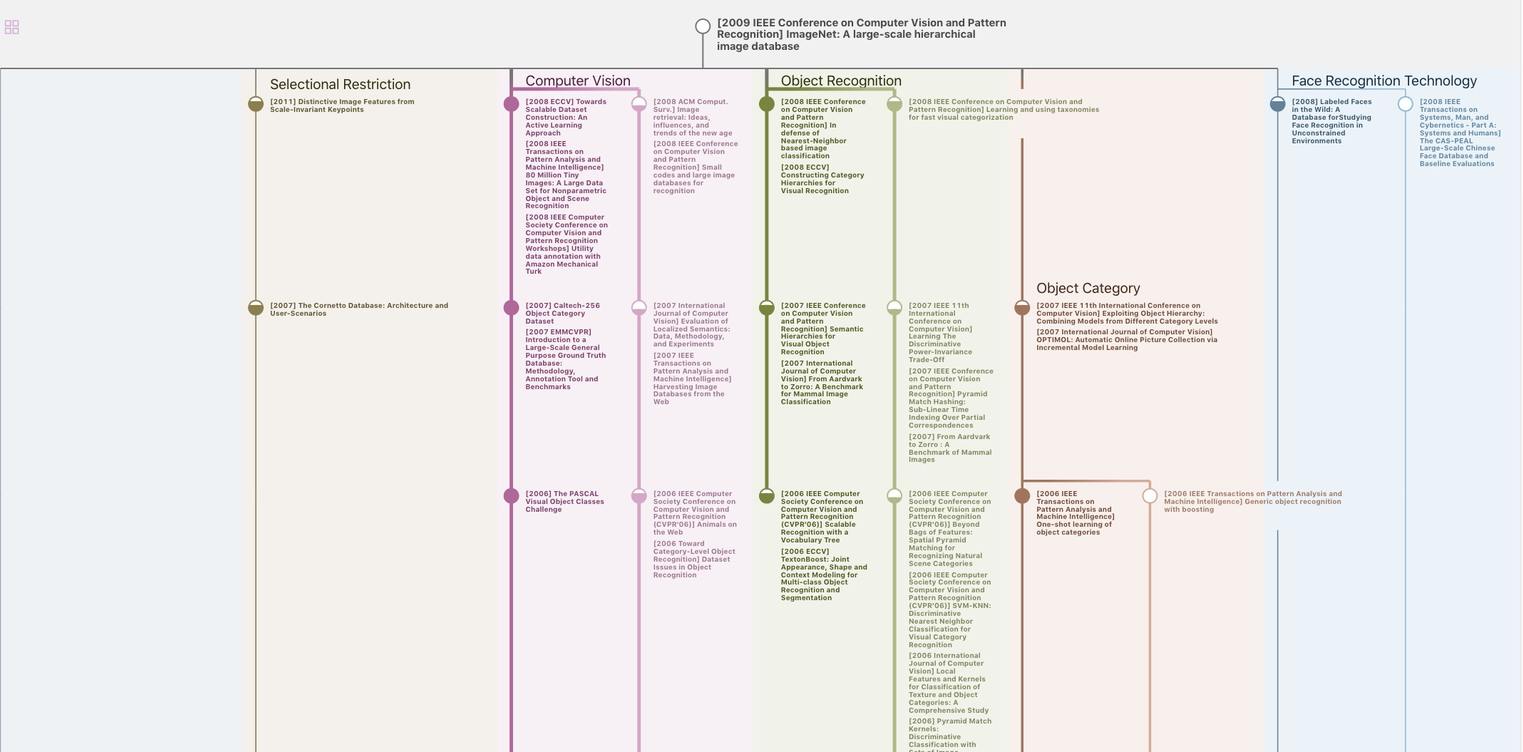
生成溯源树,研究论文发展脉络
Chat Paper
正在生成论文摘要