ReStainGAN: Leveraging IHC to IF Stain Domain Translation for in-silico Data Generation
CoRR(2024)
摘要
The creation of in-silico datasets can expand the utility of existing
annotations to new domains with different staining patterns in computational
pathology. As such, it has the potential to significantly lower the cost
associated with building large and pixel precise datasets needed to train
supervised deep learning models. We propose a novel approach for the generation
of in-silico immunohistochemistry (IHC) images by disentangling morphology
specific IHC stains into separate image channels in immunofluorescence (IF)
images. The proposed approach qualitatively and quantitatively outperforms
baseline methods as proven by training nucleus segmentation models on the
created in-silico datasets.
更多查看译文
AI 理解论文
溯源树
样例
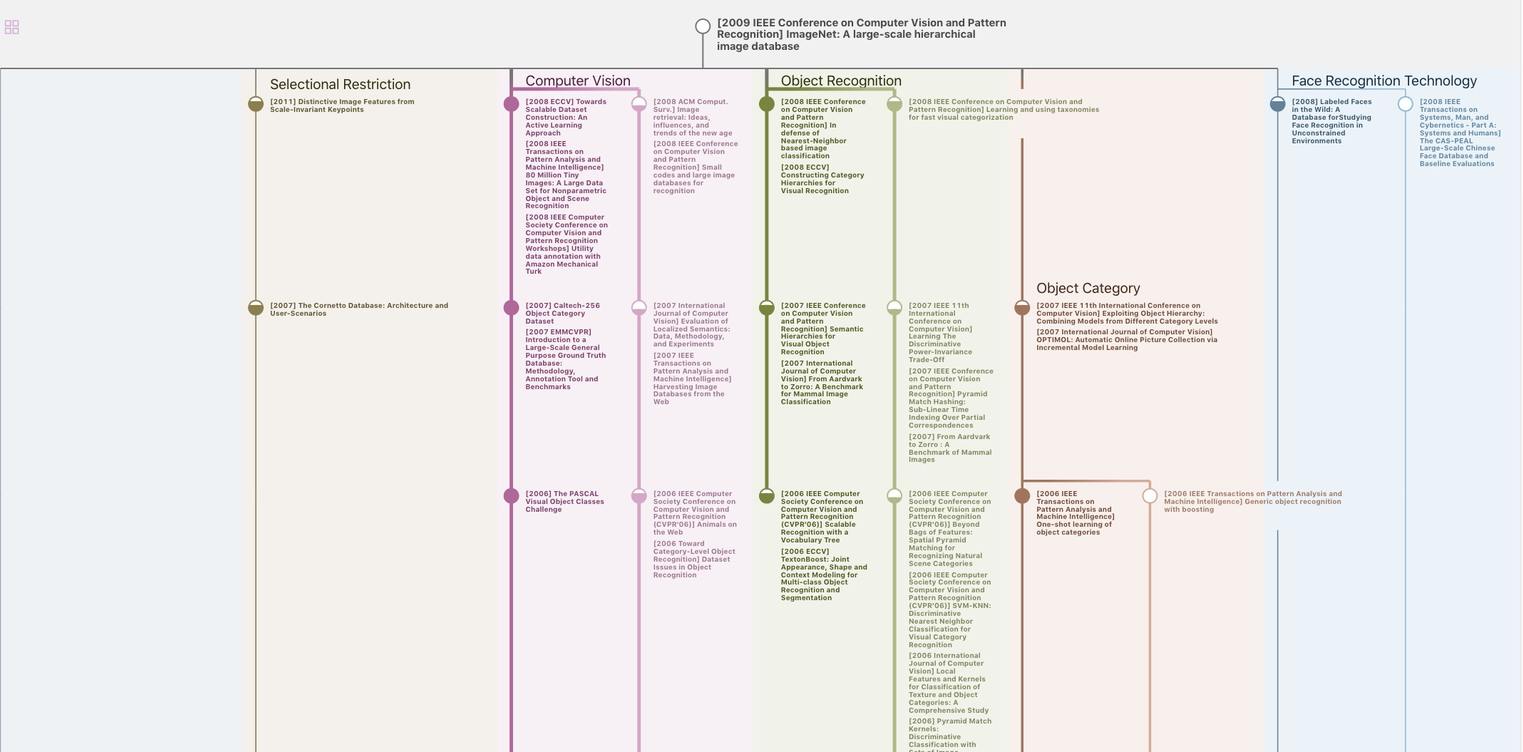
生成溯源树,研究论文发展脉络
Chat Paper
正在生成论文摘要