Assessing Coastal Resilience from Space
crossref(2024)
摘要
Satellite imagery proves to be a promising data source to gain insights in historic shoreline behavior over the last 4 decades on a global scale. To enable the use of such a large amount of satellite data, image processing techniques are introduced to interpret such large datasets. Furthermore, Machine Learning (ML) algorithms allow for an extra in-depth understanding of the shoreline dynamics, while growing computational power and standardization of ML packages, opens possibilities for studying shoreline dynamics and their drivers on a global scale. In this way, human drivers, such as nourishments, ports, coastal structures, and natural drivers, such as relative sea level rise, inlet systems, and storms, can be identified across the globe. The high spatial and temporal resolution of this information yields more comprehensive understanding of our coasts and their resilience to cope with a changing climate. This is not only of great added value in data-poor environments, but it will also allow for more cost-effective coastal monitoring in data rich environments as the necessity of in-situ measurements will reduce in future. Furthermore, information on the governing drivers for local coastal change is one of the key elements required for shoreline predictions. Providing such a prediction for future shoreline positions is just one example of a climate service. To prepare coastal zones for a changing climate in the future, coastal managers are demanding various other climate services to efficiently access and use state-of-the-art data on projections related to flooding, erosion, subsidence, vulnerability of assets and adaptation measures. The CoCliCo platform will be presented that fulfils the stakeholder needs by providing climate services at a pan-European scale.
更多查看译文
AI 理解论文
溯源树
样例
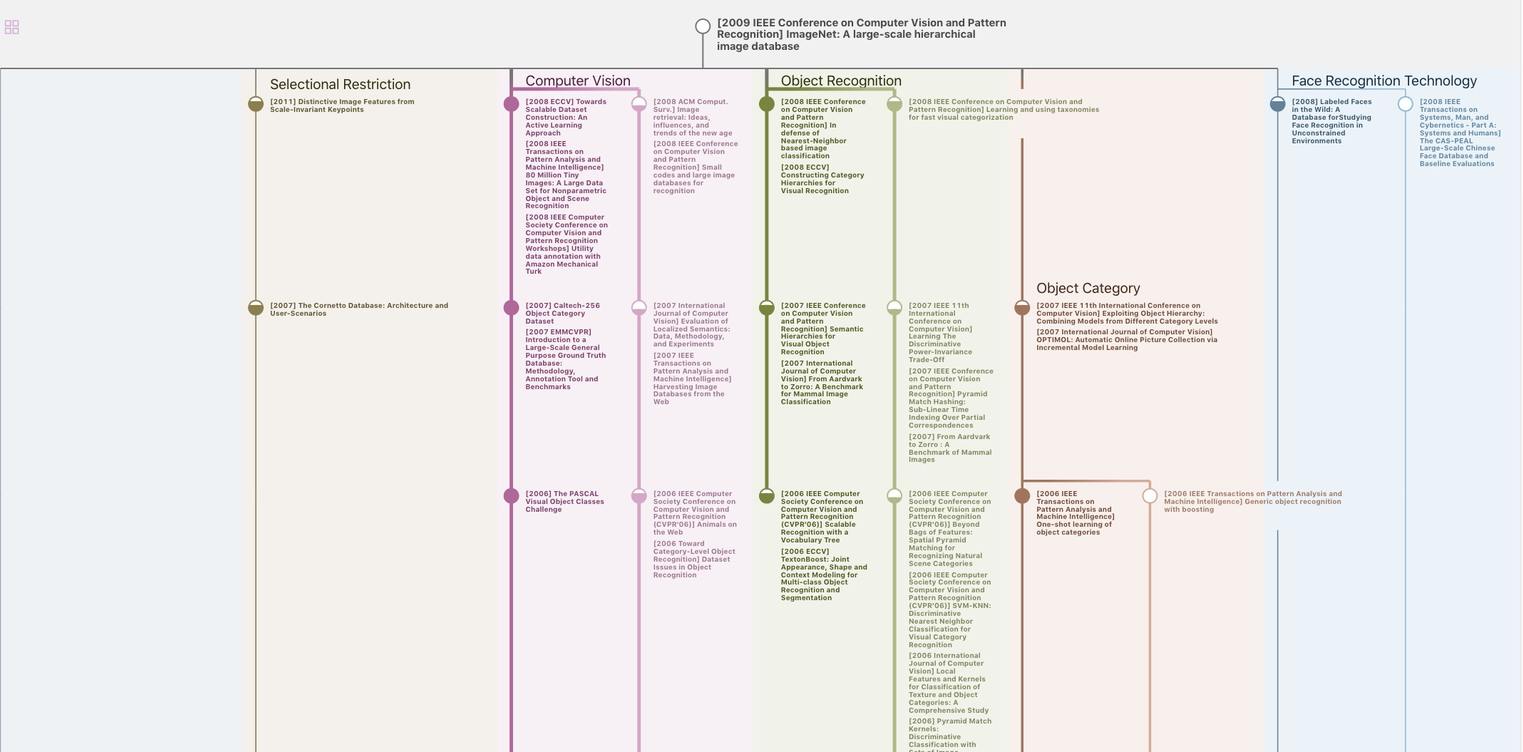
生成溯源树,研究论文发展脉络
Chat Paper
正在生成论文摘要